DataFrame(데이터프레임) 심화
1. 데이터 결합 및 분해
1.1 데이터 결합
1.1.1 equals
equals
메서드는 데이터프레임이 다른 데이터프레임과 같은지 비교할 수 있습니다. 출력 결과를 보시면 df1과 df2는 다른 데이터프레임인 것을 확인하실 수 있습니다.
df1 = pl.DataFrame(
{
"foo": [1, 2, 3],
"bar": [6.0, 7.0, 8.0],
"ham": ["a", "b", "c"],
}
)
df2 = pl.DataFrame(
{
"foo": [3, 2, 1],
"bar": [8.0, 7.0, 6.0],
"ham": ["c", "b", "a"],
}
)
# 데이터프레임1.equals(데이터프레임2)
print(df1.equals(df1))
print(df1.equals(df2))
df1 = pl.DataFrame(
{
"foo": [1, 2, 3],
"bar": [6.0, 7.0, 8.0],
"ham": ["a", "b", "c"],
}
)
df2 = pl.DataFrame(
{
"foo": [3, 2, 1],
"bar": [8.0, 7.0, 6.0],
"ham": ["c", "b", "a"],
}
)
# 데이터프레임1.equals(데이터프레임2)
print(df1.equals(df1))
print(df1.equals(df2))
True
False
True
False
df1과 구조, 값 모두 같은 데이터프레임을 생성해보고 비교해보도록 하겠습니다. 출력 결과를 보시면 df와 df1은 같은 데이터프레임을 알 수 있습니다. 이처럼 데이터프레임의 컬럼명과, 구조, 데이터 값들이 모두 같으면 True라고 출력됩니다.
df = pl.DataFrame(
{
"foo": [1, 2, 3],
"bar": [6.0, 7.0, 8.0],
"ham": ["a", "b", "c"],
}
)
print(df.equals(df1))
df = pl.DataFrame(
{
"foo": [1, 2, 3],
"bar": [6.0, 7.0, 8.0],
"ham": ["a", "b", "c"],
}
)
print(df.equals(df1))
True
True
null_equal=True
(기본값)으로 설정하면 데이터프레임에 널 값이 있는 경우 동등한 데이터프레임 값으로 인식합니다.
df1 = pl.DataFrame(
{
"foo": [1, 2, None],
"bar": [6.0, 7.0, 8.0],
"ham": ["a", "b", "c"],
}
)
print(df1.equals(df1, null_equal=True))
print(df1.equals(df1, null_equal=False))
df1 = pl.DataFrame(
{
"foo": [1, 2, None],
"bar": [6.0, 7.0, 8.0],
"ham": ["a", "b", "c"],
}
)
print(df1.equals(df1, null_equal=True))
print(df1.equals(df1, null_equal=False))
True
False
True
False
이번에는 특정 컬럼의 값이 동일한지 확인해보도록 하겠습니다. 출력 결과를 보시면 df1의 bar 컬럼의 값과 df2의 bar 컬럼의 값은 동일하기 때문에 True로 출력된 것을 보실 수 있습니다.
df2 = pl.DataFrame(
{
"foo": [3, 2, 1],
"bar": [6.0, 7.0, 8.0],
"ham": ["c", "b", "a"],
}
)
print(df1['bar'].equals(df2['bar']))
df2 = pl.DataFrame(
{
"foo": [3, 2, 1],
"bar": [6.0, 7.0, 8.0],
"ham": ["c", "b", "a"],
}
)
print(df1['bar'].equals(df2['bar']))
True
True
1.1.2 hstack
hstack
메서드는 여러 개의 Series를 수평으로 쌓아 확장된 새로운 데이터프레임을 반환합니다. 이때, in_place=True
로 설정하시면 바로 원본에 반영됩니다. 출력 결과를 보시면 오른쪽에 apple 열이 추가된 것을 보실 수 있습니다.
x = pl.Series("apple", [10, 20, 30])
print(df1.hstack([x]))
x = pl.Series("apple", [10, 20, 30])
print(df1.hstack([x]))
shape: (3, 4)
┌─────┬─────┬─────┬───────┐
│ foo ┆ bar ┆ ham ┆ apple │
│ --- ┆ --- ┆ --- ┆ --- │
│ i64 ┆ f64 ┆ str ┆ i64 │
╞═════╪═════╪═════╪═══════╡
│ 1 ┆ 6.0 ┆ a ┆ 10 │
│ 2 ┆ 7.0 ┆ b ┆ 20 │
│ 3 ┆ 8.0 ┆ c ┆ 30 │
└─────┴─────┴─────┴───────┘
shape: (3, 4)
┌─────┬─────┬─────┬───────┐
│ foo ┆ bar ┆ ham ┆ apple │
│ --- ┆ --- ┆ --- ┆ --- │
│ i64 ┆ f64 ┆ str ┆ i64 │
╞═════╪═════╪═════╪═══════╡
│ 1 ┆ 6.0 ┆ a ┆ 10 │
│ 2 ┆ 7.0 ┆ b ┆ 20 │
│ 3 ┆ 8.0 ┆ c ┆ 30 │
└─────┴─────┴─────┴───────┘
1.1.3 vstack
vstack
메서드는 데이터프레임에 데이터를 수직으로 쌓아서 데이터프레임을 늘립니다. 이때, in_place=True
로 설정하시면 바로 원본에 반영됩니다. 출력 결과를 보시면 아래쪽으로 행의 값이 추가된 것을 보실 수 있습니다.
df2 = pl.DataFrame(
{
"foo": [3, 4],
"bar": [8.0, 9.0],
"ham": ["c", "d"],
}
)
print(df1.vstack(df2))
df2 = pl.DataFrame(
{
"foo": [3, 4],
"bar": [8.0, 9.0],
"ham": ["c", "d"],
}
)
print(df1.vstack(df2))
shape: (5, 3)
┌─────┬─────┬─────┐
│ foo ┆ bar ┆ ham │
│ --- ┆ --- ┆ --- │
│ i64 ┆ f64 ┆ str │
╞═════╪═════╪═════╡
│ 1 ┆ 6.0 ┆ a │
│ 2 ┆ 7.0 ┆ b │
│ 3 ┆ 8.0 ┆ c │
│ 3 ┆ 8.0 ┆ c │
│ 4 ┆ 9.0 ┆ d │
└─────┴─────┴─────┘
shape: (5, 3)
┌─────┬─────┬─────┐
│ foo ┆ bar ┆ ham │
│ --- ┆ --- ┆ --- │
│ i64 ┆ f64 ┆ str │
╞═════╪═════╪═════╡
│ 1 ┆ 6.0 ┆ a │
│ 2 ┆ 7.0 ┆ b │
│ 3 ┆ 8.0 ┆ c │
│ 3 ┆ 8.0 ┆ c │
│ 4 ┆ 9.0 ┆ d │
└─────┴─────┴─────┘
1.1.4 extend
extend
메서드는 vstack과 같이 데이터프레임이 지원하는 메모리를 다른 데이터프레임의 값으로 세로로 확장합니다.
df1 = pl.DataFrame({"foo": [1, 2, 3], "bar": [4, 5, 6]})
df2 = pl.DataFrame({"foo": [10, 20, 30], "bar": [40, 50, 60]})
print(df1.extend(df2))
df1 = pl.DataFrame({"foo": [1, 2, 3], "bar": [4, 5, 6]})
df2 = pl.DataFrame({"foo": [10, 20, 30], "bar": [40, 50, 60]})
print(df1.extend(df2))
shape: (6, 2)
┌─────┬─────┐
│ foo ┆ bar │
│ --- ┆ --- │
│ i64 ┆ i64 │
╞═════╪═════╡
│ 1 ┆ 4 │
│ 2 ┆ 5 │
│ 3 ┆ 6 │
│ 10 ┆ 40 │
│ 20 ┆ 50 │
│ 30 ┆ 60 │
└─────┴─────┘
shape: (6, 2)
┌─────┬─────┐
│ foo ┆ bar │
│ --- ┆ --- │
│ i64 ┆ i64 │
╞═════╪═════╡
│ 1 ┆ 4 │
│ 2 ┆ 5 │
│ 3 ┆ 6 │
│ 10 ┆ 40 │
│ 20 ┆ 50 │
│ 30 ┆ 60 │
└─────┴─────┘
extend
메서드는 다른 데이터프레임의 청크를 기존 데이터프레임의 청크에 추가하는 vstack
과 달리, extend
는 다른 데이터프레임의 데이터를 기본 메모리 위치에 추가하므로 재할당이 발생할 수 있습니다. 재할당이 발생하지 않으면 결과 데이터프레임 구조에 추가 청크가 없으므로 쿼리가 더 빨라집니다.
- 한 번 추가한 후 쿼리를 수행하려는 경우
vstack
보다extend
메서드 사용하시는 것을 권장드립니다. - n개의 행을 추가하고 쿼리를 다시 실행하려는 경우나 쿼리를 수행하기 전에 여러 번 추가하려는 경우
extend
보다vstack
을 추천드립니다. - 여러 파일을 읽어서 단일 데이터프레임에 저장하려는 경우, 리청크를 통해
vstack
메서드를 사용하시길 권장드립니다.
1.1.5 update
update
메서드는 두 데이터프레임에 같은 컬럼명이 있는 경우 해당 열의 데이터프레임의 값을 다른 데이터프레임의 값으로 업데이트합니다.
df의 B열을 new_df의 B열로 업데이트합니다. 만약, 값이 없거나 null 값인 경우 df 값을 유지합니다.
df = pl.DataFrame(
{
"A": [1, 2, 3, 4],
"B": [400, 500, 600, 700],
}
)
new_df = pl.DataFrame(
{
"B": [-66, None, -99],
"C": [5, 3, 1],
}
)
# df.update(새로운데이터프레임)
print(df.update(new_df))
df = pl.DataFrame(
{
"A": [1, 2, 3, 4],
"B": [400, 500, 600, 700],
}
)
new_df = pl.DataFrame(
{
"B": [-66, None, -99],
"C": [5, 3, 1],
}
)
# df.update(새로운데이터프레임)
print(df.update(new_df))
shape: (4, 2)
┌─────┬─────┐
│ A ┆ B │
│ --- ┆ --- │
│ i64 ┆ i64 │
╞═════╪═════╡
│ 1 ┆ -66 │
│ 2 ┆ 500 │
│ 3 ┆ -99 │
│ 4 ┆ 700 │
└─────┴─────┘
shape: (4, 2)
┌─────┬─────┐
│ A ┆ B │
│ --- ┆ --- │
│ i64 ┆ i64 │
╞═════╪═════╡
│ 1 ┆ -66 │
│ 2 ┆ 500 │
│ 3 ┆ -99 │
│ 4 ┆ 700 │
└─────┴─────┘
how
매개변수는 어떤 방식으로 데이터프레임을 업데이트할 지 조인 방식을 지정합니다. inner 조인을 해보도록 하겠습니다. 출력 결과를 보시면 new_df 행이 3개밖에 없기 때문에 3개의 행만 출력됩니다.
# df.update(new_df, how="조인 종류")
print(df.update(new_df, how="inner"))
# df.update(new_df, how="조인 종류")
print(df.update(new_df, how="inner"))
shape: (3, 2)
┌─────┬─────┐
│ A ┆ B │
│ --- ┆ --- │
│ i64 ┆ i64 │
╞═════╪═════╡
│ 1 ┆ -66 │
│ 2 ┆ 500 │
│ 3 ┆ -99 │
└─────┴─────┘
shape: (3, 2)
┌─────┬─────┐
│ A ┆ B │
│ --- ┆ --- │
│ i64 ┆ i64 │
╞═════╪═════╡
│ 1 ┆ -66 │
│ 2 ┆ 500 │
│ 3 ┆ -99 │
└─────┴─────┘
- left : 왼쪽 테이블의 모든 행을 유지하며, 오른쪽 테이블의 매칭되는 값만 업데이트하고 나머지는 원래 값을 유지합니다. 만약, 여러번 매칭되면 행이 중복될 수 있습니다.
- inner : 두 데이터프레임에서 공통으로 존재하는 값, null이 아닌 매칭되는 값만 결과에 포함됩니다.
- full : 왼쪽 테이블의 모든 행을 유지하며, 오른쪽 테이블의 매칭되는 값만 업데이트하고 새로운 데이터가 있는 경우 행에 추가합니다.
이번에는 각 데이터프레임에서 명시적으로 조인할 열을 설정하여 값을 업데이트합니다. B의 열을 기준으로 full join을 해보도록 하겠습니다.
# df.update(새로운데이터프레임, on=["컬럼명"], how="조인 종류")
print(df.update(new_df, on=["B"], how="full"))
# df.update(새로운데이터프레임, on=["컬럼명"], how="조인 종류")
print(df.update(new_df, on=["B"], how="full"))
- on : 조인할 컬럼명, 두 데이터프레임에 해당 컬럼명이 존재해야 합니다. 없음(기본값)으로 설정하면 각 데이터프레임의 암묵적인 행 인덱스가 조인 키로 사용됩니다.
shape: (7, 2)
┌──────┬──────┐
│ A ┆ B │
│ --- ┆ --- │
│ i64 ┆ i64 │
╞══════╪══════╡
│ 1 ┆ 400 │
│ 2 ┆ 500 │
│ 3 ┆ 600 │
│ 4 ┆ 700 │
│ null ┆ null │
│ null ┆ -66 │
│ null ┆ -99 │
└──────┴──────┘
shape: (7, 2)
┌──────┬──────┐
│ A ┆ B │
│ --- ┆ --- │
│ i64 ┆ i64 │
╞══════╪══════╡
│ 1 ┆ 400 │
│ 2 ┆ 500 │
│ 3 ┆ 600 │
│ 4 ┆ 700 │
│ null ┆ null │
│ null ┆ -66 │
│ null ┆ -99 │
└──────┴──────┘
왼쪽 데이터프레임의 A 열의 값과 오른쪽 데이터프레임의 C 열의 값이 같은 행을 찾아 해당 행의 B 값을 업데이트해보도록 하겠습니다.
# df.update(새로운데이터프레임, left_on=["기존 데이터프레임 컬럼명"], right_on=["새로운 데이터프레임 컬럼명"], how="조인 종류")
print(df.update(new_df, left_on=["A"], right_on=["C"], how="full"))
# df.update(새로운데이터프레임, left_on=["기존 데이터프레임 컬럼명"], right_on=["새로운 데이터프레임 컬럼명"], how="조인 종류")
print(df.update(new_df, left_on=["A"], right_on=["C"], how="full"))
- left_on : 왼쪽 데이터프레임의 열을 기준으로 조인합니다.
- right_on : 오른쪽 데이터프레임의 열을 기준으로 조인합니다.
shape: (5, 2)
┌─────┬─────┐
│ A ┆ B │
│ --- ┆ --- │
│ i64 ┆ i64 │
╞═════╪═════╡
│ 1 ┆ -99 │
│ 2 ┆ 500 │
│ 3 ┆ 600 │
│ 4 ┆ 700 │
│ 5 ┆ -66 │
└─────┴─────┘
shape: (5, 2)
┌─────┬─────┐
│ A ┆ B │
│ --- ┆ --- │
│ i64 ┆ i64 │
╞═════╪═════╡
│ 1 ┆ -99 │
│ 2 ┆ 500 │
│ 3 ┆ 600 │
│ 4 ┆ 700 │
│ 5 ┆ -66 │
└─────┴─────┘
include_nulls
매개변수는 left/inner 조인을 위한 매개변수로 True로 설정하면 null 값을 포함하여 값 업데이트합니다. 이때, 왼쪽 데이터프레임의 값을 오른쪽 데이터프레임의 null 값으로 덮어씁니다. False(기본값)로 설정하면 오른쪽 데이터프레임의 null 값은 무시됩니다.
print(df.update(new_df, left_on="A", right_on="C", how="full", include_nulls=True))
print(df.update(new_df, left_on="A", right_on="C", how="full", include_nulls=True))
shape: (5, 2)
┌─────┬──────┐
│ A ┆ B │
│ --- ┆ --- │
│ i64 ┆ i64 │
╞═════╪══════╡
│ 1 ┆ -99 │
│ 2 ┆ 500 │
│ 3 ┆ null │
│ 4 ┆ 700 │
│ 5 ┆ -66 │
└─────┴──────┘
shape: (5, 2)
┌─────┬──────┐
│ A ┆ B │
│ --- ┆ --- │
│ i64 ┆ i64 │
╞═════╪══════╡
│ 1 ┆ -99 │
│ 2 ┆ 500 │
│ 3 ┆ null │
│ 4 ┆ 700 │
│ 5 ┆ -66 │
└─────┴──────┘
1.1.6 join
join
메서드는 두 데이터프레임을 특정 컬럼 기준으로 조인합니다. df와 other_df를 ham 열을 기준으로 조인해보도록 하겠습니다. 출력 결과를 보시면 ham의 열에서 공통으로 존재하는 값의 행만 출력된 것을 보실 수 있습니다.
df = pl.DataFrame(
{
"foo": [1, 2, 3],
"bar": [6.0, 7.0, 8.0],
"ham": ["a", "b", "c"],
}
)
other_df = pl.DataFrame(
{
"apple": ["x", "y", "z"],
"ham": ["a", "b", "d"],
}
)
# df.join(새로운 데이터프레임, on="컬럼명")
print(df.join(other_df, on="ham"))
df = pl.DataFrame(
{
"foo": [1, 2, 3],
"bar": [6.0, 7.0, 8.0],
"ham": ["a", "b", "c"],
}
)
other_df = pl.DataFrame(
{
"apple": ["x", "y", "z"],
"ham": ["a", "b", "d"],
}
)
# df.join(새로운 데이터프레임, on="컬럼명")
print(df.join(other_df, on="ham"))
shape: (2, 4)
┌─────┬─────┬─────┬───────┐
│ foo ┆ bar ┆ ham ┆ apple │
│ --- ┆ --- ┆ --- ┆ --- │
│ i64 ┆ f64 ┆ str ┆ str │
╞═════╪═════╪═════╪═══════╡
│ 1 ┆ 6.0 ┆ a ┆ x │
│ 2 ┆ 7.0 ┆ b ┆ y │
└─────┴─────┴─────┴───────┘
shape: (2, 4)
┌─────┬─────┬─────┬───────┐
│ foo ┆ bar ┆ ham ┆ apple │
│ --- ┆ --- ┆ --- ┆ --- │
│ i64 ┆ f64 ┆ str ┆ str │
╞═════╪═════╪═════╪═══════╡
│ 1 ┆ 6.0 ┆ a ┆ x │
│ 2 ┆ 7.0 ┆ b ┆ y │
└─────┴─────┴─────┴───────┘
만약, 조인할 열의 이름이 다를 경우 on이 아닌 left_on, right_on을 사용하셔야 합니다. df2와 other_df2를 조인할 때, df의 ham1 열과 other_df2의 ham2 열을 기준으로 조인해보도록 하겠습니다.
df2 = pl.DataFrame(
{
"foo": [1, 2, 3],
"bar": [6.0, 7.0, 8.0],
"ham1": ["a", "b", "c"],
}
)
other_df2 = pl.DataFrame(
{
"apple": ["x", "y", "z"],
"ham2": ["a", "b", "d"],
}
)
# df.join(새로운 데이터프레임, left_on="왼쪽 데이터프레임의 컬럼명", right_on="오른쪽 데이터프레임의 컬럼명")
print(df2.join(other_df2, left_on="ham1", right_on="ham2"))
df2 = pl.DataFrame(
{
"foo": [1, 2, 3],
"bar": [6.0, 7.0, 8.0],
"ham1": ["a", "b", "c"],
}
)
other_df2 = pl.DataFrame(
{
"apple": ["x", "y", "z"],
"ham2": ["a", "b", "d"],
}
)
# df.join(새로운 데이터프레임, left_on="왼쪽 데이터프레임의 컬럼명", right_on="오른쪽 데이터프레임의 컬럼명")
print(df2.join(other_df2, left_on="ham1", right_on="ham2"))
shape: (2, 4)
┌─────┬─────┬──────┬───────┐
│ foo ┆ bar ┆ ham1 ┆ apple │
│ --- ┆ --- ┆ --- ┆ --- │
│ i64 ┆ f64 ┆ str ┆ str │
╞═════╪═════╪══════╪═══════╡
│ 1 ┆ 6.0 ┆ a ┆ x │
│ 2 ┆ 7.0 ┆ b ┆ y │
└─────┴─────┴──────┴───────┘
shape: (2, 4)
┌─────┬─────┬──────┬───────┐
│ foo ┆ bar ┆ ham1 ┆ apple │
│ --- ┆ --- ┆ --- ┆ --- │
│ i64 ┆ f64 ┆ str ┆ str │
╞═════╪═════╪══════╪═══════╡
│ 1 ┆ 6.0 ┆ a ┆ x │
│ 2 ┆ 7.0 ┆ b ┆ y │
└─────┴─────┴──────┴───────┘
두 데이터프레임을 어떻게 조인할지 조인 방법을 정할 수 있습니다. df와 other_df를 full join해보도록 하겠습니다.
# df.join(other_df, on="컬럼명", how="조인방법")
print(df.join(other_df, on="ham", how="full"))
# df.join(other_df, on="컬럼명", how="조인방법")
print(df.join(other_df, on="ham", how="full"))
Polars에서 옵션 없이 join을 사용할 경우 기본 설정(default)는 Inner Join이 됩니다. key 값으로 병렬할 기준을 선택할 수 있지만 key 값 생략의 경우, 동일한 컬럼명을 기준으로 join합니다.
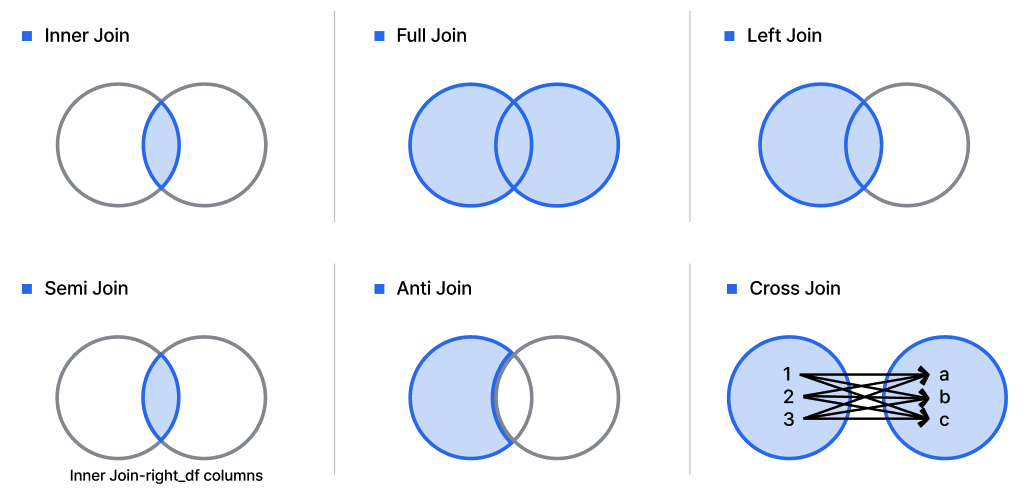
- inner : 두 데이터프레임의 중복된 요소만을 join 해줍니다.
- full : 두 데이터프레임의 모든 요소를 join 해줍니다.
- left : 두 데이터프레임 중 왼쪽 데이터 셋을 기준으로 join 해줍니다. 왼쪽 데이터프레임의 모든 행과 오른쪽 데이터프레임의 일치하는 행을 반환합니다. 출력 결과는 왼쪽 데이터프레임의 행 순서를 유지합니다.
- semi : 왼쪽 데이터프레임에서 오른쪽 데이터프레임에 일치하는 행이 있는 행을 반환합니다.
- anti : 왼쪽 데이터프레임에서 오른쪽 데이터프레임에 일치하는 항목이 없는 행을 반환합니다.
- cross : 두 데이터프레임의 행의 데카르트 곱을 반환합니다. 이때, on 매개변수는 사용하지 않습니다.
shape: (4, 5)
┌──────┬──────┬──────┬───────┬───────────┐
│ foo ┆ bar ┆ ham ┆ apple ┆ ham_right │
│ --- ┆ --- ┆ --- ┆ --- ┆ --- │
│ i64 ┆ f64 ┆ str ┆ str ┆ str │
╞══════╪══════╪══════╪═══════╪═══════════╡
│ 1 ┆ 6.0 ┆ a ┆ x ┆ a │
│ 2 ┆ 7.0 ┆ b ┆ y ┆ b │
│ null ┆ null ┆ null ┆ z ┆ d │
│ 3 ┆ 8.0 ┆ c ┆ null ┆ null │
└──────┴──────┴──────┴───────┴───────────┘
shape: (4, 5)
┌──────┬──────┬──────┬───────┬───────────┐
│ foo ┆ bar ┆ ham ┆ apple ┆ ham_right │
│ --- ┆ --- ┆ --- ┆ --- ┆ --- │
│ i64 ┆ f64 ┆ str ┆ str ┆ str │
╞══════╪══════╪══════╪═══════╪═══════════╡
│ 1 ┆ 6.0 ┆ a ┆ x ┆ a │
│ 2 ┆ 7.0 ┆ b ┆ y ┆ b │
│ null ┆ null ┆ null ┆ z ┆ d │
│ 3 ┆ 8.0 ┆ c ┆ null ┆ null │
└──────┴──────┴──────┴───────┴───────────┘
print(df.join(other_df, on="ham", how="semi"))
print(df.join(other_df, on="ham", how="semi"))
shape: (2, 3)
┌─────┬─────┬─────┐
│ foo ┆ bar ┆ ham │
│ --- ┆ --- ┆ --- │
│ i64 ┆ f64 ┆ str │
╞═════╪═════╪═════╡
│ 1 ┆ 6.0 ┆ a │
│ 2 ┆ 7.0 ┆ b │
└─────┴─────┴─────┘
shape: (2, 3)
┌─────┬─────┬─────┐
│ foo ┆ bar ┆ ham │
│ --- ┆ --- ┆ --- │
│ i64 ┆ f64 ┆ str │
╞═════╪═════╪═════╡
│ 1 ┆ 6.0 ┆ a │
│ 2 ┆ 7.0 ┆ b │
└─────┴─────┴─────┘
print(df.join(other_df, on="ham", how="anti"))
print(df.join(other_df, on="ham", how="anti"))
shape: (1, 3)
┌─────┬─────┬─────┐
│ foo ┆ bar ┆ ham │
│ --- ┆ --- ┆ --- │
│ i64 ┆ f64 ┆ str │
╞═════╪═════╪═════╡
│ 3 ┆ 8.0 ┆ c │
└─────┴─────┴─────┘
shape: (1, 3)
┌─────┬─────┬─────┐
│ foo ┆ bar ┆ ham │
│ --- ┆ --- ┆ --- │
│ i64 ┆ f64 ┆ str │
╞═════╪═════╪═════╡
│ 3 ┆ 8.0 ┆ c │
└─────┴─────┴─────┘
full, cross 조인 결과를 보면 컬럼명_right 라고 새로운 컬럼이 만들어진 것을 볼 수 있습니다. 만약, 컬럼명_right가 아닌 다른 접미사를 추가하고 싶다면 suffix
를 이용하시면 됩니다. 출력 결과를 보시면 접미사가 변경된 것을 보실 수 있습니다.
print(df.join(other_df, on="ham", how="full", suffix="_new"))
print(df.join(other_df, on="ham", how="full", suffix="_new"))
shape: (4, 5)
┌──────┬──────┬──────┬───────┬─────────┐
│ foo ┆ bar ┆ ham ┆ apple ┆ ham_new │
│ --- ┆ --- ┆ --- ┆ --- ┆ --- │
│ i64 ┆ f64 ┆ str ┆ str ┆ str │
╞══════╪══════╪══════╪═══════╪═════════╡
│ 1 ┆ 6.0 ┆ a ┆ x ┆ a │
│ 2 ┆ 7.0 ┆ b ┆ y ┆ b │
│ null ┆ null ┆ null ┆ z ┆ d │
│ 3 ┆ 8.0 ┆ c ┆ null ┆ null │
└──────┴──────┴──────┴───────┴─────────┘
shape: (4, 5)
┌──────┬──────┬──────┬───────┬─────────┐
│ foo ┆ bar ┆ ham ┆ apple ┆ ham_new │
│ --- ┆ --- ┆ --- ┆ --- ┆ --- │
│ i64 ┆ f64 ┆ str ┆ str ┆ str │
╞══════╪══════╪══════╪═══════╪═════════╡
│ 1 ┆ 6.0 ┆ a ┆ x ┆ a │
│ 2 ┆ 7.0 ┆ b ┆ y ┆ b │
│ null ┆ null ┆ null ┆ z ┆ d │
│ 3 ┆ 8.0 ┆ c ┆ null ┆ null │
└──────┴──────┴──────┴───────┴─────────┘
coalesce=True
로 설정하여 조인 열을 병합합니다. 만약, 계산식에 의해 조인한다면 병합이 해제됩니다. False(기본값, None)로 설정할 경우 조인 열을 병합하지 않습니다. 출력 결과를 보시면 df의 ham 열과 other_df의 ham 열이 병합된 것을 보실 수 있습니다.
print(df.join(other_df, on="ham", how="left", coalesce=True))
print(df.join(other_df, on="ham", how="left", coalesce=True))
shape: (3, 4)
┌─────┬─────┬─────┬───────┐
│ foo ┆ bar ┆ ham ┆ apple │
│ --- ┆ --- ┆ --- ┆ --- │
│ i64 ┆ f64 ┆ str ┆ str │
╞═════╪═════╪═════╪═══════╡
│ 1 ┆ 6.0 ┆ a ┆ x │
│ 2 ┆ 7.0 ┆ b ┆ y │
│ 3 ┆ 8.0 ┆ c ┆ null │
└─────┴─────┴─────┴───────┘
shape: (3, 4)
┌─────┬─────┬─────┬───────┐
│ foo ┆ bar ┆ ham ┆ apple │
│ --- ┆ --- ┆ --- ┆ --- │
│ i64 ┆ f64 ┆ str ┆ str │
╞═════╪═════╪═════╪═══════╡
│ 1 ┆ 6.0 ┆ a ┆ x │
│ 2 ┆ 7.0 ┆ b ┆ y │
│ 3 ┆ 8.0 ┆ c ┆ null │
└─────┴─────┴─────┴───────┘
범주형 데이터가 있는 열에 대한 조인은 polars.StringCache
를 사용하시길 바랍니다.
# 두 데이터프레임에 범주형 데이터가 있는 경우
df1 = pl.DataFrame({
"id": [1, 2, 3],
"category": ["A", "B", "A"] # 범주형 데이터
})
df2 = pl.DataFrame({
"id": [1, 2, 4],
"category": ["A", "C", "B"] # 범주형 데이터
})
# 범주형 데이터를 StringCache를 사용하여 변환
with pl.StringCache():
# 각 데이터프레임의 category 열을 범주형으로 변환
df1 = df1.with_columns(pl.col("category").cast(pl.Categorical))
df2 = df2.with_columns(pl.col("category").cast(pl.Categorical))
result = df1.join(df2, on="category", how="left")
print(result)
# 두 데이터프레임에 범주형 데이터가 있는 경우
df1 = pl.DataFrame({
"id": [1, 2, 3],
"category": ["A", "B", "A"] # 범주형 데이터
})
df2 = pl.DataFrame({
"id": [1, 2, 4],
"category": ["A", "C", "B"] # 범주형 데이터
})
# 범주형 데이터를 StringCache를 사용하여 변환
with pl.StringCache():
# 각 데이터프레임의 category 열을 범주형으로 변환
df1 = df1.with_columns(pl.col("category").cast(pl.Categorical))
df2 = df2.with_columns(pl.col("category").cast(pl.Categorical))
result = df1.join(df2, on="category", how="left")
print(result)
- 메모리 효율성: 문자열을 정수로 인코딩하여 메모리 사용을 줄임
- 성능 향상: 문자열 비교 대신 정수 비교를 수행하여 조인 성능 향상
- 일관성 유지: 서로 다른 데이터프레임 간에 범주형 데이터의 매핑을 일관되게 유지
특히 대용량 데이터에서 범주형 열을 기준으로 조인할 때 StringCache
를 사용하면 성능이 크게 향상될 수 있습니다.
join_nulls=True
로 설정하여 null 값끼리 조인을 허용합니다. False(기본값)은 null 값끼리 조인을 수행하지 않습니다.
df = pl.DataFrame(
{
"foo": [1, 2, 3],
"bar": [6.0, 7.0, 8.0],
"ham": ["a", None, "c"],
}
)
other_df = pl.DataFrame(
{
"apple": ["x", "y", "z"],
"ham": ["a", None, "d"],
}
)
print(df.join(other_df, on="ham", how="full", join_nulls=True))
df = pl.DataFrame(
{
"foo": [1, 2, 3],
"bar": [6.0, 7.0, 8.0],
"ham": ["a", None, "c"],
}
)
other_df = pl.DataFrame(
{
"apple": ["x", "y", "z"],
"ham": ["a", None, "d"],
}
)
print(df.join(other_df, on="ham", how="full", join_nulls=True))
shape: (4, 5)
┌──────┬──────┬──────┬───────┬───────────┐
│ foo ┆ bar ┆ ham ┆ apple ┆ ham_right │
│ --- ┆ --- ┆ --- ┆ --- ┆ --- │
│ i64 ┆ f64 ┆ str ┆ str ┆ str │
╞══════╪══════╪══════╪═══════╪═══════════╡
│ 1 ┆ 6.0 ┆ a ┆ x ┆ a │
│ 2 ┆ 7.0 ┆ null ┆ y ┆ null │
│ null ┆ null ┆ null ┆ z ┆ d │
│ 3 ┆ 8.0 ┆ c ┆ null ┆ null │
└──────┴──────┴──────┴───────┴───────────┘
shape: (4, 5)
┌──────┬──────┬──────┬───────┬───────────┐
│ foo ┆ bar ┆ ham ┆ apple ┆ ham_right │
│ --- ┆ --- ┆ --- ┆ --- ┆ --- │
│ i64 ┆ f64 ┆ str ┆ str ┆ str │
╞══════╪══════╪══════╪═══════╪═══════════╡
│ 1 ┆ 6.0 ┆ a ┆ x ┆ a │
│ 2 ┆ 7.0 ┆ null ┆ y ┆ null │
│ null ┆ null ┆ null ┆ z ┆ d │
│ 3 ┆ 8.0 ┆ c ┆ null ┆ null │
└──────┴──────┴──────┴───────┴───────────┘
# print(df.join(other_df, on="ham", how="full"))
print(df.join(other_df, on="ham", how="full", join_nulls=False))
# print(df.join(other_df, on="ham", how="full"))
print(df.join(other_df, on="ham", how="full", join_nulls=False))
shape: (5, 5)
┌──────┬──────┬──────┬───────┬───────────┐
│ foo ┆ bar ┆ ham ┆ apple ┆ ham_right │
│ --- ┆ --- ┆ --- ┆ --- ┆ --- │
│ i64 ┆ f64 ┆ str ┆ str ┆ str │
╞══════╪══════╪══════╪═══════╪═══════════╡
│ 1 ┆ 6.0 ┆ a ┆ x ┆ a │
│ null ┆ null ┆ null ┆ y ┆ null │
│ null ┆ null ┆ null ┆ z ┆ d │
│ 3 ┆ 8.0 ┆ c ┆ null ┆ null │
│ 2 ┆ 7.0 ┆ null ┆ null ┆ null │
└──────┴──────┴──────┴───────┴───────────┘
shape: (5, 5)
┌──────┬──────┬──────┬───────┬───────────┐
│ foo ┆ bar ┆ ham ┆ apple ┆ ham_right │
│ --- ┆ --- ┆ --- ┆ --- ┆ --- │
│ i64 ┆ f64 ┆ str ┆ str ┆ str │
╞══════╪══════╪══════╪═══════╪═══════════╡
│ 1 ┆ 6.0 ┆ a ┆ x ┆ a │
│ null ┆ null ┆ null ┆ y ┆ null │
│ null ┆ null ┆ null ┆ z ┆ d │
│ 3 ┆ 8.0 ┆ c ┆ null ┆ null │
│ 2 ┆ 7.0 ┆ null ┆ null ┆ null │
└──────┴──────┴──────┴───────┴───────────┘
validate
는 두 데이터프레임 관계가 조인 유형과 동일한지 확인합니다. 만약, 다른 경우 에러가 나게 됩니다.
df = pl.DataFrame(
{
"foo": [1, 2, 3],
"bar": [6.0, 7.0, 8.0],
"ham": ["a", "a" , "c"],
}
)
other_df = pl.DataFrame(
{
"apple": ["x", "y", "z"],
"ham": ["a", "a", "d"],
}
)
print(df.join(other_df, on="ham", how="full", validate="m:m"))
# print(df.join(other_df, on="ham", how="full", validate="1:m"))
# print(df.join(other_df, on="ham", how="full", validate="m:1"))
# print(df.join(other_df, on="ham", how="full", validate="1:1"))
df = pl.DataFrame(
{
"foo": [1, 2, 3],
"bar": [6.0, 7.0, 8.0],
"ham": ["a", "a" , "c"],
}
)
other_df = pl.DataFrame(
{
"apple": ["x", "y", "z"],
"ham": ["a", "a", "d"],
}
)
print(df.join(other_df, on="ham", how="full", validate="m:m"))
# print(df.join(other_df, on="ham", how="full", validate="1:m"))
# print(df.join(other_df, on="ham", how="full", validate="m:1"))
# print(df.join(other_df, on="ham", how="full", validate="1:1"))
shape: (6, 5)
┌──────┬──────┬──────┬───────┬───────────┐
│ foo ┆ bar ┆ ham ┆ apple ┆ ham_right │
│ --- ┆ --- ┆ --- ┆ --- ┆ --- │
│ i64 ┆ f64 ┆ str ┆ str ┆ str │
╞══════╪══════╪══════╪═══════╪═══════════╡
│ 1 ┆ 6.0 ┆ a ┆ x ┆ a │
│ 2 ┆ 7.0 ┆ a ┆ x ┆ a │
│ 1 ┆ 6.0 ┆ a ┆ y ┆ a │
│ 2 ┆ 7.0 ┆ a ┆ y ┆ a │
│ null ┆ null ┆ null ┆ z ┆ d │
│ 3 ┆ 8.0 ┆ c ┆ null ┆ null │
└──────┴──────┴──────┴───────┴───────────┘
ComputeError: the join keys did not fulfil 1:m validation
ComputeError: the join keys did not fulfil m:1 validation
ComputeError: the join keys did not fulfil 1:1 validation
shape: (6, 5)
┌──────┬──────┬──────┬───────┬───────────┐
│ foo ┆ bar ┆ ham ┆ apple ┆ ham_right │
│ --- ┆ --- ┆ --- ┆ --- ┆ --- │
│ i64 ┆ f64 ┆ str ┆ str ┆ str │
╞══════╪══════╪══════╪═══════╪═══════════╡
│ 1 ┆ 6.0 ┆ a ┆ x ┆ a │
│ 2 ┆ 7.0 ┆ a ┆ x ┆ a │
│ 1 ┆ 6.0 ┆ a ┆ y ┆ a │
│ 2 ┆ 7.0 ┆ a ┆ y ┆ a │
│ null ┆ null ┆ null ┆ z ┆ d │
│ 3 ┆ 8.0 ┆ c ┆ null ┆ null │
└──────┴──────┴──────┴───────┴───────────┘
ComputeError: the join keys did not fulfil 1:m validation
ComputeError: the join keys did not fulfil m:1 validation
ComputeError: the join keys did not fulfil 1:1 validation
- m
(many_to_many) : 기본값, 다대다 관계인지 확인합니다. 양쪽 데이터프레임 조인 키가 고유하지 않습니다. left_df join_columns n a 1 a 3 c 2 right_df join_columns n ------------ --- a 1 a 2 e 3 - 1
(one_to_many) : 왼쪽 데이터 셋에서 조인 키가 고유한지 확인합니다. left_df join_columns n a 1 b 3 c 2 right_df join_columns n ------------ --- a 1 a 2 e 3 - m:1(many_to_one) : 오른쪽 데이터 셋에서 조인 키가 고유한지 확인합니다.
left_df
join_columns n a 1 a 3 c 2 right_df join_columns n ------------ --- a 1 d 2 e 3 - 1:1(one_to_one) : 왼쪽 및 오른쪽 데이터 셋 모두에서 조인 키가 고유한지 확인합니다.
left_df
join_columns n a 1 b 3 c 2 right_df join_columns n ------------ --- a 1 d 2 e 3
1.1.7 join_asof
ASOF(As-Of) 조인은 두 테이블을 병합하는 방법 중 하나로, 특히 시계열 데이터에서 유용합니다. ASOF 조인은 기준 열에서 값이 정확히 일치하지 않더라도 가장 가까운 이전 값과 매칭되는 조인 키를 찾습니다. 이는 시계열 데이터의 비동기적 특성을 처리하는데 유용합니다.
동일한 조인 키가 아닌 가장 가까운 조인 키를 기준으로 매칭한다는 점을 제외하면 기존 조인 방법과 비슷합니다. 이때, 두 데이터프레임 모두 조인 키를 기준으로 정렬되어야 합니다.
from datetime import date
gdp = pl.DataFrame(
{
"date": pl.date_range(
date(2016, 1, 1),
date(2020, 1, 1),
"1y",
eager=True,
),
"gdp": [4164, 4411, 4566, 4696, 4827],
}
)
population = pl.DataFrame(
{
"date": [date(2016, 3, 1), date(2018, 8, 1), date(2019, 1, 1)],
"population": [82.19, 82.66, 83.12],
}
).sort("date")
from datetime import date
gdp = pl.DataFrame(
{
"date": pl.date_range(
date(2016, 1, 1),
date(2020, 1, 1),
"1y",
eager=True,
),
"gdp": [4164, 4411, 4566, 4696, 4827],
}
)
population = pl.DataFrame(
{
"date": [date(2016, 3, 1), date(2018, 8, 1), date(2019, 1, 1)],
"population": [82.19, 82.66, 83.12],
}
).sort("date")
- date_range(시작날짜, 종료날짜, 간격, eager) : 시작 날짜부터 종료 날짜까지 일정한 간격으로 날짜 시퀀스를 생성합니다.
eager=True
: 결과를 즉시 계산하여 메모리에 저장
# df.join_asof(df2, on="컬럼명", strategy="조인 방법")
print(population.join_asof(gdp, on="date", strategy="backward"))
# df.join_asof(df2, on="컬럼명", strategy="조인 방법")
print(population.join_asof(gdp, on="date", strategy="backward"))
- on: 두 데이터프레임의 조인할 컬럼명입니다. 만약, 두 데이터프레임의 조인할 컬럼명이 다를 경우 join에서와 같이 on 대신 left_on, right_on을 사용하여 각각의 조인할 컬럼명을 입력합니다.
- strategy: 어떤 방법으로 조인할지 설정합니다.
- backward(기본값) : 각 행에 대해 오른쪽 데이터프레임의 행의 값이 왼쪽 데이터프레임의 행의 값보다 작거나 같은 마지막 행을 선택합니다.
- forward : 각 행에 대해 오른쪽 데이터프레임의 행의 값이 왼쪽 데이터프레임의 행의 값보다 크거나 같은 첫 번째 행을 선택합니다.
- nearest : 각 행에 대해 오른쪽 데이터프레임의 행의 값이 왼쪽 데이터프레임의 행의 값에 가장 가까운 값을 가진 마지막 행을 선택합니다. nearest에는 데이터 타입이 문자열인 경우 ASOF 조인이 지원되지 않습니다.
출력 결과를 확인해보시면 날짜가 정확히 일치하지 않는 population의 각 날짜는 gdp에서 가장 가까운 이전 날짜와 매칭된 것을 볼 수 있습니다.
shape: (3, 3)
┌────────────┬────────────┬──────┐
│ date ┆ population ┆ gdp │
│ --- ┆ --- ┆ --- │
│ date ┆ f64 ┆ i64 │
╞════════════╪════════════╪══════╡
│ 2016-03-01 ┆ 82.19 ┆ 4164 │
│ 2018-08-01 ┆ 82.66 ┆ 4566 │
│ 2019-01-01 ┆ 83.12 ┆ 4696 │
└────────────┴────────────┴──────┘
shape: (3, 3)
┌────────────┬────────────┬──────┐
│ date ┆ population ┆ gdp │
│ --- ┆ --- ┆ --- │
│ date ┆ f64 ┆ i64 │
╞════════════╪════════════╪══════╡
│ 2016-03-01 ┆ 82.19 ┆ 4164 │
│ 2018-08-01 ┆ 82.66 ┆ 4566 │
│ 2019-01-01 ┆ 83.12 ┆ 4696 │
└────────────┴────────────┴──────┘
strategy=’forward’
로 설정하면, population의 2016-03-01 날짜는 gdp의 2016-01-01과 매칭되고, population의 2018-08-01 날짜는 gdp의 2018-01-01과 매칭됩니다. 이처럼 정확히 일치하지 않는 population의 각 날짜는 gdp에서 가장 가까운 이후의 날짜와 매칭됩니다.
print(population.join_asof(gdp, on="date", strategy="forward"))
print(population.join_asof(gdp, on="date", strategy="forward"))
shape: (3, 3)
┌────────────┬────────────┬──────┐
│ date ┆ population ┆ gdp │
│ --- ┆ --- ┆ --- │
│ date ┆ f64 ┆ i64 │
╞════════════╪════════════╪══════╡
│ 2016-03-01 ┆ 82.19 ┆ 4411 │
│ 2018-08-01 ┆ 82.66 ┆ 4696 │
│ 2019-01-01 ┆ 83.12 ┆ 4696 │
└────────────┴────────────┴──────┘
shape: (3, 3)
┌────────────┬────────────┬──────┐
│ date ┆ population ┆ gdp │
│ --- ┆ --- ┆ --- │
│ date ┆ f64 ┆ i64 │
╞════════════╪════════════╪══════╡
│ 2016-03-01 ┆ 82.19 ┆ 4411 │
│ 2018-08-01 ┆ 82.66 ┆ 4696 │
│ 2019-01-01 ┆ 83.12 ┆ 4696 │
└────────────┴────────────┴──────┘
strategy=’nearest’
로 설정하면, population의 2016-03-01 날짜는 gdp의 2017-01-01과 매칭되고, population의 2018-08-01 날짜는 gdp의 2019-01-01과 매칭됩니다. 이처럼 정확히 일치하지 않는 population의 각 날짜가 이전이든 이후든 상관없이 gdp에서 가장 가까운 날짜와 매칭되므로 위의 두 결과를 혼합하여 제공합니다.
print(population.join_asof(gdp, on="date", strategy="nearest"))
print(population.join_asof(gdp, on="date", strategy="nearest"))
shape: (3, 3)
┌────────────┬────────────┬──────┐
│ date ┆ population ┆ gdp │
│ --- ┆ --- ┆ --- │
│ date ┆ f64 ┆ i64 │
╞════════════╪════════════╪══════╡
│ 2016-03-01 ┆ 82.19 ┆ 4164 │
│ 2018-08-01 ┆ 82.66 ┆ 4696 │
│ 2019-01-01 ┆ 83.12 ┆ 4696 │
└────────────┴────────────┴──────┘
shape: (3, 3)
┌────────────┬────────────┬──────┐
│ date ┆ population ┆ gdp │
│ --- ┆ --- ┆ --- │
│ date ┆ f64 ┆ i64 │
╞════════════╪════════════╪══════╡
│ 2016-03-01 ┆ 82.19 ┆ 4164 │
│ 2018-08-01 ┆ 82.66 ┆ 4696 │
│ 2019-01-01 ┆ 83.12 ┆ 4696 │
└────────────┴────────────┴──────┘
by
매개변수를 사용하면 asof 조인 전에 다른 열에 먼저 조인할 수 있습니다. 국가별로 먼저 조인한 다음 날짜별로 asof 조인합니다.
gdp_dates = pl.date_range( # fmt: skip
date(2016, 1, 1), date(2020, 1, 1), "1y", eager=True
)
gdp2 = pl.DataFrame(
{
"country": ["Germany"] * 5 + ["Netherlands"] * 5,
"date": pl.concat([gdp_dates, gdp_dates]),
"gdp": [4164, 4411, 4566, 4696, 4827, 784, 833, 914, 910, 909],
}
).sort("country", "date")
pop2 = pl.DataFrame(
{
"country": ["Germany"] * 3 + ["Netherlands"] * 3,
"date": [
date(2016, 3, 1),
date(2018, 8, 1),
date(2019, 1, 1),
date(2016, 3, 1),
date(2018, 8, 1),
date(2019, 1, 1),
],
"population": [82.19, 82.66, 83.12, 17.11, 17.32, 17.40],
}
).sort("country", "date")
# df.join_asof(df2, by="첫번째 조인 컬럼명", on="두번째 조인 컬럼명", strategy="조인방법")
print(pop2.join_asof(gdp2, by="country", on="date", strategy="nearest"))
gdp_dates = pl.date_range( # fmt: skip
date(2016, 1, 1), date(2020, 1, 1), "1y", eager=True
)
gdp2 = pl.DataFrame(
{
"country": ["Germany"] * 5 + ["Netherlands"] * 5,
"date": pl.concat([gdp_dates, gdp_dates]),
"gdp": [4164, 4411, 4566, 4696, 4827, 784, 833, 914, 910, 909],
}
).sort("country", "date")
pop2 = pl.DataFrame(
{
"country": ["Germany"] * 3 + ["Netherlands"] * 3,
"date": [
date(2016, 3, 1),
date(2018, 8, 1),
date(2019, 1, 1),
date(2016, 3, 1),
date(2018, 8, 1),
date(2019, 1, 1),
],
"population": [82.19, 82.66, 83.12, 17.11, 17.32, 17.40],
}
).sort("country", "date")
# df.join_asof(df2, by="첫번째 조인 컬럼명", on="두번째 조인 컬럼명", strategy="조인방법")
print(pop2.join_asof(gdp2, by="country", on="date", strategy="nearest"))
출력 결과를 보시면 population의 국가가 Germany 2016-03-01, 날짜는 gdp의 국가가 Germany 2016-01-01과 매칭되고, population의 국가가 Germany 2018-08-01 날짜는 gdp의 국가가 Germany 2019-01-01과 매칭됩니다.
on 매개변수와 마찬가지로 두 데이터프레임의 컬럼명이 다를 경우 by_left, by_right 매개변수에 각각의 컬럼명을 입력합니다.
shape: (6, 4)
┌─────────────┬────────────┬────────────┬──────┐
│ country ┆ date ┆ population ┆ gdp │
│ --- ┆ --- ┆ --- ┆ --- │
│ str ┆ date ┆ f64 ┆ i64 │
╞═════════════╪════════════╪════════════╪══════╡
│ Germany ┆ 2016-03-01 ┆ 82.19 ┆ 4164 │
│ Germany ┆ 2018-08-01 ┆ 82.66 ┆ 4696 │
│ Germany ┆ 2019-01-01 ┆ 83.12 ┆ 4696 │
│ Netherlands ┆ 2016-03-01 ┆ 17.11 ┆ 784 │
│ Netherlands ┆ 2018-08-01 ┆ 17.32 ┆ 910 │
│ Netherlands ┆ 2019-01-01 ┆ 17.4 ┆ 910 │
└─────────────┴────────────┴────────────┴──────┘
shape: (6, 4)
┌─────────────┬────────────┬────────────┬──────┐
│ country ┆ date ┆ population ┆ gdp │
│ --- ┆ --- ┆ --- ┆ --- │
│ str ┆ date ┆ f64 ┆ i64 │
╞═════════════╪════════════╪════════════╪══════╡
│ Germany ┆ 2016-03-01 ┆ 82.19 ┆ 4164 │
│ Germany ┆ 2018-08-01 ┆ 82.66 ┆ 4696 │
│ Germany ┆ 2019-01-01 ┆ 83.12 ┆ 4696 │
│ Netherlands ┆ 2016-03-01 ┆ 17.11 ┆ 784 │
│ Netherlands ┆ 2018-08-01 ┆ 17.32 ┆ 910 │
│ Netherlands ┆ 2019-01-01 ┆ 17.4 ┆ 910 │
└─────────────┴────────────┴────────────┴──────┘
두 데이터프레임에 컬럼명이 동일한 경우 suffix
매개변수에 이름이 중복된 열에 추가할 접미사를 입력하여 출력할 수 있습니다.
# 'value' 열이 있는 경우
df1 = pl.DataFrame({
"date": [date(2023, 1, 1), date(2023, 2, 1), date(2023, 3, 1)],
"value": [100, 200, 300]
})
df2 = pl.DataFrame({
"date": [date(2023, 1, 15), date(2023, 2, 15), date(2023, 3, 15)],
"value": [150, 250, 350]
})
# suffix를 사용하여 중복 열 이름 처리
result = df1.join_asof(df2, on="date", suffix="_new")
print(result)
# 'value' 열이 있는 경우
df1 = pl.DataFrame({
"date": [date(2023, 1, 1), date(2023, 2, 1), date(2023, 3, 1)],
"value": [100, 200, 300]
})
df2 = pl.DataFrame({
"date": [date(2023, 1, 15), date(2023, 2, 15), date(2023, 3, 15)],
"value": [150, 250, 350]
})
# suffix를 사용하여 중복 열 이름 처리
result = df1.join_asof(df2, on="date", suffix="_new")
print(result)
shape: (3, 3)
┌────────────┬───────┬────────────┐
│ date ┆ value ┆ value_new │
│ --- ┆ --- ┆ --- │
│ date ┆ i64 ┆ i64 │
╞════════════╪═══════╪════════════╡
│ 2023-01-01 ┆ 100 ┆ null │
│ 2023-02-01 ┆ 200 ┆ 150 │
│ 2023-03-01 ┆ 300 ┆ 250 │
└────────────┴───────┴────────────┘
shape: (3, 3)
┌────────────┬───────┬────────────┐
│ date ┆ value ┆ value_new │
│ --- ┆ --- ┆ --- │
│ date ┆ i64 ┆ i64 │
╞════════════╪═══════╪════════════╡
│ 2023-01-01 ┆ 100 ┆ null │
│ 2023-02-01 ┆ 200 ┆ 150 │
│ 2023-03-01 ┆ 300 ┆ 250 │
└────────────┴───────┴────────────┘
-
tolerance : 숫자 허용 오차로 값을 설정하여 가까운 조인키가 범위 내에 있는 경우에만 조인이 수행됩니다. 데이터 타입이 "Date", "Datetime", "Duration", "Time"의 열에 대해 asof 조인을 수행하는 경우 datetime.timedelta 개체 또는 아래의 문자열 언어를 사용합니다.
-
1ns(1나노초)
-
1us(1마이크로초)
-
1ms(1밀리초)
-
1s(1초)
-
1m(1분)
-
1h(1시간)
ex) 3d12h4m25s (3일, 12시간 4분 25초)
- 1d(1역일)
- 1w(1역주)
- 1mo(1역월)
- 1q(1역분기)
- 1y(1역연도)
'역일'이란 다음 날의 해당 시간(서머타임으로 인해 24시간이 아닐 수 있음)을 의미합니다. '역주', '역월', '역분기', '역연도'도 마찬가지입니다.
df1 = pl.DataFrame({
"date": [date(2023, 1, 1), date(2023, 2, 1), date(2023, 3, 1)],
"value": [100, 200, 300]
})
df2 = pl.DataFrame({
"date": [date(2023, 1, 15), date(2023, 2, 15), date(2023, 3, 15)],
"value": [150, 250, 350]
})
# 20일 이내의 데이터 매칭
print(df1.join_asof(
df2,
on="date",
tolerance="20d", # 20일로 허용 오차 증가
suffix="_new"
))
df1 = pl.DataFrame({
"date": [date(2023, 1, 1), date(2023, 2, 1), date(2023, 3, 1)],
"value": [100, 200, 300]
})
df2 = pl.DataFrame({
"date": [date(2023, 1, 15), date(2023, 2, 15), date(2023, 3, 15)],
"value": [150, 250, 350]
})
# 20일 이내의 데이터 매칭
print(df1.join_asof(
df2,
on="date",
tolerance="20d", # 20일로 허용 오차 증가
suffix="_new"
))
shape: (3, 3)
┌────────────┬───────┬─────────────┐
│ date ┆ value ┆ value_new │
│ --- ┆ --- ┆ --- │
│ date ┆ i64 ┆ i64 │
╞════════════╪═══════╪═════════════╡
│ 2023-01-01 ┆ 100 ┆ null │
│ 2023-02-01 ┆ 200 ┆ 150 │
│ 2023-03-01 ┆ 300 ┆ 250 │
└────────────┴───────┴─────────────┘
shape: (3, 3)
┌────────────┬───────┬─────────────┐
│ date ┆ value ┆ value_new │
│ --- ┆ --- ┆ --- │
│ date ┆ i64 ┆ i64 │
╞════════════╪═══════╪═════════════╡
│ 2023-01-01 ┆ 100 ┆ null │
│ 2023-02-01 ┆ 200 ┆ 150 │
│ 2023-03-01 ┆ 300 ┆ 250 │
└────────────┴───────┴─────────────┘
대용량 데이터 셋에서 병렬 처리 활성화하는 방법에 대해 알아보도록 하겠습니다.
from datetime import date
df1 = pl.DataFrame({
"date": pl.date_range(date(2023, 1, 1), date(2023, 12, 31), "1d", eager=True), # eager=True 추가
"value": list(range(365))
})
df2 = pl.DataFrame({
"date": pl.date_range(date(2023, 1, 15), date(2023, 12, 15), "1d", eager=True), # eager=True 추가
"value": list(range(335))
})
# 병렬 처리 허용
result_allow = df1.join_asof(
df2,
on="date",
allow_parallel=True,
suffix="_new"
)
print(result_allow.head())
# 병렬 처리 강제
result_force = df1.join_asof(
df2,
on="date",
force_parallel=True,
suffix="_new"
)
print(result_force.head())
from datetime import date
df1 = pl.DataFrame({
"date": pl.date_range(date(2023, 1, 1), date(2023, 12, 31), "1d", eager=True), # eager=True 추가
"value": list(range(365))
})
df2 = pl.DataFrame({
"date": pl.date_range(date(2023, 1, 15), date(2023, 12, 15), "1d", eager=True), # eager=True 추가
"value": list(range(335))
})
# 병렬 처리 허용
result_allow = df1.join_asof(
df2,
on="date",
allow_parallel=True,
suffix="_new"
)
print(result_allow.head())
# 병렬 처리 강제
result_force = df1.join_asof(
df2,
on="date",
force_parallel=True,
suffix="_new"
)
print(result_force.head())
- allow_parallel : 두 데이터프레임을 조인할 때, 컴퓨터의 여러 코어를 사용해서 동시에(병렬로) 처리할지 여부를 설정합니다. True로 설정하면 병렬 처리를 허용합니다.
- force_parallel : 두 데이터프레임을 조인할 때, 컴퓨터의 여러 코어를 사용해서 동시에(병렬로) 처리하도록 강제합니다. 선택이 아닌 강제로 병렬 처리를 실행합니다.
shape: (5, 3)
┌────────────┬───────┬─────────────┐
│ date ┆ value ┆ value_new │
│ --- ┆ --- ┆ --- │
│ date ┆ i64 ┆ i64 │
╞════════════╪═══════╪═════════════╡
│ 2023-01-01 ┆ 0 ┆ null │
│ 2023-01-02 ┆ 1 ┆ null │
│ 2023-01-03 ┆ 2 ┆ null │
│ 2023-01-04 ┆ 3 ┆ null │
│ 2023-01-05 ┆ 4 ┆ null │
└────────────┴───────┴─────────────┘
shape: (5, 3)
┌────────────┬───────┬─────────────┐
│ date ┆ value ┆ value_new │
│ --- ┆ --- ┆ --- │
│ date ┆ i64 ┆ i64 │
╞════════════╪═══════╪═════════════╡
│ 2023-01-01 ┆ 0 ┆ null │
│ 2023-01-02 ┆ 1 ┆ null │
│ 2023-01-03 ┆ 2 ┆ null │
│ 2023-01-04 ┆ 3 ┆ null │
│ 2023-01-05 ┆ 4 ┆ null │
└────────────┴───────┴─────────────┘
shape: (5, 3)
┌────────────┬───────┬─────────────┐
│ date ┆ value ┆ value_new │
│ --- ┆ --- ┆ --- │
│ date ┆ i64 ┆ i64 │
╞════════════╪═══════╪═════════════╡
│ 2023-01-01 ┆ 0 ┆ null │
│ 2023-01-02 ┆ 1 ┆ null │
│ 2023-01-03 ┆ 2 ┆ null │
│ 2023-01-04 ┆ 3 ┆ null │
│ 2023-01-05 ┆ 4 ┆ null │
└────────────┴───────┴─────────────┘
shape: (5, 3)
┌────────────┬───────┬─────────────┐
│ date ┆ value ┆ value_new │
│ --- ┆ --- ┆ --- │
│ date ┆ i64 ┆ i64 │
╞════════════╪═══════╪═════════════╡
│ 2023-01-01 ┆ 0 ┆ null │
│ 2023-01-02 ┆ 1 ┆ null │
│ 2023-01-03 ┆ 2 ┆ null │
│ 2023-01-04 ┆ 3 ┆ null │
│ 2023-01-05 ┆ 4 ┆ null │
└────────────┴───────┴─────────────┘
df1 = pl.DataFrame({
"date": [date(2023, 1, 1), date(2023, 2, 1), date(2023, 3, 1)],
"value": [100, None, 300]
})
df2 = pl.DataFrame({
"date": [date(2023, 1, 15), date(2023, 2, 15), date(2023, 3, 15)],
"value": [150, 250, 350]
})
# coalesce를 True로 설정하여 조인 열 병합
result = df1.join_asof(
df2,
on="date",
coalesce=True,# None 값을 df2의 값으로 대체
suffix="_new"
)
print(result)
df1 = pl.DataFrame({
"date": [date(2023, 1, 1), date(2023, 2, 1), date(2023, 3, 1)],
"value": [100, None, 300]
})
df2 = pl.DataFrame({
"date": [date(2023, 1, 15), date(2023, 2, 15), date(2023, 3, 15)],
"value": [150, 250, 350]
})
# coalesce를 True로 설정하여 조인 열 병합
result = df1.join_asof(
df2,
on="date",
coalesce=True,# None 값을 df2의 값으로 대체
suffix="_new"
)
print(result)
- coalesce : 조인할 때 같은 이름의 열들을 하나로 병합하는 설정입니다. 단순 열(col)이 아닌 다른 표현식으로 조인하면 이 병합 기능이 해제됩니다.
- True: 항상 조인 열을 병합합니다.
- False: 조인 열을 병합하지 않습니다.
shape: (3, 3)
┌────────────┬───────┬─────────────┐
│ date ┆ value ┆ value_new │
│ --- ┆ --- ┆ --- │
│ date ┆ i64 ┆ i64 │
╞════════════╪═══════╪═════════════╡
│ 2023-01-01 ┆ 100 ┆ null │
│ 2023-02-01 ┆ null ┆ 150 │
│ 2023-03-01 ┆ 300 ┆ 250 │
└────────────┴───────┴─────────────┘
shape: (3, 3)
┌────────────┬───────┬─────────────┐
│ date ┆ value ┆ value_new │
│ --- ┆ --- ┆ --- │
│ date ┆ i64 ┆ i64 │
╞════════════╪═══════╪═════════════╡
│ 2023-01-01 ┆ 100 ┆ null │
│ 2023-02-01 ┆ null ┆ 150 │
│ 2023-03-01 ┆ 300 ┆ 250 │
└────────────┴───────┴─────────────┘
1.1.8 merge_sorted
merge_sorted
메서드는 정렬된 두 개의 데이터프레임을 가져와 정렬된 조인 키로 병합합니다. 출력 결과도 정렬되어 나옵니다.
이때, 두 데이터프레임의 스키마는 동일해야하며, 각각의 데이터 프레임은 정렬할 키를 기준으로 정렬되어 있어야 합니다.
df0 = pl.DataFrame(
{"name": ["steve", "elise", "bob"], "age": [42, 44, 18]}
).sort("age")
df1 = pl.DataFrame(
{"name": ["anna", "megan", "steve", "thomas"], "age": [21, 33, 42, 20]}
).sort("age")
# 데이터프레임1.merge_sorted(데이터프레임2, key="정렬할 컬럼명")
print(df0.merge_sorted(df1, key="age"))
df0 = pl.DataFrame(
{"name": ["steve", "elise", "bob"], "age": [42, 44, 18]}
).sort("age")
df1 = pl.DataFrame(
{"name": ["anna", "megan", "steve", "thomas"], "age": [21, 33, 42, 20]}
).sort("age")
# 데이터프레임1.merge_sorted(데이터프레임2, key="정렬할 컬럼명")
print(df0.merge_sorted(df1, key="age"))
shape: (7, 2)
┌────────┬─────┐
│ name ┆ age │
│ --- ┆ --- │
│ str ┆ i64 │
╞════════╪═════╡
│ bob ┆ 18 │
│ thomas ┆ 20 │
│ anna ┆ 21 │
│ megan ┆ 33 │
│ steve ┆ 42 │
│ steve ┆ 42 │
│ elise ┆ 44 │
└────────┴─────┘
shape: (7, 2)
┌────────┬─────┐
│ name ┆ age │
│ --- ┆ --- │
│ str ┆ i64 │
╞════════╪═════╡
│ bob ┆ 18 │
│ thomas ┆ 20 │
│ anna ┆ 21 │
│ megan ┆ 33 │
│ steve ┆ 42 │
│ steve ┆ 42 │
│ elise ┆ 44 │
└────────┴─────┘
1.2 데이터 분해
1.2.1 explode
explode 메서드는 지정된 열을 분해하여 데이터 프레임을 긴 형식으로 분해합니다. 분해되는 기본 열은 리스트 또는 배열 데이터 타입이어야 합니다.
df = pl.DataFrame({
"letters": ["a", "a", "b", "c"],
"numbers": [[1], [2, 3], [4, 5], [6, 7, 8]],
})
print(df.explode("numbers"))
df = pl.DataFrame({
"letters": ["a", "a", "b", "c"],
"numbers": [[1], [2, 3], [4, 5], [6, 7, 8]],
})
print(df.explode("numbers"))
shape: (8, 2)
┌─────────┬─────────┐
│ letters ┆ numbers │
│ --- ┆ --- │
│ str ┆ i64 │
╞═════════╪═════════╡
│ a ┆ 1 │
│ a ┆ 2 │
│ a ┆ 3 │
│ b ┆ 4 │
│ b ┆ 5 │
│ c ┆ 6 │
│ c ┆ 7 │
│ c ┆ 8 │
└─────────┴─────────┘
shape: (8, 2)
┌─────────┬─────────┐
│ letters ┆ numbers │
│ --- ┆ --- │
│ str ┆ i64 │
╞═════════╪═════════╡
│ a ┆ 1 │
│ a ┆ 2 │
│ a ┆ 3 │
│ b ┆ 4 │
│ b ┆ 5 │
│ c ┆ 6 │
│ c ┆ 7 │
│ c ┆ 8 │
└─────────┴─────────┘
1.2.2 unnest
unnest 메서드는 각 필드에 대해 구조체 열을 별도의 열로 분해합니다. 새 열은 기존 열의 위치에 있는 데이터프레임에 옆에 추가됩니다.
df = pl.DataFrame({
"before": ["foo", "bar"],
"t_a": [1, 2],
"t_b": ["a", "b"],
"t_c": [True, None],
"t_d": [[1, 2], [3]],
"after": ["baz", "womp"],
}).select("before", pl.struct(pl.col("^t_.$")).alias("t_struct"), "after")
print(df.unnest("t_struct"))
df = pl.DataFrame({
"before": ["foo", "bar"],
"t_a": [1, 2],
"t_b": ["a", "b"],
"t_c": [True, None],
"t_d": [[1, 2], [3]],
"after": ["baz", "womp"],
}).select("before", pl.struct(pl.col("^t_.$")).alias("t_struct"), "after")
print(df.unnest("t_struct"))
pl.struct()
는 이 선택된 열들을 하나의 구조체로 묶습니다.^t_.$
: "t_"로 시작하고 그 뒤에 한 문자가 오는 모든 열을 선택합니다.
shape: (2, 6)
┌────────┬─────┬─────┬──────┬───────────┬───────┐
│ before ┆ t_a ┆ t_b ┆ t_c ┆ t_d ┆ after │
│ --- ┆ --- ┆ --- ┆ --- ┆ --- ┆ --- │
│ str ┆ i64 ┆ str ┆ bool ┆ list[i64] ┆ str │
╞════════╪═════╪═════╪══════╪═══════════╪═══════╡
│ foo ┆ 1 ┆ a ┆ true ┆ [1, 2] ┆ baz │
│ bar ┆ 2 ┆ b ┆ null ┆ [3] ┆ womp │
└────────┴─────┴─────┴──────┴───────────┴───────┘
shape: (2, 6)
┌────────┬─────┬─────┬──────┬───────────┬───────┐
│ before ┆ t_a ┆ t_b ┆ t_c ┆ t_d ┆ after │
│ --- ┆ --- ┆ --- ┆ --- ┆ --- ┆ --- │
│ str ┆ i64 ┆ str ┆ bool ┆ list[i64] ┆ str │
╞════════╪═════╪═════╪══════╪═══════════╪═══════╡
│ foo ┆ 1 ┆ a ┆ true ┆ [1, 2] ┆ baz │
│ bar ┆ 2 ┆ b ┆ null ┆ [3] ┆ womp │
└────────┴─────┴─────┴──────┴───────────┴───────┘
2. 데이터 그룹화
2.1 group_by
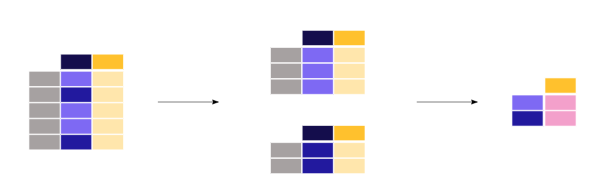
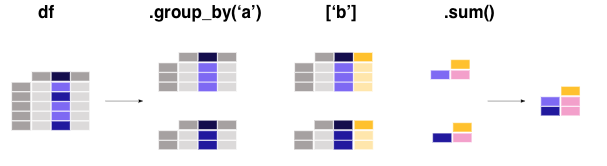
데이터프레임을 생성하고 a열을 기준으로 그룹화 연산을 수행해보도록 하겠습니다.
df = pl.DataFrame(
{
"a": ["a", "b", "a", "b", "c"],
"b": [1, 2, 1, 3, 3],
"c": [5, 4, 3, 2, 1],
}
)
# df.group_by("컬럼명").agg(연산)
print(df.group_by("a").agg(pl.col("b").sum()))
print(df.group_by("a").agg([pl.col("b").sum().alias('b_sum'), pl.col("b").max().alias('b_max')]))
print(df.group_by("a").agg(pl.col("b").sum(), pl.col("c").max()))
df = pl.DataFrame(
{
"a": ["a", "b", "a", "b", "c"],
"b": [1, 2, 1, 3, 3],
"c": [5, 4, 3, 2, 1],
}
)
# df.group_by("컬럼명").agg(연산)
print(df.group_by("a").agg(pl.col("b").sum()))
print(df.group_by("a").agg([pl.col("b").sum().alias('b_sum'), pl.col("b").max().alias('b_max')]))
print(df.group_by("a").agg(pl.col("b").sum(), pl.col("c").max()))
- agg : 한 번에 여러 함수를 동시 입력 및 출력이 가능한 함수입니다.
agg(pl.col("b").sum())
: 그룹별 b열 합계agg([pl.col("b").sum().alias('b_sum'), pl.col("b").max().alias('b_max')])
: 그룹별 b열 합계, b열 최대값- 연산 결과의 컬럼명은 수행한 열의 컬럼명과 같기 때문에 동일한 컬럼명이 여러개가 있으므로 에러가 뜨게 됩니다. 그러므로 동일한 열의 연산을 여러번 수행할 경우
.alias()
를 사용하여 별칭을 지어주셔야 합니다.
- 연산 결과의 컬럼명은 수행한 열의 컬럼명과 같기 때문에 동일한 컬럼명이 여러개가 있으므로 에러가 뜨게 됩니다. 그러므로 동일한 열의 연산을 여러번 수행할 경우
agg(pl.col("b").sum(), pl.col("c").max())
: 그룹별 b열 합계, c열 최대값
shape: (3, 2)
┌─────┬─────┐
│ a ┆ b │
│ --- ┆ --- │
│ str ┆ i64 │
╞═════╪═════╡
│ a ┆ 2 │
│ b ┆ 5 │
│ c ┆ 3 │
└─────┴─────┘
shape: (3, 3)
┌─────┬───────┬───────┐
│ a ┆ b_sum ┆ b_max │
│ --- ┆ --- ┆ --- │
│ str ┆ i64 ┆ i64 │
╞═════╪═══════╪═══════╡
│ a ┆ 2 ┆ 1 │
│ c ┆ 3 ┆ 3 │
│ b ┆ 5 ┆ 3 │
└─────┴───────┴───────┘
shape: (3, 3)
┌─────┬─────┬─────┐
│ a ┆ b ┆ c │
│ --- ┆ --- ┆ --- │
│ str ┆ i64 ┆ i64 │
╞═════╪═════╪═════╡
│ c ┆ 3 ┆ 1 │
│ a ┆ 2 ┆ 5 │
│ b ┆ 5 ┆ 4 │
└─────┴─────┴─────┘
shape: (3, 2)
┌─────┬─────┐
│ a ┆ b │
│ --- ┆ --- │
│ str ┆ i64 │
╞═════╪═════╡
│ a ┆ 2 │
│ b ┆ 5 │
│ c ┆ 3 │
└─────┴─────┘
shape: (3, 3)
┌─────┬───────┬───────┐
│ a ┆ b_sum ┆ b_max │
│ --- ┆ --- ┆ --- │
│ str ┆ i64 ┆ i64 │
╞═════╪═══════╪═══════╡
│ a ┆ 2 ┆ 1 │
│ c ┆ 3 ┆ 3 │
│ b ┆ 5 ┆ 3 │
└─────┴───────┴───────┘
shape: (3, 3)
┌─────┬─────┬─────┐
│ a ┆ b ┆ c │
│ --- ┆ --- ┆ --- │
│ str ┆ i64 ┆ i64 │
╞═════╪═════╪═════╡
│ c ┆ 3 ┆ 1 │
│ a ┆ 2 ┆ 5 │
│ b ┆ 5 ┆ 4 │
└─────┴─────┴─────┘
위에 코드를 실행 결과를 보면, 그룹의 순서가 데이터프레임 생성 순서와 일치하지 않는 것을 볼 수 있습니다. 만약, 일치하도록 유지하려면 maintain_order=True
로 설정합니다. True로 설정하면 스트리밍 엔진에서 실행될 가능성이 차단되며, False로 설정했을 때보다 느리게 동작합니다. 이때, 각 그룹 내에서의 행 순서는 기존 순서와 일치합니다.
print(df.group_by("a", maintain_order=True).agg(pl.col("c")))
print(df.group_by("a", maintain_order=True).agg(pl.col("c")))
shape: (3, 2)
┌─────┬───────────┐
│ a ┆ c │
│ --- ┆ --- │
│ str ┆ list[i64] │
╞═════╪═══════════╡
│ a ┆ [5, 3] │
│ b ┆ [4, 2] │
│ c ┆ [1] │
└─────┴───────────┘
shape: (3, 2)
┌─────┬───────────┐
│ a ┆ c │
│ --- ┆ --- │
│ str ┆ list[i64] │
╞═════╪═══════════╡
│ a ┆ [5, 3] │
│ b ┆ [4, 2] │
│ c ┆ [1] │
└─────┴───────────┘
이번에는 여러열을 기준으로 그룹화를 진행해보도록 하겠습니다. 이때, 그룹화 할 열들을 순차적으로 작성하거나 리스트로 전달하여 그룹화를 수행할 수 있습니다. a열과 b열을 기준으로 그룹화하고 그룹별 c열의 최대값을 구해보도록 하겠습니다.
print(df.group_by(["a", "b"]).agg(pl.max("c")))
print(df.group_by(["a", "b"]).agg(pl.max("c")))
shape: (4, 3)
┌─────┬─────┬─────┐
│ a ┆ b ┆ c │
│ --- ┆ --- ┆ --- │
│ str ┆ i64 ┆ i64 │
╞═════╪═════╪═════╡
│ a ┆ 1 ┆ 5 │
│ b ┆ 2 ┆ 4 │
│ b ┆ 3 ┆ 2 │
│ c ┆ 3 ┆ 1 │
└─────┴─────┴─────┘
shape: (4, 3)
┌─────┬─────┬─────┐
│ a ┆ b ┆ c │
│ --- ┆ --- ┆ --- │
│ str ┆ i64 ┆ i64 │
╞═════╪═════╪═════╡
│ a ┆ 1 ┆ 5 │
│ b ┆ 2 ┆ 4 │
│ b ┆ 3 ┆ 2 │
│ c ┆ 3 ┆ 1 │
└─────┴─────┴─────┘
이때, 그룹화 한 컬럼명은 사용된 컬럼으로 집계에 사용할 수 없습니다.
print(df.group_by(["a", "b"]).agg(pl.max("a")))
print(df.group_by(["a", "b"]).agg(pl.max("a")))
DuplicateError: column with name 'a' has more than one occurrence
Resolved plan until failure:
---> FAILED HERE RESOLVING 'group by'
DF ("a", "b" "c"]. PROJECT */3 COLUMNS; SELECTION: None
DuplicateError: column with name 'a' has more than one occurrence
Resolved plan until failure:
---> FAILED HERE RESOLVING 'group by'
DF ("a", "b" "c"]. PROJECT */3 COLUMNS; SELECTION: None
이번에는 표현식을 사용하여 a열을 기준으로 먼저 그룹화하고 b열을 2로 나눈 값의 몫으로 그룹화를 수행하여 그룹별 c열의 평균 값을 구해보도록 하겠습니다.
print(df.group_by("a", pl.col("b") // 2).agg(pl.col("c").mean()))
print(df.group_by("a", pl.col("b") // 2).agg(pl.col("c").mean()))
shape: (3, 3)
┌─────┬─────┬─────┐
│ a ┆ b ┆ c │
│ --- ┆ --- ┆ --- │
│ str ┆ i64 ┆ f64 │
╞═════╪═════╪═════╡
│ b ┆ 1 ┆ 3.0 │
│ c ┆ 1 ┆ 1.0 │
│ a ┆ 0 ┆ 4.0 │
└─────┴─────┴─────┘
shape: (3, 3)
┌─────┬─────┬─────┐
│ a ┆ b ┆ c │
│ --- ┆ --- ┆ --- │
│ str ┆ i64 ┆ f64 │
╞═════╪═════╪═════╡
│ b ┆ 1 ┆ 3.0 │
│ c ┆ 1 ┆ 1.0 │
│ a ┆ 0 ┆ 4.0 │
└─────┴─────┴─────┘
group_by를 수행한 후 반환된 GroupBy 객체는 반복 가능합니다.
print(df.group_by("a"))
print(df.group_by("a"))
<polars.dataframe.group by.GroupBy object at 0x7adce6c013f0>
<polars.dataframe.group by.GroupBy object at 0x7adce6c013f0>
GroupBy 객체를 순회하여 각 그룹명과 데이터를 반환해보도록 하겠습니다. 출력 결과를 보시면 그룹 이름과 그룹별 데이터가 출력된 것을 보실 수 있습니다.
for name, data in df.group_by("a"):
print(name)
print(data)
for name, data in df.group_by("a"):
print(name)
print(data)
a
shape: (2, 3)
┌─────┬─────┬─────┐
│ a ┆ b ┆ c │
│ --- ┆ --- ┆ --- │
│ str ┆ i64 ┆ i64 │
╞═════╪═════╪═════╡
│ a ┆ 1 ┆ 5 │
│ a ┆ 1 ┆ 3 │
└─────┴─────┴─────┘
b
shape: (2, 3)
┌─────┬─────┬─────┐
│ a ┆ b ┆ c │
│ --- ┆ --- ┆ --- │
│ str ┆ i64 ┆ i64 │
╞═════╪═════╪═════╡
│ b ┆ 2 ┆ 4 │
│ b ┆ 3 ┆ 2 │
└─────┴─────┴─────┘
c
shape: (1, 3)
┌─────┬─────┬─────┐
│ a ┆ b ┆ c │
│ --- ┆ --- ┆ --- │
│ str ┆ i64 ┆ i64 │
╞═════╪═════╪═════╡
│ c ┆ 3 ┆ 1 │
└─────┴─────┴─────┘
a
shape: (2, 3)
┌─────┬─────┬─────┐
│ a ┆ b ┆ c │
│ --- ┆ --- ┆ --- │
│ str ┆ i64 ┆ i64 │
╞═════╪═════╪═════╡
│ a ┆ 1 ┆ 5 │
│ a ┆ 1 ┆ 3 │
└─────┴─────┴─────┘
b
shape: (2, 3)
┌─────┬─────┬─────┐
│ a ┆ b ┆ c │
│ --- ┆ --- ┆ --- │
│ str ┆ i64 ┆ i64 │
╞═════╪═════╪═════╡
│ b ┆ 2 ┆ 4 │
│ b ┆ 3 ┆ 2 │
└─────┴─────┴─────┘
c
shape: (1, 3)
┌─────┬─────┬─────┐
│ a ┆ b ┆ c │
│ --- ┆ --- ┆ --- │
│ str ┆ i64 ┆ i64 │
╞═════╪═════╪═════╡
│ c ┆ 3 ┆ 1 │
└─────┴─────┴─────┘
2.2 group_by_dynamic
group_by_dynamic 메서드는 시간 값(또는 Int32, Int64 유형의 인덱스 값)을 기준으로 그룹화합니다. every, period, offset 매개변수의 인수로 아래와 같은 문자열로 그룹화 할 기간을 설정할 수 있습니다.
- 1ns(1나노초)
- 1us(1마이크로초)
- 1ms(1밀리초)
- 1s(1초)
- 1m(1분)
- 1h(1시간)
- 1d(1역일)
- 1w(1역주)
- 1mo(1역월)
- 1q(1역분기)
- 1y(1역연도)
ex) 3d12h4m25s (3일, 12시간 4분 25초)
'역일'이란 다음 날의 해당 시간(서머타임으로 인해 24시간이 아닐 수 있음)을 의미합니다. '역주', '역월', '역분기', '역연도'도 마찬가지입니다.
실습에 앞서, 시계열 데이터를 생성해보도록 하겠습니다.
from datetime import datetime
df = pl.DataFrame(
{
"time": pl.datetime_range(
start=datetime(2021, 12, 16),
end=datetime(2021, 12, 16, 3),
interval="30m",
eager=True,
),
"n": range(7),
}
)
print(df)
from datetime import datetime
df = pl.DataFrame(
{
"time": pl.datetime_range(
start=datetime(2021, 12, 16),
end=datetime(2021, 12, 16, 3),
interval="30m",
eager=True,
),
"n": range(7),
}
)
print(df)
- datetime_range(시작날짜, 종료날짜, interval, eager) : 시작 날짜/시간부터 종료 날짜/시간까지 일정한 간격으로 날짜/시간 시퀀스를 생성합니다.
- interval : 일정 간격으로 데이터 생성
eager=True
: 결과를 즉시 계산하여 메모리에 저장
shape: (7, 2)
┌─────────────────────┬─────┐
│ time ┆ n │
│ --- ┆ --- │
│ datetime[μs] ┆ i64 │
╞═════════════════════╪═════╡
│ 2021-12-16 00:00:00 ┆ 0 │
│ 2021-12-16 00:30:00 ┆ 1 │
│ 2021-12-16 01:00:00 ┆ 2 │
│ 2021-12-16 01:30:00 ┆ 3 │
│ 2021-12-16 02:00:00 ┆ 4 │
│ 2021-12-16 02:30:00 ┆ 5 │
│ 2021-12-16 03:00:00 ┆ 6 │
└─────────────────────┴─────┘
shape: (7, 2)
┌─────────────────────┬─────┐
│ time ┆ n │
│ --- ┆ --- │
│ datetime[μs] ┆ i64 │
╞═════════════════════╪═════╡
│ 2021-12-16 00:00:00 ┆ 0 │
│ 2021-12-16 00:30:00 ┆ 1 │
│ 2021-12-16 01:00:00 ┆ 2 │
│ 2021-12-16 01:30:00 ┆ 3 │
│ 2021-12-16 02:00:00 ┆ 4 │
│ 2021-12-16 02:30:00 ┆ 5 │
│ 2021-12-16 03:00:00 ┆ 6 │
└─────────────────────┴─────┘
2021-12-16 00:00:00(start)부터 1시간 단위로 그룹화를 해보도록 하겠습니다. 출력 결과를 보시면 time 컬럼을 기준으로 1시간 단위로 그룹화 된 것을 보실 수 있습니다.
# df.group_by_dynamic("컬럼명", every="시작점 이동 간격", closed="닫을 방향").agg(pl.col("n"))
print(df.group_by_dynamic("time", every="1h", closed="right").agg(pl.col("n")))
# df.group_by_dynamic("컬럼명", every="시작점 이동 간격", closed="닫을 방향").agg(pl.col("n"))
print(df.group_by_dynamic("time", every="1h", closed="right").agg(pl.col("n")))
shape: (4, 2)
┌─────────────────────┬───────────┐
│ time ┆ n │
│ --- ┆ --- │
│ datetime[μs] ┆ list[i64] │
╞═════════════════════╪═══════════╡
│ 2021-12-15 23:00:00 ┆ [0] │
│ 2021-12-16 00:00:00 ┆ [1, 2] │
│ 2021-12-16 01:00:00 ┆ [3, 4] │
│ 2021-12-16 02:00:00 ┆ [5, 6] │
└─────────────────────┴───────────┘
shape: (4, 2)
┌─────────────────────┬───────────┐
│ time ┆ n │
│ --- ┆ --- │
│ datetime[μs] ┆ list[i64] │
╞═════════════════════╪═══════════╡
│ 2021-12-15 23:00:00 ┆ [0] │
│ 2021-12-16 00:00:00 ┆ [1, 2] │
│ 2021-12-16 01:00:00 ┆ [3, 4] │
│ 2021-12-16 02:00:00 ┆ [5, 6] │
└─────────────────────┴───────────┘
-
컬럼명 : 그룹화에 사용되는 열로 데이터 타입은 보통 날짜/시간 데이터 타입입니다. 이때, 그룹화 할 열은 오름차순으로 정렬해야 하며, 오름차순으로 정렬하지 않을 경우 에러가 납니다. new_row 데이터프레임 마지막 행에 df 데이터프레임을 결합하여 정렬하지 않았을 때, 어떤 오류가 뜨는지 확인해보도록 하겠습니다.
new_row = pl.DataFrame( { "time": [datetime(2021, 12, 17, 2, 0, 0), datetime(2021, 12, 18, 2, 0, 0), datetime(2021, 12, 19, 2, 0, 0), datetime(2021, 12, 20, 2, 0, 0)], "n": [4,6,3,2] } ) new_df = new_row.vstack(df) print(new_df)
new_row = pl.DataFrame( { "time": [datetime(2021, 12, 17, 2, 0, 0), datetime(2021, 12, 18, 2, 0, 0), datetime(2021, 12, 19, 2, 0, 0), datetime(2021, 12, 20, 2, 0, 0)], "n": [4,6,3,2] } ) new_df = new_row.vstack(df) print(new_df)
shape: (11, 2) ┌─────────────────────┬─────┐ │ time ┆ n │ │ --- ┆ --- │ │ datetime[μs] ┆ i64 │ ╞═════════════════════╪═════╡ │ 2021-12-17 02:00:00 ┆ 4 │ │ 2021-12-18 02:00:00 ┆ 6 │ │ 2021-12-19 02:00:00 ┆ 3 │ │ 2021-12-20 02:00:00 ┆ 2 │ │ 2021-12-16 00:00:00 ┆ 0 │ │ … ┆ … │ │ 2021-12-16 01:00:00 ┆ 2 │ │ 2021-12-16 01:30:00 ┆ 3 │ │ 2021-12-16 02:00:00 ┆ 4 │ │ 2021-12-16 02:30:00 ┆ 5 │ │ 2021-12-16 03:00:00 ┆ 6 │ └─────────────────────┴─────┘
shape: (11, 2) ┌─────────────────────┬─────┐ │ time ┆ n │ │ --- ┆ --- │ │ datetime[μs] ┆ i64 │ ╞═════════════════════╪═════╡ │ 2021-12-17 02:00:00 ┆ 4 │ │ 2021-12-18 02:00:00 ┆ 6 │ │ 2021-12-19 02:00:00 ┆ 3 │ │ 2021-12-20 02:00:00 ┆ 2 │ │ 2021-12-16 00:00:00 ┆ 0 │ │ … ┆ … │ │ 2021-12-16 01:00:00 ┆ 2 │ │ 2021-12-16 01:30:00 ┆ 3 │ │ 2021-12-16 02:00:00 ┆ 4 │ │ 2021-12-16 02:30:00 ┆ 5 │ │ 2021-12-16 03:00:00 ┆ 6 │ └─────────────────────┴─────┘
출력 결과를 보시면 그룹화할 열이 정렬되어 있지 않았기 때문에 에러가 뜬 것을 보실 수 있습니다.
print(new_df.group_by_dynamic("time", every="1h", closed="right").agg(pl.col("n")))
print(new_df.group_by_dynamic("time", every="1h", closed="right").agg(pl.col("n")))
InvalidOperationError: argument in operation 'group_by_dynamic' is not sorted, please sort the 'expr/series/column' first
InvalidOperationError: argument in operation 'group_by_dynamic' is not sorted, please sort the 'expr/series/column' first
-
every : 시작 경계값이 얼마나 이동하는지를 결정하므로 설정된 시간 간격별로 그룹화된 행이 다시 할당됩니다.
every, period, offset 를 설정하면 시간이 계산되고 출력 결과에 행이 할당됩니다.
- [start, start + period] - [start + every, start + every + period] - [start + 2*every, start + 2*every + period] - … 여기서 start는 start_by, offset, every 매개변수에 따라 가장 빠른 시간으로 결정됩니다.
- [start, start + period] - [start + every, start + every + period] - [start + 2*every, start + 2*every + period] - … 여기서 start는 start_by, offset, every 매개변수에 따라 가장 빠른 시간으로 결정됩니다.
-
closed : 시간 간격을 어느 쪽을 닫을지 포함 관계를 정의합니다.
- right : 시작 값은
df[0,'time']
에서 -1시간 된 값이며, 종료 값은df[-1,'time']
입니다. 집계할 때는 시작 경계값 < time ≤ 종료 경계값이 됩니다. 그렇기 때문에 첫 group_by_dynamic 메서드 출력 결과에서 2021-12-15 23:00:00 값이 들어가게 됩니다. - left(기본값) : 시작 값은
df[0,'time']
이며, 종료 값은df[-1,'time']
에서 +1시간 된 값 입니다. 집계할 때는 시작 경계값 ≤ time < 종료 경계값이 됩니다.print(df.group_by_dynamic("time", every="1h", closed="left").agg(pl.col("n")))
print(df.group_by_dynamic("time", every="1h", closed="left").agg(pl.col("n")))
shape: (4, 2) ┌─────────────────────┬───────────┐ │ time ┆ n │ │ --- ┆ --- │ │ datetime[μs] ┆ list[i64] │ ╞═════════════════════╪═══════════╡ │ 2021-12-16 00:00:00 ┆ [0, 1] │ │ 2021-12-16 01:00:00 ┆ [2, 3] │ │ 2021-12-16 02:00:00 ┆ [4, 5] │ │ 2021-12-16 03:00:00 ┆ [6] │ └─────────────────────┴───────────┘
shape: (4, 2) ┌─────────────────────┬───────────┐ │ time ┆ n │ │ --- ┆ --- │ │ datetime[μs] ┆ list[i64] │ ╞═════════════════════╪═══════════╡ │ 2021-12-16 00:00:00 ┆ [0, 1] │ │ 2021-12-16 01:00:00 ┆ [2, 3] │ │ 2021-12-16 02:00:00 ┆ [4, 5] │ │ 2021-12-16 03:00:00 ┆ [6] │ └─────────────────────┴───────────┘
- both : 시작 값은
df[0,'time']
에서 -1시간 된 값이며, 종료 값은df[-1,'time']
에서 +1시간 된 값입니다. 집계할 때는 시작 경계값 ≤ time ≤ 종료 경계값이 됩니다. 이처럼 time 값은 두 경계에 모두 속하기 때문에 하나의 행이 여러 그룹의 멤버가 될 수 있습니다.print(df.group_by_dynamic("time", every="1h", closed="both").agg(pl.col("n")))
print(df.group_by_dynamic("time", every="1h", closed="both").agg(pl.col("n")))
shape: (5, 2) ┌─────────────────────┬───────────┐ │ time ┆ n │ │ --- ┆ --- │ │ datetime[μs] ┆ list[i64] │ ╞═════════════════════╪═══════════╡ │ 2021-12-15 23:00:00 ┆ [0] │ │ 2021-12-16 00:00:00 ┆ [0, 1, 2] │ │ 2021-12-16 01:00:00 ┆ [2, 3, 4] │ │ 2021-12-16 02:00:00 ┆ [4, 5, 6] │ │ 2021-12-16 03:00:00 ┆ [6] │ └─────────────────────┴───────────┘
shape: (5, 2) ┌─────────────────────┬───────────┐ │ time ┆ n │ │ --- ┆ --- │ │ datetime[μs] ┆ list[i64] │ ╞═════════════════════╪═══════════╡ │ 2021-12-15 23:00:00 ┆ [0] │ │ 2021-12-16 00:00:00 ┆ [0, 1, 2] │ │ 2021-12-16 01:00:00 ┆ [2, 3, 4] │ │ 2021-12-16 02:00:00 ┆ [4, 5, 6] │ │ 2021-12-16 03:00:00 ┆ [6] │ └─────────────────────┴───────────┘
- none : 시작 값은
df[0,'time']
값이며, 종료 값은df[-1,'time']
값 입니다. 집계할 때는 시작 경계값 < time < 종료 경계값으로, time 값은 두 경계에 모두 포함하지 않으며, 중간에 값이 있는 경우만 출력합니다.print(df.group_by_dynamic("time", every="1h", closed="none").agg(pl.col("n")))
print(df.group_by_dynamic("time", every="1h", closed="none").agg(pl.col("n")))
shape: (3, 2) ┌─────────────────────┬───────────┐ │ time ┆ n │ │ --- ┆ --- │ │ datetime[μs] ┆ list[i64] │ ╞═════════════════════╪═══════════╡ │ 2021-12-16 00:00:00 ┆ [1] │ │ 2021-12-16 01:00:00 ┆ [3] │ │ 2021-12-16 02:00:00 ┆ [5] │ └─────────────────────┴───────────┘
shape: (3, 2) ┌─────────────────────┬───────────┐ │ time ┆ n │ │ --- ┆ --- │ │ datetime[μs] ┆ list[i64] │ ╞═════════════════════╪═══════════╡ │ 2021-12-16 00:00:00 ┆ [1] │ │ 2021-12-16 01:00:00 ┆ [3] │ │ 2021-12-16 02:00:00 ┆ [5] │ └─────────────────────┴───────────┘
- right : 시작 값은
-
offset : 그룹화 할 범위를 넓힐 수 있습니다. 기본값은 0이며, start_by가 'datapoint'인 경우 적용되지는 않습니다. time 컬럼을 기준으로 1시간 단위로 그룹화 하고 시작 경계값과 종료 경계값을 10분씩 이동시켜 n을 그룹화해보도록 하겠습니다.
print(df.group_by_dynamic("time", every="1h", include_boundaries=True, offset="10m").agg(pl.col("n")))
print(df.group_by_dynamic("time", every="1h", include_boundaries=True, offset="10m").agg(pl.col("n")))
include_boundaries=True
로 설정할 경우 범위의 시작과 종료를 출력 결과에 추가하여 그룹화 범위를 쉽게 파악할 수 있지만, 병렬 처리가 더 어려워지므로 성능에 영향을 미칩니다.
shape: (4, 4) ┌─────────────────────┬─────────────────────┬─────────────────────┬───────────┐ │ _lower_boundary ┆ _upper_boundary ┆ time ┆ n │ │ --- ┆ --- ┆ --- ┆ --- │ │ datetime[μs] ┆ datetime[μs] ┆ datetime[μs] ┆ list[i64] │ ╞═════════════════════╪═════════════════════╪═════════════════════╪═══════════╡ │ 2021-12-15 23:10:00 ┆ 2021-12-16 00:10:00 ┆ 2021-12-15 23:10:00 ┆ [0] │ │ 2021-12-16 00:10:00 ┆ 2021-12-16 01:10:00 ┆ 2021-12-16 00:10:00 ┆ [1, 2] │ │ 2021-12-16 01:10:00 ┆ 2021-12-16 02:10:00 ┆ 2021-12-16 01:10:00 ┆ [3, 4] │ │ 2021-12-16 02:10:00 ┆ 2021-12-16 03:10:00 ┆ 2021-12-16 02:10:00 ┆ [5, 6] │ └─────────────────────┴─────────────────────┴─────────────────────┴───────────┘
shape: (4, 4) ┌─────────────────────┬─────────────────────┬─────────────────────┬───────────┐ │ _lower_boundary ┆ _upper_boundary ┆ time ┆ n │ │ --- ┆ --- ┆ --- ┆ --- │ │ datetime[μs] ┆ datetime[μs] ┆ datetime[μs] ┆ list[i64] │ ╞═════════════════════╪═════════════════════╪═════════════════════╪═══════════╡ │ 2021-12-15 23:10:00 ┆ 2021-12-16 00:10:00 ┆ 2021-12-15 23:10:00 ┆ [0] │ │ 2021-12-16 00:10:00 ┆ 2021-12-16 01:10:00 ┆ 2021-12-16 00:10:00 ┆ [1, 2] │ │ 2021-12-16 01:10:00 ┆ 2021-12-16 02:10:00 ┆ 2021-12-16 01:10:00 ┆ [3, 4] │ │ 2021-12-16 02:10:00 ┆ 2021-12-16 03:10:00 ┆ 2021-12-16 02:10:00 ┆ [5, 6] │ └─────────────────────┴─────────────────────┴─────────────────────┴───────────┘
-
label : 출력 결과에 사용할 라벨 정의할 수 있습니다.
- left : 시작 경계값을 time 값으로 설정합니다.
print(df.group_by_dynamic( "time", every="1h", include_boundaries=True, closed="right", label='left' ).agg(pl.col("n")))
print(df.group_by_dynamic( "time", every="1h", include_boundaries=True, closed="right", label='left' ).agg(pl.col("n")))
shape: (4, 4) ┌─────────────────────┬─────────────────────┬─────────────────────┬───────────┐ │ _lower_boundary ┆ _upper_boundary ┆ time ┆ n │ │ --- ┆ --- ┆ --- ┆ --- │ │ datetime[μs] ┆ datetime[μs] ┆ datetime[μs] ┆ list[i64] │ ╞═════════════════════╪═════════════════════╪═════════════════════╪═══════════╡ │ 2021-12-15 23:00:00 ┆ 2021-12-16 00:00:00 ┆ 2021-12-15 23:00:00 ┆ [0] │ │ 2021-12-16 00:00:00 ┆ 2021-12-16 01:00:00 ┆ 2021-12-16 00:00:00 ┆ [1, 2] │ │ 2021-12-16 01:00:00 ┆ 2021-12-16 02:00:00 ┆ 2021-12-16 01:00:00 ┆ [3, 4] │ │ 2021-12-16 02:00:00 ┆ 2021-12-16 03:00:00 ┆ 2021-12-16 02:00:00 ┆ [5, 6] │ └─────────────────────┴─────────────────────┴─────────────────────┴───────────┘
shape: (4, 4) ┌─────────────────────┬─────────────────────┬─────────────────────┬───────────┐ │ _lower_boundary ┆ _upper_boundary ┆ time ┆ n │ │ --- ┆ --- ┆ --- ┆ --- │ │ datetime[μs] ┆ datetime[μs] ┆ datetime[μs] ┆ list[i64] │ ╞═════════════════════╪═════════════════════╪═════════════════════╪═══════════╡ │ 2021-12-15 23:00:00 ┆ 2021-12-16 00:00:00 ┆ 2021-12-15 23:00:00 ┆ [0] │ │ 2021-12-16 00:00:00 ┆ 2021-12-16 01:00:00 ┆ 2021-12-16 00:00:00 ┆ [1, 2] │ │ 2021-12-16 01:00:00 ┆ 2021-12-16 02:00:00 ┆ 2021-12-16 01:00:00 ┆ [3, 4] │ │ 2021-12-16 02:00:00 ┆ 2021-12-16 03:00:00 ┆ 2021-12-16 02:00:00 ┆ [5, 6] │ └─────────────────────┴─────────────────────┴─────────────────────┴───────────┘
- right : 종료 경계값을 time 값으로 설정합니다.
print(df.group_by_dynamic( "time", every="1h", include_boundaries=True, closed="right", label='right' ).agg(pl.col("n")))
print(df.group_by_dynamic( "time", every="1h", include_boundaries=True, closed="right", label='right' ).agg(pl.col("n")))
shape: (4, 4) ┌─────────────────────┬─────────────────────┬─────────────────────┬───────────┐ │ _lower_boundary ┆ _upper_boundary ┆ time ┆ n │ │ --- ┆ --- ┆ --- ┆ --- │ │ datetime[μs] ┆ datetime[μs] ┆ datetime[μs] ┆ list[i64] │ ╞═════════════════════╪═════════════════════╪═════════════════════╪═══════════╡ │ 2021-12-15 23:00:00 ┆ 2021-12-16 00:00:00 ┆ 2021-12-16 00:00:00 ┆ [0] │ │ 2021-12-16 00:00:00 ┆ 2021-12-16 01:00:00 ┆ 2021-12-16 01:00:00 ┆ [1, 2] │ │ 2021-12-16 01:00:00 ┆ 2021-12-16 02:00:00 ┆ 2021-12-16 02:00:00 ┆ [3, 4] │ │ 2021-12-16 02:00:00 ┆ 2021-12-16 03:00:00 ┆ 2021-12-16 03:00:00 ┆ [5, 6] │ └─────────────────────┴─────────────────────┴─────────────────────┴───────────┘
shape: (4, 4) ┌─────────────────────┬─────────────────────┬─────────────────────┬───────────┐ │ _lower_boundary ┆ _upper_boundary ┆ time ┆ n │ │ --- ┆ --- ┆ --- ┆ --- │ │ datetime[μs] ┆ datetime[μs] ┆ datetime[μs] ┆ list[i64] │ ╞═════════════════════╪═════════════════════╪═════════════════════╪═══════════╡ │ 2021-12-15 23:00:00 ┆ 2021-12-16 00:00:00 ┆ 2021-12-16 00:00:00 ┆ [0] │ │ 2021-12-16 00:00:00 ┆ 2021-12-16 01:00:00 ┆ 2021-12-16 01:00:00 ┆ [1, 2] │ │ 2021-12-16 01:00:00 ┆ 2021-12-16 02:00:00 ┆ 2021-12-16 02:00:00 ┆ [3, 4] │ │ 2021-12-16 02:00:00 ┆ 2021-12-16 03:00:00 ┆ 2021-12-16 03:00:00 ┆ [5, 6] │ └─────────────────────┴─────────────────────┴─────────────────────┴───────────┘
- datapoint : 주어진 time 리스트에서 첫 번째 값으로 레이블이 경계 중 하나에 위치할 필요가 없는 경우, datapoint를 선택하면 성능을 극대화할 수 있습니다.
print(df.group_by_dynamic( "time", every="1h", include_boundaries=True, closed="right", label='datapoint' ).agg(pl.col("n")))
print(df.group_by_dynamic( "time", every="1h", include_boundaries=True, closed="right", label='datapoint' ).agg(pl.col("n")))
shape: (4, 4) ┌─────────────────────┬─────────────────────┬─────────────────────┬───────────┐ │ _lower_boundary ┆ _upper_boundary ┆ time ┆ n │ │ --- ┆ --- ┆ --- ┆ --- │ │ datetime[μs] ┆ datetime[μs] ┆ datetime[μs] ┆ list[i64] │ ╞═════════════════════╪═════════════════════╪═════════════════════╪═══════════╡ │ 2021-12-15 23:00:00 ┆ 2021-12-16 00:00:00 ┆ 2021-12-16 00:00:00 ┆ [0] │ │ 2021-12-16 00:00:00 ┆ 2021-12-16 01:00:00 ┆ 2021-12-16 00:30:00 ┆ [1, 2] │ │ 2021-12-16 01:00:00 ┆ 2021-12-16 02:00:00 ┆ 2021-12-16 01:30:00 ┆ [3, 4] │ │ 2021-12-16 02:00:00 ┆ 2021-12-16 03:00:00 ┆ 2021-12-16 02:30:00 ┆ [5, 6] │ └─────────────────────┴─────────────────────┴─────────────────────┴───────────┘
shape: (4, 4) ┌─────────────────────┬─────────────────────┬─────────────────────┬───────────┐ │ _lower_boundary ┆ _upper_boundary ┆ time ┆ n │ │ --- ┆ --- ┆ --- ┆ --- │ │ datetime[μs] ┆ datetime[μs] ┆ datetime[μs] ┆ list[i64] │ ╞═════════════════════╪═════════════════════╪═════════════════════╪═══════════╡ │ 2021-12-15 23:00:00 ┆ 2021-12-16 00:00:00 ┆ 2021-12-16 00:00:00 ┆ [0] │ │ 2021-12-16 00:00:00 ┆ 2021-12-16 01:00:00 ┆ 2021-12-16 00:30:00 ┆ [1, 2] │ │ 2021-12-16 01:00:00 ┆ 2021-12-16 02:00:00 ┆ 2021-12-16 01:30:00 ┆ [3, 4] │ │ 2021-12-16 02:00:00 ┆ 2021-12-16 03:00:00 ┆ 2021-12-16 02:30:00 ┆ [5, 6] │ └─────────────────────┴─────────────────────┴─────────────────────┴───────────┘
- left : 시작 경계값을 time 값으로 설정합니다.
-
start_by : 첫 번째 시작 경계값을 결정하는 방법을 설정할 수 있습니다.
-
window(기본값): 열 내에 있는 가장 빠른 시간을 가져와서 간격별로 잘라냅니다. 만약, 주별은 월요일부터 시작됩니다. 출력 결과를 보시면 가장 빠른 시간인 2021-12-16 00:00:00 데이터를 가져와서 시간 간격별로 잘라낸 것을 보실 수 있습니다.
print(df.group_by_dynamic( "time", every="1h", include_boundaries=True, closed="right", start_by='window' ).agg(pl.col("n")))
print(df.group_by_dynamic( "time", every="1h", include_boundaries=True, closed="right", start_by='window' ).agg(pl.col("n")))
shape: (4, 4) ┌─────────────────────┬─────────────────────┬─────────────────────┬───────────┐ │ _lower_boundary ┆ _upper_boundary ┆ time ┆ n │ │ --- ┆ --- ┆ --- ┆ --- │ │ datetime[μs] ┆ datetime[μs] ┆ datetime[μs] ┆ list[i64] │ ╞═════════════════════╪═════════════════════╪═════════════════════╪═══════════╡ │ 2021-12-15 23:00:00 ┆ 2021-12-16 00:00:00 ┆ 2021-12-16 00:00:00 ┆ [0] │ │ 2021-12-16 00:00:00 ┆ 2021-12-16 01:00:00 ┆ 2021-12-16 00:30:00 ┆ [1, 2] │ │ 2021-12-16 01:00:00 ┆ 2021-12-16 02:00:00 ┆ 2021-12-16 01:30:00 ┆ [3, 4] │ │ 2021-12-16 02:00:00 ┆ 2021-12-16 03:00:00 ┆ 2021-12-16 02:30:00 ┆ [5, 6] │ └─────────────────────┴─────────────────────┴─────────────────────┴───────────┘
shape: (4, 4) ┌─────────────────────┬─────────────────────┬─────────────────────┬───────────┐ │ _lower_boundary ┆ _upper_boundary ┆ time ┆ n │ │ --- ┆ --- ┆ --- ┆ --- │ │ datetime[μs] ┆ datetime[μs] ┆ datetime[μs] ┆ list[i64] │ ╞═════════════════════╪═════════════════════╪═════════════════════╪═══════════╡ │ 2021-12-15 23:00:00 ┆ 2021-12-16 00:00:00 ┆ 2021-12-16 00:00:00 ┆ [0] │ │ 2021-12-16 00:00:00 ┆ 2021-12-16 01:00:00 ┆ 2021-12-16 00:30:00 ┆ [1, 2] │ │ 2021-12-16 01:00:00 ┆ 2021-12-16 02:00:00 ┆ 2021-12-16 01:30:00 ┆ [3, 4] │ │ 2021-12-16 02:00:00 ┆ 2021-12-16 03:00:00 ┆ 2021-12-16 02:30:00 ┆ [5, 6] │ └─────────────────────┴─────────────────────┴─────────────────────┴───────────┘
-
datapoint : 처음 발견된 데이터부터 시작합니다. 출력 결과를 보시면 처음 생성된 데이터인 2021-12-16 00:00:00 데이터부터 시작하므로 경계값에 없는 첫번째 행(n=0)은 출력되지 않는 것을 보실 수 있습니다.
print(df.group_by_dynamic( "time", every="1h", include_boundaries=True, closed="right", start_by='datapoint' ).agg(pl.col("n")))
print(df.group_by_dynamic( "time", every="1h", include_boundaries=True, closed="right", start_by='datapoint' ).agg(pl.col("n")))
shape: (3, 4) ┌─────────────────────┬─────────────────────┬─────────────────────┬───────────┐ │ _lower_boundary ┆ _upper_boundary ┆ time ┆ n │ │ --- ┆ --- ┆ --- ┆ --- │ │ datetime[μs] ┆ datetime[μs] ┆ datetime[μs] ┆ list[i64] │ ╞═════════════════════╪═════════════════════╪═════════════════════╪═══════════╡ │ 2021-12-16 00:00:00 ┆ 2021-12-16 01:00:00 ┆ 2021-12-16 00:00:00 ┆ [1, 2] │ │ 2021-12-16 01:00:00 ┆ 2021-12-16 02:00:00 ┆ 2021-12-16 01:00:00 ┆ [3, 4] │ │ 2021-12-16 02:00:00 ┆ 2021-12-16 03:00:00 ┆ 2021-12-16 02:00:00 ┆ [5, 6] │ └─────────────────────┴─────────────────────┴─────────────────────┴───────────┘
shape: (3, 4) ┌─────────────────────┬─────────────────────┬─────────────────────┬───────────┐ │ _lower_boundary ┆ _upper_boundary ┆ time ┆ n │ │ --- ┆ --- ┆ --- ┆ --- │ │ datetime[μs] ┆ datetime[μs] ┆ datetime[μs] ┆ list[i64] │ ╞═════════════════════╪═════════════════════╪═════════════════════╪═══════════╡ │ 2021-12-16 00:00:00 ┆ 2021-12-16 01:00:00 ┆ 2021-12-16 00:00:00 ┆ [1, 2] │ │ 2021-12-16 01:00:00 ┆ 2021-12-16 02:00:00 ┆ 2021-12-16 01:00:00 ┆ [3, 4] │ │ 2021-12-16 02:00:00 ┆ 2021-12-16 03:00:00 ┆ 2021-12-16 02:00:00 ┆ [5, 6] │ └─────────────────────┴─────────────────────┴─────────────────────┴───────────┘
offset 매개변수를 설명할 때,
start_by='datapoint'
인 경우 offset이 적용되지 않는다고 언급했었습니다.offset
을 10분으로 적용했을때 어떻게 출력되는지 보도록 하겠습니다. 출력 결과를 보시면 offset이 적용되지 않고 출력된 것을 보실 수 있습니다.print(df.group_by_dynamic( "time", every="1h", include_boundaries=True, closed="right", start_by='datapoint', offset='10m' ).agg(pl.col("n")))
print(df.group_by_dynamic( "time", every="1h", include_boundaries=True, closed="right", start_by='datapoint', offset='10m' ).agg(pl.col("n")))
shape: (3, 4) ┌─────────────────────┬─────────────────────┬─────────────────────┬───────────┐ │ _lower_boundary ┆ _upper_boundary ┆ time ┆ n │ │ --- ┆ --- ┆ --- ┆ --- │ │ datetime[μs] ┆ datetime[μs] ┆ datetime[μs] ┆ list[i64] │ ╞═════════════════════╪═════════════════════╪═════════════════════╪═══════════╡ │ 2021-12-16 00:00:00 ┆ 2021-12-16 01:00:00 ┆ 2021-12-16 00:00:00 ┆ [1, 2] │ │ 2021-12-16 01:00:00 ┆ 2021-12-16 02:00:00 ┆ 2021-12-16 01:00:00 ┆ [3, 4] │ │ 2021-12-16 02:00:00 ┆ 2021-12-16 03:00:00 ┆ 2021-12-16 02:00:00 ┆ [5, 6] │ └─────────────────────┴─────────────────────┴─────────────────────┴───────────┘
shape: (3, 4) ┌─────────────────────┬─────────────────────┬─────────────────────┬───────────┐ │ _lower_boundary ┆ _upper_boundary ┆ time ┆ n │ │ --- ┆ --- ┆ --- ┆ --- │ │ datetime[μs] ┆ datetime[μs] ┆ datetime[μs] ┆ list[i64] │ ╞═════════════════════╪═════════════════════╪═════════════════════╪═══════════╡ │ 2021-12-16 00:00:00 ┆ 2021-12-16 01:00:00 ┆ 2021-12-16 00:00:00 ┆ [1, 2] │ │ 2021-12-16 01:00:00 ┆ 2021-12-16 02:00:00 ┆ 2021-12-16 01:00:00 ┆ [3, 4] │ │ 2021-12-16 02:00:00 ┆ 2021-12-16 03:00:00 ┆ 2021-12-16 02:00:00 ┆ [5, 6] │ └─────────────────────┴─────────────────────┴─────────────────────┴───────────┘
-
요일 : 주별로 그룹화 할 때(every에 'w'가 포함된 경우), monday, tuesday, wednesday, thursday, friday, saturday, sunday 값이 인수로 들어갈 수 있습니다. df 데이터프레임 마지막 행에 new_row 데이터프레임을 추가해보도록 하겠습니다.
new_row = pl.DataFrame({ "time": [datetime(2021, 12, 17, 2, 0, 0),datetime(2021, 12, 18, 2, 0, 0),datetime(2021, 12, 19, 2, 0, 0),datetime(2021, 12, 20, 2, 0, 0)], "n": [4,6,3,2] }) new_df = df.vstack(new_row) print(new_df)
new_row = pl.DataFrame({ "time": [datetime(2021, 12, 17, 2, 0, 0),datetime(2021, 12, 18, 2, 0, 0),datetime(2021, 12, 19, 2, 0, 0),datetime(2021, 12, 20, 2, 0, 0)], "n": [4,6,3,2] }) new_df = df.vstack(new_row) print(new_df)
shape: (11, 2) ┌─────────────────────┬─────┐ │ time ┆ n │ │ --- ┆ --- │ │ datetime[μs] ┆ i64 │ ╞═════════════════════╪═════╡ │ 2021-12-16 00:00:00 ┆ 0 │ │ 2021-12-16 00:30:00 ┆ 1 │ │ 2021-12-16 01:00:00 ┆ 2 │ │ 2021-12-16 01:30:00 ┆ 3 │ │ 2021-12-16 02:00:00 ┆ 4 │ │ … ┆ … │ │ 2021-12-16 03:00:00 ┆ 6 │ │ 2021-12-17 02:00:00 ┆ 4 │ │ 2021-12-18 02:00:00 ┆ 6 │ │ 2021-12-19 02:00:00 ┆ 3 │ │ 2021-12-20 02:00:00 ┆ 2 │ └─────────────────────┴─────┘
shape: (11, 2) ┌─────────────────────┬─────┐ │ time ┆ n │ │ --- ┆ --- │ │ datetime[μs] ┆ i64 │ ╞═════════════════════╪═════╡ │ 2021-12-16 00:00:00 ┆ 0 │ │ 2021-12-16 00:30:00 ┆ 1 │ │ 2021-12-16 01:00:00 ┆ 2 │ │ 2021-12-16 01:30:00 ┆ 3 │ │ 2021-12-16 02:00:00 ┆ 4 │ │ … ┆ … │ │ 2021-12-16 03:00:00 ┆ 6 │ │ 2021-12-17 02:00:00 ┆ 4 │ │ 2021-12-18 02:00:00 ┆ 6 │ │ 2021-12-19 02:00:00 ┆ 3 │ │ 2021-12-20 02:00:00 ┆ 2 │ └─────────────────────┴─────┘
이번에는 time열을 기준으로 일주일 간격으로 그룹화를 진행해보도록 하겠습니다. 이때, start_by를sunday로 설정할 경우 시작 경계값의 날짜의 요일이 전주 일요일부터 시작하게 됩니다. 출력 결과는 가장 빠른 시간이 범위 안에 있거나 앞에 있을 때까지 뒤로 이동합니다.
print(new_df.group_by_dynamic( "time", every="1w", include_boundaries=True, closed="right", start_by='sunday' ).agg(pl.col("n")))
print(new_df.group_by_dynamic( "time", every="1w", include_boundaries=True, closed="right", start_by='sunday' ).agg(pl.col("n")))
shape: (2, 4) ┌─────────────────────┬─────────────────────┬─────────────────────┬─────────────┐ │ _lower_boundary ┆ _upper_boundary ┆ time ┆ n │ │ --- ┆ --- ┆ --- ┆ --- │ │ datetime[μs] ┆ datetime[μs] ┆ datetime[μs] ┆ list[i64] │ ╞═════════════════════╪═════════════════════╪═════════════════════╪═════════════╡ │ 2021-12-12 00:00:00 ┆ 2021-12-19 00:00:00 ┆ 2021-12-12 00:00:00 ┆ [0, 1, … 6] │ │ 2021-12-19 00:00:00 ┆ 2021-12-26 00:00:00 ┆ 2021-12-19 00:00:00 ┆ [3, 2] │ └─────────────────────┴─────────────────────┴─────────────────────┴─────────────┘
shape: (2, 4) ┌─────────────────────┬─────────────────────┬─────────────────────┬─────────────┐ │ _lower_boundary ┆ _upper_boundary ┆ time ┆ n │ │ --- ┆ --- ┆ --- ┆ --- │ │ datetime[μs] ┆ datetime[μs] ┆ datetime[μs] ┆ list[i64] │ ╞═════════════════════╪═════════════════════╪═════════════════════╪═════════════╡ │ 2021-12-12 00:00:00 ┆ 2021-12-19 00:00:00 ┆ 2021-12-12 00:00:00 ┆ [0, 1, … 6] │ │ 2021-12-19 00:00:00 ┆ 2021-12-26 00:00:00 ┆ 2021-12-19 00:00:00 ┆ [3, 2] │ └─────────────────────┴─────────────────────┴─────────────────────┴─────────────┘
만약, start_by를 따로 설정하지 않으면 월요일을 기준으로 그룹화하는 것을 보실 수 있습니다.
print(new_df.group_by_dynamic( "time", every="1w", include_boundaries=True, closed="right" ).agg(pl.col("n")))
print(new_df.group_by_dynamic( "time", every="1w", include_boundaries=True, closed="right" ).agg(pl.col("n")))
shape: (2, 4) ┌─────────────────────┬─────────────────────┬─────────────────────┬─────────────┐ │ _lower_boundary ┆ _upper_boundary ┆ time ┆ n │ │ --- ┆ --- ┆ --- ┆ --- │ │ datetime[μs] ┆ datetime[μs] ┆ datetime[μs] ┆ list[i64] │ ╞═════════════════════╪═════════════════════╪═════════════════════╪═════════════╡ │ 2021-12-13 00:00:00 ┆ 2021-12-20 00:00:00 ┆ 2021-12-13 00:00:00 ┆ [0, 1, … 3] │ │ 2021-12-20 00:00:00 ┆ 2021-12-27 00:00:00 ┆ 2021-12-20 00:00:00 ┆ [3, 2] │ └─────────────────────┴─────────────────────┴─────────────────────┴─────────────┘
shape: (2, 4) ┌─────────────────────┬─────────────────────┬─────────────────────┬─────────────┐ │ _lower_boundary ┆ _upper_boundary ┆ time ┆ n │ │ --- ┆ --- ┆ --- ┆ --- │ │ datetime[μs] ┆ datetime[μs] ┆ datetime[μs] ┆ list[i64] │ ╞═════════════════════╪═════════════════════╪═════════════════════╪═════════════╡ │ 2021-12-13 00:00:00 ┆ 2021-12-20 00:00:00 ┆ 2021-12-13 00:00:00 ┆ [0, 1, … 3] │ │ 2021-12-20 00:00:00 ┆ 2021-12-27 00:00:00 ┆ 2021-12-20 00:00:00 ┆ [3, 2] │ └─────────────────────┴─────────────────────┴─────────────────────┴─────────────┘
-
-
group_by : group_by_dynamic 메서드는 다른 컬럼 그룹화와 결합할 수도 있습니다. group_by 매개변수에 컬럼명을 입력하여 어떤 열을 기준으로 그룹별로 집계할 지 정할 수 있습니다. group_by 메서드가 전달되면 출력 결과는 그룹화 할 컬럼을 기준으로 각 그룹 내에서 오름차순으로 정렬됩니다. 먼저 df 데이터프레임에 groups라는 컬럼을 추가해보도록 하겠습니다.
df = df.with_columns(groups=pl.Series(["a", "a", "a", "b", "b", "a", "a"])) print(df)
df = df.with_columns(groups=pl.Series(["a", "a", "a", "b", "b", "a", "a"])) print(df)
shape: (7, 3) ┌─────────────────────┬─────┬────────┐ │ time ┆ n ┆ groups │ │ --- ┆ --- ┆ --- │ │ datetime[μs] ┆ i64 ┆ str │ ╞═════════════════════╪═════╪════════╡ │ 2021-12-16 00:00:00 ┆ 0 ┆ a │ │ 2021-12-16 00:30:00 ┆ 1 ┆ a │ │ 2021-12-16 01:00:00 ┆ 2 ┆ a │ │ 2021-12-16 01:30:00 ┆ 3 ┆ b │ │ 2021-12-16 02:00:00 ┆ 4 ┆ b │ │ 2021-12-16 02:30:00 ┆ 5 ┆ a │ │ 2021-12-16 03:00:00 ┆ 6 ┆ a │ └─────────────────────┴─────┴────────┘
shape: (7, 3) ┌─────────────────────┬─────┬────────┐ │ time ┆ n ┆ groups │ │ --- ┆ --- ┆ --- │ │ datetime[μs] ┆ i64 ┆ str │ ╞═════════════════════╪═════╪════════╡ │ 2021-12-16 00:00:00 ┆ 0 ┆ a │ │ 2021-12-16 00:30:00 ┆ 1 ┆ a │ │ 2021-12-16 01:00:00 ┆ 2 ┆ a │ │ 2021-12-16 01:30:00 ┆ 3 ┆ b │ │ 2021-12-16 02:00:00 ┆ 4 ┆ b │ │ 2021-12-16 02:30:00 ┆ 5 ┆ a │ │ 2021-12-16 03:00:00 ┆ 6 ┆ a │ └─────────────────────┴─────┴────────┘
group_by를 groups로 설정하여 groups를 기준으로 먼저 그룹화한 후에 time을 기준으로 그룹화를 진행해보도록 하겠습니다.
print( df.group_by_dynamic( "time", every="1h", closed="both", group_by="groups", include_boundaries=True, ).agg(pl.col("n")) )
print( df.group_by_dynamic( "time", every="1h", closed="both", group_by="groups", include_boundaries=True, ).agg(pl.col("n")) )
shape: (7, 5) ┌────────┬─────────────────────┬─────────────────────┬─────────────────────┬───────────┐ │ groups ┆ _lower_boundary ┆ _upper_boundary ┆ time ┆ n │ │ --- ┆ --- ┆ --- ┆ --- ┆ --- │ │ str ┆ datetime[μs] ┆ datetime[μs] ┆ datetime[μs] ┆ list[i64] │ ╞════════╪═════════════════════╪═════════════════════╪═════════════════════╪═══════════╡ │ a ┆ 2021-12-15 23:00:00 ┆ 2021-12-16 00:00:00 ┆ 2021-12-15 23:00:00 ┆ [0] │ │ a ┆ 2021-12-16 00:00:00 ┆ 2021-12-16 01:00:00 ┆ 2021-12-16 00:00:00 ┆ [0, 1, 2] │ │ a ┆ 2021-12-16 01:00:00 ┆ 2021-12-16 02:00:00 ┆ 2021-12-16 01:00:00 ┆ [2] │ │ a ┆ 2021-12-16 02:00:00 ┆ 2021-12-16 03:00:00 ┆ 2021-12-16 02:00:00 ┆ [5, 6] │ │ a ┆ 2021-12-16 03:00:00 ┆ 2021-12-16 04:00:00 ┆ 2021-12-16 03:00:00 ┆ [6] │ │ b ┆ 2021-12-16 01:00:00 ┆ 2021-12-16 02:00:00 ┆ 2021-12-16 01:00:00 ┆ [3, 4] │ │ b ┆ 2021-12-16 02:00:00 ┆ 2021-12-16 03:00:00 ┆ 2021-12-16 02:00:00 ┆ [4] │ └────────┴─────────────────────┴─────────────────────┴─────────────────────┴───────────┘
shape: (7, 5) ┌────────┬─────────────────────┬─────────────────────┬─────────────────────┬───────────┐ │ groups ┆ _lower_boundary ┆ _upper_boundary ┆ time ┆ n │ │ --- ┆ --- ┆ --- ┆ --- ┆ --- │ │ str ┆ datetime[μs] ┆ datetime[μs] ┆ datetime[μs] ┆ list[i64] │ ╞════════╪═════════════════════╪═════════════════════╪═════════════════════╪═══════════╡ │ a ┆ 2021-12-15 23:00:00 ┆ 2021-12-16 00:00:00 ┆ 2021-12-15 23:00:00 ┆ [0] │ │ a ┆ 2021-12-16 00:00:00 ┆ 2021-12-16 01:00:00 ┆ 2021-12-16 00:00:00 ┆ [0, 1, 2] │ │ a ┆ 2021-12-16 01:00:00 ┆ 2021-12-16 02:00:00 ┆ 2021-12-16 01:00:00 ┆ [2] │ │ a ┆ 2021-12-16 02:00:00 ┆ 2021-12-16 03:00:00 ┆ 2021-12-16 02:00:00 ┆ [5, 6] │ │ a ┆ 2021-12-16 03:00:00 ┆ 2021-12-16 04:00:00 ┆ 2021-12-16 03:00:00 ┆ [6] │ │ b ┆ 2021-12-16 01:00:00 ┆ 2021-12-16 02:00:00 ┆ 2021-12-16 01:00:00 ┆ [3, 4] │ │ b ┆ 2021-12-16 02:00:00 ┆ 2021-12-16 03:00:00 ┆ 2021-12-16 02:00:00 ┆ [4] │ └────────┴─────────────────────┴─────────────────────┴─────────────────────┴───────────┘
group_by_dynamic 메서드는 group_by 메서드와 다른 점은 every, period, offset, closed, group_by, start_by 매개변수 인수 설정에 따라 하나의 행이 여러 그룹의 멤버가 될 수 있다는 점입니다.
-
period: 시작 경계값과 종료 경계값 사이의 간격 길이를 의미합니다. None으로 설정할 경우 every와 같습니다. 만약, 정수 열을 그룹화하려는 경우 1i(1 인덱스 수) 으로 작성합니다. ex) 1i(길이 1), 10i(길이 10)
df = pl.DataFrame( { "idx": pl.int_range(0, 6, eager=True), "A": ["A", "A", "B", "B", "B", "C"], } ) print(df)
df = pl.DataFrame( { "idx": pl.int_range(0, 6, eager=True), "A": ["A", "A", "B", "B", "B", "C"], } ) print(df)
- pl.int_range(start, end, eager) : start부터 end값까지 1씩 증가하여 반환합니다.
shape: (6, 2) ┌─────┬─────┐ │ idx ┆ A │ │ --- ┆ --- │ │ i64 ┆ str │ ╞═════╪═════╡ │ 0 ┆ A │ │ 1 ┆ A │ │ 2 ┆ B │ │ 3 ┆ B │ │ 4 ┆ B │ │ 5 ┆ C │ └─────┴─────┘
shape: (6, 2) ┌─────┬─────┐ │ idx ┆ A │ │ --- ┆ --- │ │ i64 ┆ str │ ╞═════╪═════╡ │ 0 ┆ A │ │ 1 ┆ A │ │ 2 ┆ B │ │ 3 ┆ B │ │ 4 ┆ B │ │ 5 ┆ C │ └─────┴─────┘
이번에는 idx를 기준으로 시작 경계값을 2씩 건너뛰고, 시작 경계값과 종료 경계값 사이의 간격은 3으로 설정하여 A 열을 그룹화해보도록 하겠습니다.
print(( df.group_by_dynamic( "idx", every="2i", period="3i", include_boundaries=True, closed="right", ).agg(pl.col("A").alias("A_agg_list")) ))
print(( df.group_by_dynamic( "idx", every="2i", period="3i", include_boundaries=True, closed="right", ).agg(pl.col("A").alias("A_agg_list")) ))
shape: (4, 4) ┌─────────────────┬─────────────────┬─────┬─────────────────┐ │ _lower_boundary ┆ _upper_boundary ┆ idx ┆ A_agg_list │ │ --- ┆ --- ┆ --- ┆ --- │ │ i64 ┆ i64 ┆ i64 ┆ list[str] │ ╞═════════════════╪═════════════════╪═════╪═════════════════╡ │ -2 ┆ 1 ┆ -2 ┆ ["A", "A"] │ │ 0 ┆ 3 ┆ 0 ┆ ["A", "B", "B"] │ │ 2 ┆ 5 ┆ 2 ┆ ["B", "B", "C"] │ │ 4 ┆ 7 ┆ 4 ┆ ["C"] │ └─────────────────┴─────────────────┴─────┴─────────────────┘
shape: (4, 4) ┌─────────────────┬─────────────────┬─────┬─────────────────┐ │ _lower_boundary ┆ _upper_boundary ┆ idx ┆ A_agg_list │ │ --- ┆ --- ┆ --- ┆ --- │ │ i64 ┆ i64 ┆ i64 ┆ list[str] │ ╞═════════════════╪═════════════════╪═════╪═════════════════╡ │ -2 ┆ 1 ┆ -2 ┆ ["A", "A"] │ │ 0 ┆ 3 ┆ 0 ┆ ["A", "B", "B"] │ │ 2 ┆ 5 ┆ 2 ┆ ["B", "B", "C"] │ │ 4 ┆ 7 ┆ 4 ┆ ["C"] │ └─────────────────┴─────────────────┴─────┴─────────────────┘
숫자형 자료형을 그룹화 할 경우, 데이터 타입은 Int32, Int64 중 하나이어야 합니다. Int32는 일시적으로 Int64로 형변환되므로 성능이 중요한 경우 Int64 열을 사용시길 바랍니다.
이번에는 Polars의 group_by_dynamic과 Pandas의 resample을 비교해보도록 하겠습니다. 먼저, Polars의 group_by_dynamic을 사용해 time열을 기준으로 1시간 단위로 묶어 n의 합계를 계산해보도록 하겠습니다.
# polars
from datetime import datetime
import polars as pl
df = pl.DataFrame(
{
"time": pl.datetime_range(
start=datetime(2021, 12, 16),
end=datetime(2021, 12, 16, 3),
interval="30m",
eager=True,
),
"n": range(7),
}
)
print(df.group_by_dynamic("time", every="1h").agg(pl.col("n").sum()))
# polars
from datetime import datetime
import polars as pl
df = pl.DataFrame(
{
"time": pl.datetime_range(
start=datetime(2021, 12, 16),
end=datetime(2021, 12, 16, 3),
interval="30m",
eager=True,
),
"n": range(7),
}
)
print(df.group_by_dynamic("time", every="1h").agg(pl.col("n").sum()))
shape: (4, 2)
┌─────────────────────┬─────┐
│ time ┆ n │
│ --- ┆ --- │
│ datetime[μs] ┆ i64 │
╞═════════════════════╪═════╡
│ 2021-12-16 00:00:00 ┆ 1 │
│ 2021-12-16 01:00:00 ┆ 5 │
│ 2021-12-16 02:00:00 ┆ 9 │
│ 2021-12-16 03:00:00 ┆ 6 │
└─────────────────────┴─────┘
shape: (4, 2)
┌─────────────────────┬─────┐
│ time ┆ n │
│ --- ┆ --- │
│ datetime[μs] ┆ i64 │
╞═════════════════════╪═════╡
│ 2021-12-16 00:00:00 ┆ 1 │
│ 2021-12-16 01:00:00 ┆ 5 │
│ 2021-12-16 02:00:00 ┆ 9 │
│ 2021-12-16 03:00:00 ┆ 6 │
└─────────────────────┴─────┘
이번에는 Pandas resample을 사용해 time열을 기준으로 1시간 단위로 묶어 n의 합계를 계산해보도록 하겠습니다.
# pandas
import pandas as pd
df = pd.DataFrame(
{
"time": pd.date_range(
start=datetime(2021, 12, 16),
end=datetime(2021, 12, 16, 3),
freq="30min"
),
"n": range(7),
}
)
print(df.set_index("time").resample("h")["n"].sum().reset_index())
# pandas
import pandas as pd
df = pd.DataFrame(
{
"time": pd.date_range(
start=datetime(2021, 12, 16),
end=datetime(2021, 12, 16, 3),
freq="30min"
),
"n": range(7),
}
)
print(df.set_index("time").resample("h")["n"].sum().reset_index())
- pd.date_range(start, end, freq) : 시작 날짜부터 종료날짜까지 간격별 데이터를 생성합니다.
- set_index(’컬럼명’) : 해당 컬럼명을 인덱스로 설정합니다.
- reset_index() : 인덱스 설정을 해제합니다.
- resample(’날짜/시간 단위’) : 날짜/시간 단위를 기준으로 그룹화합니다.
time n
0 2021-12-16 00:00:00 1
1 2021-12-16 01:00:00 5
2 2021-12-16 02:00:00 9
3 2021-12-16 03:00:00 6
time n
0 2021-12-16 00:00:00 1
1 2021-12-16 01:00:00 5
2 2021-12-16 02:00:00 9
3 2021-12-16 03:00:00 6
이처럼 Polars의 group_by_dynamic는 Pandas의 resample로 사용하실 수 있습니다. 두 코드의 출력 결과는 동일하게 작동하지만, Polars는 Pandas와 달리 데이터가 있는 시간대만 보여줍니다. 만약, 시간 간격을 정확히 같은 간격으로 맞추고 싶거나, 아니면 불규칙한 시간 데이터를 규칙적인 간격으로 정리하고 싶을때나, index_column의 간격을 균등하게 유지해야 하는 경우 Pandas resample()을 사용하시거나 결합하여 사용하시길 바랍니다.
2.3 upsample
업샘플링은 만약 친구와 한달에 한번 만나기로 해서 아래와 같이 계획을 짰는데 일주일에 한번 만나기로 했을 경우 월 단위가 아닌 일주일 단위로 데이터를 추가해야겠죠? 이때, upsample을 사용하여 오른쪽 테이블과 같이 일주일 단위로 날짜를 추가할 수 있습니다.
날짜 | 인원 |
---|---|
2021-06-01 | 3 |
2021-07-01 | 2 |
… | … |
2021-12-01 | 5 |
— upsample →
날짜 | 인원 |
---|---|
2021-06-01 | 3 |
2021-06-08 | null |
2021-06-15 | null |
… | |
2021-11-30 | null |
또는 2월과 5월에 만나자고 했는데 3, 4월에도 만나기로 계획을 변경했을 경우 데이터를 아래와 같이 추가할 때 사용하는 메서드입니다.
날짜 | 인원 |
---|---|
2021-02-01 | 3 |
2021-05-01 | 2 |
— upsample →
날짜 | 인원 |
---|---|
2021-02-01 | 3 |
2021-03-01 | null |
2021-04-01 | null |
2021-05-01 | 2 |
이처럼 특정 간격으로 데이터프레임을 업샘플링합니다. time_column을 기준으로 정렬되며, group_by 열을 전달하면 각 그룹 내에서만 정렬됩니다.
- 1ns(1나노초)
- 1us(1마이크로초)
- 1ms(1밀리초)
- 1s(1초)
- 1m(1분)
- 1h(1시간)
- 1d(1역일)
- 1w(1역주)
- 1mo(1역월)
- 1q(1역분기)
- 1y(1역연도)
ex) 3d12h4m25s (3일, 12시간 4분 25초)
:::div{.callout}
'역일'이란 다음 날의 해당 시간(서머타임으로 인해 24시간이 아닐 수 있음)을 의미합니다. '역주', '역월', '역분기', '역연도'도 마찬가지입니다.
:::
:::div{.callout}
'역일'이란 다음 날의 해당 시간(서머타임으로 인해 24시간이 아닐 수 있음)을 의미합니다. '역주', '역월', '역분기', '역연도'도 마찬가지입니다.
:::
from datetime import datetime
df = pl.DataFrame(
{
"time": [
datetime(2021, 6, 1),
datetime(2021, 2, 1),
datetime(2021, 4, 1),
datetime(2021, 5, 1),
],
"groups": ["A", "B", "A", "B"],
"values": [0, 1, 2, 3],
}
).sort("time")
print(df)
from datetime import datetime
df = pl.DataFrame(
{
"time": [
datetime(2021, 6, 1),
datetime(2021, 2, 1),
datetime(2021, 4, 1),
datetime(2021, 5, 1),
],
"groups": ["A", "B", "A", "B"],
"values": [0, 1, 2, 3],
}
).sort("time")
print(df)
shape: (4, 3)
┌─────────────────────┬────────┬────────┐
│ time ┆ groups ┆ values │
│ --- ┆ --- ┆ --- │
│ datetime[μs] ┆ str ┆ i64 │
╞═════════════════════╪════════╪════════╡
│ 2021-02-01 00:00:00 ┆ A ┆ 0 │
│ 2021-04-01 00:00:00 ┆ B ┆ 1 │
│ 2021-05-01 00:00:00 ┆ A ┆ 2 │
│ 2021-06-01 00:00:00 ┆ B ┆ 3 │
└─────────────────────┴────────┴────────┘
shape: (4, 3)
┌─────────────────────┬────────┬────────┐
│ time ┆ groups ┆ values │
│ --- ┆ --- ┆ --- │
│ datetime[μs] ┆ str ┆ i64 │
╞═════════════════════╪════════╪════════╡
│ 2021-02-01 00:00:00 ┆ A ┆ 0 │
│ 2021-04-01 00:00:00 ┆ B ┆ 1 │
│ 2021-05-01 00:00:00 ┆ A ┆ 2 │
│ 2021-06-01 00:00:00 ┆ B ┆ 3 │
└─────────────────────┴────────┴────────┘
time 컬럼을 기준으로 한달로 묶어 upsample을 진행하고 groups로 데이터를 묶어 보도록 하겠습니다. 이때, 출력 결과는 time을 기준으로 정렬되며, group_by 열을 전달하면 각 그룹 내에서만 정렬됩니다.
# df.upsample(time_column="컬럼명", every="간격", group_by="그룹화 할 컬럼명", maintain_order=True)
print(df.upsample(
time_column="time", every="1mo", group_by="groups", maintain_order=True
))
# df.upsample(time_column="컬럼명", every="간격", group_by="그룹화 할 컬럼명", maintain_order=True)
print(df.upsample(
time_column="time", every="1mo", group_by="groups", maintain_order=True
))
-
time_column : 날짜 범위를 결정하는데 사용되며, 해당 열을 정렬한 후 실행하셔야 합니다. 만약, 정렬하지 않을 경우 에러가 뜨게 됩니다.
from datetime import datetime df = pl.DataFrame( { "time": [ datetime(2021, 6, 1), datetime(2021, 2, 1), datetime(2021, 4, 1), datetime(2021, 5, 1), ], "groups": ["A", "B", "A", "B"], "values": [0, 1, 2, 3], } ) print(df)
from datetime import datetime df = pl.DataFrame( { "time": [ datetime(2021, 6, 1), datetime(2021, 2, 1), datetime(2021, 4, 1), datetime(2021, 5, 1), ], "groups": ["A", "B", "A", "B"], "values": [0, 1, 2, 3], } ) print(df)
shape: (4, 3) ┌─────────────────────┬────────┬────────┐ │ time ┆ groups ┆ values │ │ --- ┆ --- ┆ --- │ │ datetime[μs] ┆ str ┆ i64 │ ╞═════════════════════╪════════╪════════╡ │ 2021-06-01 00:00:00 ┆ A ┆ 0 │ │ 2021-02-01 00:00:00 ┆ B ┆ 1 │ │ 2021-04-01 00:00:00 ┆ A ┆ 2 │ │ 2021-05-01 00:00:00 ┆ B ┆ 3 │ └─────────────────────┴────────┴────────┘
shape: (4, 3) ┌─────────────────────┬────────┬────────┐ │ time ┆ groups ┆ values │ │ --- ┆ --- ┆ --- │ │ datetime[μs] ┆ str ┆ i64 │ ╞═════════════════════╪════════╪════════╡ │ 2021-06-01 00:00:00 ┆ A ┆ 0 │ │ 2021-02-01 00:00:00 ┆ B ┆ 1 │ │ 2021-04-01 00:00:00 ┆ A ┆ 2 │ │ 2021-05-01 00:00:00 ┆ B ┆ 3 │ └─────────────────────┴────────┴────────┘
print(df.upsample( time_column="time", every="1mo", group_by="groups", maintain_order=True ))
print(df.upsample( time_column="time", every="1mo", group_by="groups", maintain_order=True ))
InvalidOperationError: argument in operation 'upsample' is not sorted, please sort the 'expr/series/column' first
InvalidOperationError: argument in operation 'upsample' is not sorted, please sort the 'expr/series/column' first
-
every : 모든 기간을 해당 단위를 기준으로 쪼갭니다.
-
group_by : 특정 열을 기준으로 먼저 그룹화한 다음 모든 그룹에 대해 업샘플링합니다.
-
maintain_order : 기본값은 False이며, True로 설정할 경우 원본 데이터프레임 순서를 유지하기 때문에 속도가 느릴 수 있습니다.
shape: (7, 3)
┌─────────────────────┬────────┬────────┐
│ time ┆ groups ┆ values │
│ --- ┆ --- ┆ --- │
│ datetime[μs] ┆ str ┆ i64 │
╞═════════════════════╪════════╪════════╡
│ 2021-02-01 00:00:00 ┆ A ┆ 0 │
│ 2021-03-01 00:00:00 ┆ null ┆ null │
│ 2021-04-01 00:00:00 ┆ null ┆ null │
│ 2021-05-01 00:00:00 ┆ A ┆ 2 │
│ 2021-04-01 00:00:00 ┆ B ┆ 1 │
│ 2021-05-01 00:00:00 ┆ null ┆ null │
│ 2021-06-01 00:00:00 ┆ B ┆ 3 │
└─────────────────────┴────────┴────────┘
shape: (7, 3)
┌─────────────────────┬────────┬────────┐
│ time ┆ groups ┆ values │
│ --- ┆ --- ┆ --- │
│ datetime[μs] ┆ str ┆ i64 │
╞═════════════════════╪════════╪════════╡
│ 2021-02-01 00:00:00 ┆ A ┆ 0 │
│ 2021-03-01 00:00:00 ┆ null ┆ null │
│ 2021-04-01 00:00:00 ┆ null ┆ null │
│ 2021-05-01 00:00:00 ┆ A ┆ 2 │
│ 2021-04-01 00:00:00 ┆ B ┆ 1 │
│ 2021-05-01 00:00:00 ┆ null ┆ null │
│ 2021-06-01 00:00:00 ┆ B ┆ 3 │
└─────────────────────┴────────┴────────┘
2.4 rolling
rolling 메서드는 시간 또는 정수 열을 기준으로 롤링 그룹을 만듭니다. 일정한 간격으로 그룹화하는 group_by_dynamic과 달리 개별 값에 의해 결정됩니다.
만약, 시계열 <t_0, t_1, ..., t_n>이 있는 경우 기본적으로 생성되는 범위는 아래와 같습니다.
- (t_0 - period, t_0]
- (t_1 - period, t_1]
- …
- (t_n - period, t_n]
기본값이 아닌 offset을 전달하면 범위는 아래와 같습니다.
- (t_0 + offset, t_0 + offset + period]
- (t_1 + offset, t_1 + offset + period]
- …
- (t_n + offset, t_n + offset + period]
period 및 offset 인수는 timedelta에서 생성하거나 아래 문자열을 사용하여 생성합니다.
- 1ns(1나노초)
- 1us(1마이크로초)
- 1ms(1밀리초)
- 1s(1초)
- 1m(1분)
- 1h(1시간)
- 1d(1역일)
- 1w(1역주)
- 1mo(1역월)
- 1q(1역분기)
- 1y(1역연도)
ex) 3d12h4m25s (3일, 12시간 4분 25초)
:::div{.callout}
'역일'이란 다음 날의 해당 시간(서머타임으로 인해 24시간이 아닐 수 있음)을 의미합니다. '역주', '역월', '역분기', '역연도'도 마찬가지입니다.
:::
:::div{.callout}
'역일'이란 다음 날의 해당 시간(서머타임으로 인해 24시간이 아닐 수 있음)을 의미합니다. '역주', '역월', '역분기', '역연도'도 마찬가지입니다.
:::
먼저, 데이터프레임을 생성해보도록 하겠습니다.
dates = [
"2020-01-01 13:45:48",
"2020-01-01 16:42:13",
"2020-01-01 16:45:09",
"2020-01-02 18:12:48",
"2020-01-03 19:45:32",
"2020-01-08 23:16:43",
]
df = pl.DataFrame({"dt": dates, "a": [3, 7, 5, 9, 2, 1]}).with_columns(
pl.col("dt").str.strptime(pl.Datetime).sort()
)
dates = [
"2020-01-01 13:45:48",
"2020-01-01 16:42:13",
"2020-01-01 16:45:09",
"2020-01-02 18:12:48",
"2020-01-03 19:45:32",
"2020-01-08 23:16:43",
]
df = pl.DataFrame({"dt": dates, "a": [3, 7, 5, 9, 2, 1]}).with_columns(
pl.col("dt").str.strptime(pl.Datetime).sort()
)
- .str.strptime() : 문자열을 datetime 객체로 파싱(parsing)하는 함수입니다.
rolling 메서드를 사용해 dt를 기준으로 2일 간격의 데이터를 그룹화 하여 그룹화된 a열의 값들, 합계, 최소값, 최대값을 구해보도록 하겠습니다. 출력 결과를 보시면 각각의 행마다 개별 값에 의해 2일씩 묶인 것을 보실 수가 있습니다.
# df.rolling(index_column="컬럼명", period="길이").agg(집계관련코드)
out = df.rolling(index_column="dt", period="2d").agg(
[
pl.col("a"),
pl.sum("a").alias("sum_a"),
pl.min("a").alias("min_a"),
pl.max("a").alias("max_a"),
]
)
assert out["sum_a"].to_list() == [3, 10, 15, 24, 11, 1]
assert out["max_a"].to_list() == [3, 7, 7, 9, 9, 1]
assert out["min_a"].to_list() == [3, 3, 3, 3, 2, 1]
print(out)
# df.rolling(index_column="컬럼명", period="길이").agg(집계관련코드)
out = df.rolling(index_column="dt", period="2d").agg(
[
pl.col("a"),
pl.sum("a").alias("sum_a"),
pl.min("a").alias("min_a"),
pl.max("a").alias("max_a"),
]
)
assert out["sum_a"].to_list() == [3, 10, 15, 24, 11, 1]
assert out["max_a"].to_list() == [3, 7, 7, 9, 9, 1]
assert out["min_a"].to_list() == [3, 3, 3, 3, 2, 1]
print(out)
- index_column : 시간을 기준으로 그룹화하는 데 사용되는 열로 보통 날짜/시간 데이터 타입입니다. 이때, 그룹화할 열은 오름차순으로 정렬해야 하며, 오름차순으로 정렬하지 않을 경우 에러가 납니다.
- period : 그룹화 할 날짜/시간 길이, 음수가 아닌 값
shape: (6, 5)
┌─────────────────────┬─────────────┬───────┬───────┬───────┐
│ dt ┆ a ┆ sum_a ┆ min_a ┆ max_a │
│ --- ┆ --- ┆ --- ┆ --- ┆ --- │
│ datetime[μs] ┆ list[i64] ┆ i64 ┆ i64 ┆ i64 │
╞═════════════════════╪═════════════╪═══════╪═══════╪═══════╡
│ 2020-01-01 13:45:48 ┆ [3] ┆ 3 ┆ 3 ┆ 3 │
│ 2020-01-01 16:42:13 ┆ [3, 7] ┆ 10 ┆ 3 ┆ 7 │
│ 2020-01-01 16:45:09 ┆ [3, 7, 5] ┆ 15 ┆ 3 ┆ 7 │
│ 2020-01-02 18:12:48 ┆ [3, 7, … 9] ┆ 24 ┆ 3 ┆ 9 │
│ 2020-01-03 19:45:32 ┆ [9, 2] ┆ 11 ┆ 2 ┆ 9 │
│ 2020-01-08 23:16:43 ┆ [1] ┆ 1 ┆ 1 ┆ 1 │
└─────────────────────┴─────────────┴───────┴───────┴───────┘
shape: (6, 5)
┌─────────────────────┬─────────────┬───────┬───────┬───────┐
│ dt ┆ a ┆ sum_a ┆ min_a ┆ max_a │
│ --- ┆ --- ┆ --- ┆ --- ┆ --- │
│ datetime[μs] ┆ list[i64] ┆ i64 ┆ i64 ┆ i64 │
╞═════════════════════╪═════════════╪═══════╪═══════╪═══════╡
│ 2020-01-01 13:45:48 ┆ [3] ┆ 3 ┆ 3 ┆ 3 │
│ 2020-01-01 16:42:13 ┆ [3, 7] ┆ 10 ┆ 3 ┆ 7 │
│ 2020-01-01 16:45:09 ┆ [3, 7, 5] ┆ 15 ┆ 3 ┆ 7 │
│ 2020-01-02 18:12:48 ┆ [3, 7, … 9] ┆ 24 ┆ 3 ┆ 9 │
│ 2020-01-03 19:45:32 ┆ [9, 2] ┆ 11 ┆ 2 ┆ 9 │
│ 2020-01-08 23:16:43 ┆ [1] ┆ 1 ┆ 1 ┆ 1 │
└─────────────────────┴─────────────┴───────┴───────┴───────┘
-
offset : 지정된 기간을 이동시킵니다. -period(기본값)입니다. 아래 코드는 dt 컬럼의 데이터를 1일씩 옮기면서 2일 기간 동안의 a 값을 묶은 것입니다.
out = df.rolling(index_column="dt", period="2d", offset='1d').agg(pl.col("a")) print(out)
out = df.rolling(index_column="dt", period="2d", offset='1d').agg(pl.col("a")) print(out)
shape: (6, 2) ┌─────────────────────┬───────────┐ │ dt ┆ a │ │ --- ┆ --- │ │ datetime[μs] ┆ list[i64] │ ╞═════════════════════╪═══════════╡ │ 2020-01-01 13:45:48 ┆ [9, 2] │ │ 2020-01-01 16:42:13 ┆ [9, 2] │ │ 2020-01-01 16:45:09 ┆ [9, 2] │ │ 2020-01-02 18:12:48 ┆ [2] │ │ 2020-01-03 19:45:32 ┆ [] │ │ 2020-01-08 23:16:43 ┆ [] │ └─────────────────────┴───────────┘
shape: (6, 2) ┌─────────────────────┬───────────┐ │ dt ┆ a │ │ --- ┆ --- │ │ datetime[μs] ┆ list[i64] │ ╞═════════════════════╪═══════════╡ │ 2020-01-01 13:45:48 ┆ [9, 2] │ │ 2020-01-01 16:42:13 ┆ [9, 2] │ │ 2020-01-01 16:45:09 ┆ [9, 2] │ │ 2020-01-02 18:12:48 ┆ [2] │ │ 2020-01-03 19:45:32 ┆ [] │ │ 2020-01-08 23:16:43 ┆ [] │ └─────────────────────┴───────────┘
dt period 범위 period 적용 값 offset 범위 offset 적용 값 2020-01-01 13:45:48 [2020-01-01 13:45:48, 2020-01-03 13:45:48] [3,7,5,9] [2020-01-02 13:45:48, 2020-01-04 13:45:48] [9,2] 2020-01-01 16:42:13 [2020-01-01 16:42:13, 2020-01-03 16:42:13] [3,7,5,9] [2020-01-02 16:42:13, 2020-01-04 16:42:13] [9,2] 2020-01-01 16:45:09 [2020-01-01 16:45:09, 2020-01-03 16:45:09] [3,7,5,9] [2020-01-02 16:45:09, 2020-01-04 16:45:09] [9,2] 2020-01-02 18:12:48 [2020-01-02 18:12:48, 2020-01-04 18:12:48] [3,7,5,9] [2020-01-03 18:12:48, 2020-01-05 18:12:48] [2] 2020-01-03 19:45:32 [2020-01-03 19:45:32, 2020-01-05 19:45:32] [3,7,5,9] [2020-01-04 19:45:32, 2020-01-06 19:45:32] [] 2020-01-08 23:16:43 [2020-01-08 23:16:43, 2020-01-10 23:16:43] [3,7,5,9] [2020-01-09 23:16:43, 2020-01-11 23:16:43] [] -
closed : 시간 간격의 어느 쪽을 닫을지 포함 관계를 정의합니다.
dates = [ "2020-01-01 00:00:00", "2020-01-01 16:42:13", "2020-01-01 16:45:09", "2020-01-02 18:12:48", "2020-01-03 00:00:00", "2020-01-08 23:16:43", ] df = pl.DataFrame({"dt": dates, "a": [3, 7, 5, 9, 2, 1]}).with_columns( pl.col("dt").str.strptime(pl.Datetime).set_sorted() ) print(df)
dates = [ "2020-01-01 00:00:00", "2020-01-01 16:42:13", "2020-01-01 16:45:09", "2020-01-02 18:12:48", "2020-01-03 00:00:00", "2020-01-08 23:16:43", ] df = pl.DataFrame({"dt": dates, "a": [3, 7, 5, 9, 2, 1]}).with_columns( pl.col("dt").str.strptime(pl.Datetime).set_sorted() ) print(df)
shape: (6, 2) ┌─────────────────────┬─────┐ │ dt ┆ a │ │ --- ┆ --- │ │ datetime[μs] ┆ i64 │ ╞═════════════════════╪═════╡ │ 2020-01-01 00:00:00 ┆ 3 │ │ 2020-01-01 16:42:13 ┆ 7 │ │ 2020-01-01 16:45:09 ┆ 5 │ │ 2020-01-02 18:12:48 ┆ 9 │ │ 2020-01-03 00:00:00 ┆ 2 │ │ 2020-01-08 23:16:43 ┆ 1 │ └─────────────────────┴─────┘
shape: (6, 2) ┌─────────────────────┬─────┐ │ dt ┆ a │ │ --- ┆ --- │ │ datetime[μs] ┆ i64 │ ╞═════════════════════╪═════╡ │ 2020-01-01 00:00:00 ┆ 3 │ │ 2020-01-01 16:42:13 ┆ 7 │ │ 2020-01-01 16:45:09 ┆ 5 │ │ 2020-01-02 18:12:48 ┆ 9 │ │ 2020-01-03 00:00:00 ┆ 2 │ │ 2020-01-08 23:16:43 ┆ 1 │ └─────────────────────┴─────┘
closed="right"
: 시작 경계값 < dt ≤ 종료 경계값이 됩니다. 각각의 행마다 개별 값에 의해 2일씩 묶어 출력해보도록 하겠습니다. 이때, closed를 right로 설정하였기 때문에 2020-01-03 00:00:00 데이터를 보시면 시작 경계값인 2020-01-01 00:00:00 데이터 3이 들어가지 않는 것을 보실 수 있습니다.out = df.rolling(index_column="dt", period="2d", closed='right').agg(pl.col("a")) print(out)
out = df.rolling(index_column="dt", period="2d", closed='right').agg(pl.col("a")) print(out)
shape: (6, 2) ┌─────────────────────┬─────────────┐ │ dt ┆ a │ │ --- ┆ --- │ │ datetime[μs] ┆ list[i64] │ ╞═════════════════════╪═════════════╡ │ 2020-01-01 00:00:00 ┆ [3] │ │ 2020-01-01 16:42:13 ┆ [3, 7] │ │ 2020-01-01 16:45:09 ┆ [3, 7, 5] │ │ 2020-01-02 18:12:48 ┆ [3, 7, … 9] │ │ 2020-01-03 00:00:00 ┆ [7, 5, … 2] │ │ 2020-01-08 23:16:43 ┆ [1] │ └─────────────────────┴─────────────┘
shape: (6, 2) ┌─────────────────────┬─────────────┐ │ dt ┆ a │ │ --- ┆ --- │ │ datetime[μs] ┆ list[i64] │ ╞═════════════════════╪═════════════╡ │ 2020-01-01 00:00:00 ┆ [3] │ │ 2020-01-01 16:42:13 ┆ [3, 7] │ │ 2020-01-01 16:45:09 ┆ [3, 7, 5] │ │ 2020-01-02 18:12:48 ┆ [3, 7, … 9] │ │ 2020-01-03 00:00:00 ┆ [7, 5, … 2] │ │ 2020-01-08 23:16:43 ┆ [1] │ └─────────────────────┴─────────────┘
closed="left"
(기본값) : 시작 경계값 ≤ time < 종료 경계값이 됩니다. closed를 left로 설정하였기 때문에 2020-01-03 00:00:00 데이터를 보시면 종료 경계값인 2020-01-03 00:00:00 데이터 2가 들어가지 않는 것을 보실 수 있습니다.out = df.rolling(index_column="dt", period="2d", closed='left').agg(pl.col("a")) print(out)
out = df.rolling(index_column="dt", period="2d", closed='left').agg(pl.col("a")) print(out)
shape: (6, 2) ┌─────────────────────┬─────────────┐ │ dt ┆ a │ │ --- ┆ --- │ │ datetime[μs] ┆ list[i64] │ ╞═════════════════════╪═════════════╡ │ 2020-01-01 00:00:00 ┆ [] │ │ 2020-01-01 16:42:13 ┆ [3] │ │ 2020-01-01 16:45:09 ┆ [3, 7] │ │ 2020-01-02 18:12:48 ┆ [3, 7, 5] │ │ 2020-01-03 00:00:00 ┆ [3, 7, … 9] │ │ 2020-01-08 23:16:43 ┆ [] │ └─────────────────────┴─────────────┘
shape: (6, 2) ┌─────────────────────┬─────────────┐ │ dt ┆ a │ │ --- ┆ --- │ │ datetime[μs] ┆ list[i64] │ ╞═════════════════════╪═════════════╡ │ 2020-01-01 00:00:00 ┆ [] │ │ 2020-01-01 16:42:13 ┆ [3] │ │ 2020-01-01 16:45:09 ┆ [3, 7] │ │ 2020-01-02 18:12:48 ┆ [3, 7, 5] │ │ 2020-01-03 00:00:00 ┆ [3, 7, … 9] │ │ 2020-01-08 23:16:43 ┆ [] │ └─────────────────────┴─────────────┘
closed="both"
: 시작 경계값 ≤ time ≤ 종료 경계값이 됩니다. closed를 both로 설정하였기 때문에 2020-01-03 00:00:00 데이터를 보시면 시작 경계값인 2020-01-01 00:00:00 데이터 3이 들어가고, 종료 경계값인 2020-01-03 00:00:00 데이터 2가 들어간 것을 보실 수 있습니다.out = df.rolling(index_column="dt", period="2d", closed='both').agg(pl.col("a")) print(out)
out = df.rolling(index_column="dt", period="2d", closed='both').agg(pl.col("a")) print(out)
shape: (6, 2) ┌─────────────────────┬─────────────┐ │ dt ┆ a │ │ --- ┆ --- │ │ datetime[μs] ┆ list[i64] │ ╞═════════════════════╪═════════════╡ │ 2020-01-01 00:00:00 ┆ [3] │ │ 2020-01-01 16:42:13 ┆ [3, 7] │ │ 2020-01-01 16:45:09 ┆ [3, 7, 5] │ │ 2020-01-02 18:12:48 ┆ [3, 7, … 9] │ │ 2020-01-03 00:00:00 ┆ [3, 7, … 2] │ │ 2020-01-08 23:16:43 ┆ [1] │ └─────────────────────┴─────────────┘
shape: (6, 2) ┌─────────────────────┬─────────────┐ │ dt ┆ a │ │ --- ┆ --- │ │ datetime[μs] ┆ list[i64] │ ╞═════════════════════╪═════════════╡ │ 2020-01-01 00:00:00 ┆ [3] │ │ 2020-01-01 16:42:13 ┆ [3, 7] │ │ 2020-01-01 16:45:09 ┆ [3, 7, 5] │ │ 2020-01-02 18:12:48 ┆ [3, 7, … 9] │ │ 2020-01-03 00:00:00 ┆ [3, 7, … 2] │ │ 2020-01-08 23:16:43 ┆ [1] │ └─────────────────────┴─────────────┘
closed="none"
: 시작 경계값 < time < 종료 경계값이 됩니다. closed를 both로 설정하였기 때문에 2020-01-03 00:00:00 데이터를 보시면 시작 경계값인 2020-01-01 00:00:00 데이터 3과 종료 경계값인 2020-01-03 00:00:00 데이터 2가 들어가지 않은 것을 보실 수 있습니다.out = df.rolling(index_column="dt", period="2d", closed='none').agg(pl.col("a")) print(out)
out = df.rolling(index_column="dt", period="2d", closed='none').agg(pl.col("a")) print(out)
shape: (6, 2) ┌─────────────────────┬───────────┐ │ dt ┆ a │ │ --- ┆ --- │ │ datetime[μs] ┆ list[i64] │ ╞═════════════════════╪═══════════╡ │ 2020-01-01 00:00:00 ┆ [] │ │ 2020-01-01 16:42:13 ┆ [3] │ │ 2020-01-01 16:45:09 ┆ [3, 7] │ │ 2020-01-02 18:12:48 ┆ [3, 7, 5] │ │ 2020-01-03 00:00:00 ┆ [7, 5, 9] │ │ 2020-01-08 23:16:43 ┆ [] │ └─────────────────────┴───────────┘
shape: (6, 2) ┌─────────────────────┬───────────┐ │ dt ┆ a │ │ --- ┆ --- │ │ datetime[μs] ┆ list[i64] │ ╞═════════════════════╪═══════════╡ │ 2020-01-01 00:00:00 ┆ [] │ │ 2020-01-01 16:42:13 ┆ [3] │ │ 2020-01-01 16:45:09 ┆ [3, 7] │ │ 2020-01-02 18:12:48 ┆ [3, 7, 5] │ │ 2020-01-03 00:00:00 ┆ [7, 5, 9] │ │ 2020-01-08 23:16:43 ┆ [] │ └─────────────────────┴───────────┘
-
group_by : 다른 컬럼 그룹화와 결합할 수도 있습니다. group_by에 컬럼명을 입력하여 어떤 열을 기준으로 그룹별로 집계할지 정할 수 있습니다. group_by가 전달되면 출력 결과는 그룹화 할 컬럼을 기준으로 각 그룹 내에서 오름차순으로 정렬됩니다. 실습을 위해 컬럼명을 추가해보도록 하겠습니다. 출력 결과를 보시면, groups 컬럼이 추가된 것을 보실 수 있습니다.
df = df.with_columns( pl.Series("groups", ['a', 'b', 'a', 'b', 'a', 'b']) ) print(df)
df = df.with_columns( pl.Series("groups", ['a', 'b', 'a', 'b', 'a', 'b']) ) print(df)
shape: (6, 3) ┌─────────────────────┬─────┬────────┐ │ dt ┆ a ┆ groups │ │ --- ┆ --- ┆ --- │ │ datetime[μs] ┆ i64 ┆ str │ ╞═════════════════════╪═════╪════════╡ │ 2020-01-01 00:00:00 ┆ 3 ┆ a │ │ 2020-01-01 16:42:13 ┆ 7 ┆ b │ │ 2020-01-01 16:45:09 ┆ 5 ┆ a │ │ 2020-01-02 18:12:48 ┆ 9 ┆ b │ │ 2020-01-03 00:00:00 ┆ 2 ┆ a │ │ 2020-01-08 23:16:43 ┆ 1 ┆ b │ └─────────────────────┴─────┴────────┘
shape: (6, 3) ┌─────────────────────┬─────┬────────┐ │ dt ┆ a ┆ groups │ │ --- ┆ --- ┆ --- │ │ datetime[μs] ┆ i64 ┆ str │ ╞═════════════════════╪═════╪════════╡ │ 2020-01-01 00:00:00 ┆ 3 ┆ a │ │ 2020-01-01 16:42:13 ┆ 7 ┆ b │ │ 2020-01-01 16:45:09 ┆ 5 ┆ a │ │ 2020-01-02 18:12:48 ┆ 9 ┆ b │ │ 2020-01-03 00:00:00 ┆ 2 ┆ a │ │ 2020-01-08 23:16:43 ┆ 1 ┆ b │ └─────────────────────┴─────┴────────┘
groups를 기준으로 먼저 그룹화 한 후에 dt을 기준으로 그룹별 각각의 행마다 개별 값에 의해 2일씩 묶어 출력해보도록 하겠습니다.
out = df.rolling(index_column="dt", period="2d", group_by="groups").agg(pl.col("a")) print(out)
out = df.rolling(index_column="dt", period="2d", group_by="groups").agg(pl.col("a")) print(out)
shape: (6, 3) ┌────────┬─────────────────────┬───────────┐ │ groups ┆ dt ┆ a │ │ --- ┆ --- ┆ --- │ │ str ┆ datetime[μs] ┆ list[i64] │ ╞════════╪═════════════════════╪═══════════╡ │ a ┆ 2020-01-01 00:00:00 ┆ [3] │ │ a ┆ 2020-01-01 16:45:09 ┆ [3, 5] │ │ a ┆ 2020-01-03 00:00:00 ┆ [5, 2] │ │ b ┆ 2020-01-01 16:42:13 ┆ [7] │ │ b ┆ 2020-01-02 18:12:48 ┆ [7, 9] │ │ b ┆ 2020-01-08 23:16:43 ┆ [1] │ └────────┴─────────────────────┴───────────┘
shape: (6, 3) ┌────────┬─────────────────────┬───────────┐ │ groups ┆ dt ┆ a │ │ --- ┆ --- ┆ --- │ │ str ┆ datetime[μs] ┆ list[i64] │ ╞════════╪═════════════════════╪═══════════╡ │ a ┆ 2020-01-01 00:00:00 ┆ [3] │ │ a ┆ 2020-01-01 16:45:09 ┆ [3, 5] │ │ a ┆ 2020-01-03 00:00:00 ┆ [5, 2] │ │ b ┆ 2020-01-01 16:42:13 ┆ [7] │ │ b ┆ 2020-01-02 18:12:48 ┆ [7, 9] │ │ b ┆ 2020-01-08 23:16:43 ┆ [1] │ └────────┴─────────────────────┴───────────┘
period 또는 offset에 인덱스 카운트를 사용하는 경우 index_column에 있는 값을 기준으로 합니다. 각각의 행마다 개별 값에 의해 인덱스 3씩 묶어 출력해보도록 하겠습니다. 만약, 인덱스 수를 행 번호를 기준으로 하려면 rolling과 with_row_index()를 결합하는 것이 좋습니다.
df = pl.DataFrame({"int": [0, 4, 5, 6, 8], "value": [1, 4, 2, 4, 1]})
print(df.rolling("int", period="3i").agg(pl.col("int").alias("aggregated")))
df = pl.DataFrame({"int": [0, 4, 5, 6, 8], "value": [1, 4, 2, 4, 1]})
print(df.rolling("int", period="3i").agg(pl.col("int").alias("aggregated")))
shape: (5, 2)
┌─────┬────────────┐
│ int ┆ aggregated │
│ --- ┆ --- │
│ i64 ┆ list[i64] │
╞═════╪════════════╡
│ 0 ┆ [0] │
│ 4 ┆ [4] │
│ 5 ┆ [4, 5] │
│ 6 ┆ [4, 5, 6] │
│ 8 ┆ [6, 8] │
└─────┴────────────┘
shape: (5, 2)
┌─────┬────────────┐
│ int ┆ aggregated │
│ --- ┆ --- │
│ i64 ┆ list[i64] │
╞═════╪════════════╡
│ 0 ┆ [0] │
│ 4 ┆ [4] │
│ 5 ┆ [4, 5] │
│ 6 ┆ [4, 5, 6] │
│ 8 ┆ [6, 8] │
└─────┴────────────┘
인덱스에 대한 rolling의 경우, 데이터 타입은 UInt32, UInt64, Int32, Int64 중 하나이어야 합니다. 처음에 일시적으로 Int64로 형변환되므로 성능이 중요한 경우 Int64로 사용해주시길 바랍니다.
2.5 partition_by
partition_by 메서드는 지정된 열을 기준으로 그룹화하고 여러 데이터프레임으로 분할하여 그룹별로 반환합니다. 데이터프레임을 생성하고 a열을 기준으로 그룹화 한 후에 그룹별로 데이터프레임을 생성해보도록 하겠습니다.
df = pl.DataFrame(
{
"a": ["a", "b", "a", "b", "c"],
"b": [1, 2, 1, 3, 3],
"c": [5, 4, 3, 2, 1],
}
)
print(df.partition_by("a"))
df = pl.DataFrame(
{
"a": ["a", "b", "a", "b", "c"],
"b": [1, 2, 1, 3, 3],
"c": [5, 4, 3, 2, 1],
}
)
print(df.partition_by("a"))
- by : 그룹화할 열로 해당 열을 기준으로 파티션에 단일 열 이름을 전달합니다.
[shape: (2, 3)
┌─────┬─────┬─────┐
│ a ┆ b ┆ c │
│ --- ┆ --- ┆ --- │
│ str ┆ i64 ┆ i64 │
╞═════╪═════╪═════╡
│ a ┆ 1 ┆ 5 │
│ a ┆ 1 ┆ 3 │
└─────┴─────┴─────┘,
shape: (2, 3)
┌─────┬─────┬─────┐
│ a ┆ b ┆ c │
│ --- ┆ --- ┆ --- │
│ str ┆ i64 ┆ i64 │
╞═════╪═════╪═════╡
│ b ┆ 2 ┆ 4 │
│ b ┆ 3 ┆ 2 │
└─────┴─────┴─────┘,
shape: (1, 3)
┌─────┬─────┬─────┐
│ a ┆ b ┆ c │
│ --- ┆ --- ┆ --- │
│ str ┆ i64 ┆ i64 │
╞═════╪═════╪═════╡
│ c ┆ 3 ┆ 1 │
└─────┴─────┴─────┘]
[shape: (2, 3)
┌─────┬─────┬─────┐
│ a ┆ b ┆ c │
│ --- ┆ --- ┆ --- │
│ str ┆ i64 ┆ i64 │
╞═════╪═════╪═════╡
│ a ┆ 1 ┆ 5 │
│ a ┆ 1 ┆ 3 │
└─────┴─────┴─────┘,
shape: (2, 3)
┌─────┬─────┬─────┐
│ a ┆ b ┆ c │
│ --- ┆ --- ┆ --- │
│ str ┆ i64 ┆ i64 │
╞═════╪═════╪═════╡
│ b ┆ 2 ┆ 4 │
│ b ┆ 3 ┆ 2 │
└─────┴─────┴─────┘,
shape: (1, 3)
┌─────┬─────┬─────┐
│ a ┆ b ┆ c │
│ --- ┆ --- ┆ --- │
│ str ┆ i64 ┆ i64 │
╞═════╪═════╪═════╡
│ c ┆ 3 ┆ 1 │
└─────┴─────┴─────┘]
여러 열을 기준으로 그룹화 하실때는 컬럼명을 리스트를 작성하거나 각 컬럼명을 순서대로 작성하여 파티션을 분할합니다. 출력 결과를 보시면 a열과 b열을 기준으로 그룹화하여 그룹별로 데이터프레임을 생성한 것을 보실 수 있습니다.
print(df.partition_by("a", "b"))
print(df.partition_by("a", "b"))
[shape: (2, 3)
┌─────┬─────┬─────┐
│ a ┆ b ┆ c │
│ --- ┆ --- ┆ --- │
│ str ┆ i64 ┆ i64 │
╞═════╪═════╪═════╡
│ a ┆ 1 ┆ 5 │
│ a ┆ 1 ┆ 3 │
└─────┴─────┴─────┘,
shape: (1, 3)
┌─────┬─────┬─────┐
│ a ┆ b ┆ c │
│ --- ┆ --- ┆ --- │
│ str ┆ i64 ┆ i64 │
╞═════╪═════╪═════╡
│ b ┆ 2 ┆ 4 │
└─────┴─────┴─────┘,
shape: (1, 3)
┌─────┬─────┬─────┐
│ a ┆ b ┆ c │
│ --- ┆ --- ┆ --- │
│ str ┆ i64 ┆ i64 │
╞═════╪═════╪═════╡
│ b ┆ 3 ┆ 2 │
└─────┴─────┴─────┘,
shape: (1, 3)
┌─────┬─────┬─────┐
│ a ┆ b ┆ c │
│ --- ┆ --- ┆ --- │
│ str ┆ i64 ┆ i64 │
╞═════╪═════╪═════╡
│ c ┆ 3 ┆ 1 │
└─────┴─────┴─────┘]
[shape: (2, 3)
┌─────┬─────┬─────┐
│ a ┆ b ┆ c │
│ --- ┆ --- ┆ --- │
│ str ┆ i64 ┆ i64 │
╞═════╪═════╪═════╡
│ a ┆ 1 ┆ 5 │
│ a ┆ 1 ┆ 3 │
└─────┴─────┴─────┘,
shape: (1, 3)
┌─────┬─────┬─────┐
│ a ┆ b ┆ c │
│ --- ┆ --- ┆ --- │
│ str ┆ i64 ┆ i64 │
╞═════╪═════╪═════╡
│ b ┆ 2 ┆ 4 │
└─────┴─────┴─────┘,
shape: (1, 3)
┌─────┬─────┬─────┐
│ a ┆ b ┆ c │
│ --- ┆ --- ┆ --- │
│ str ┆ i64 ┆ i64 │
╞═════╪═════╪═════╡
│ b ┆ 3 ┆ 2 │
└─────┴─────┴─────┘,
shape: (1, 3)
┌─────┬─────┬─────┐
│ a ┆ b ┆ c │
│ --- ┆ --- ┆ --- │
│ str ┆ i64 ┆ i64 │
╞═════╪═════╪═════╡
│ c ┆ 3 ┆ 1 │
└─────┴─────┴─────┘]
print(df.partition_by("a", maintain_order=True))
print(df.partition_by("a", maintain_order=True))
- maintain_order : True로 설정하면 그룹의 순서가 데이터프레임 생성 순서와 일치하도록 출력합니다. 그렇게 때문에 True로 설정하시게 되면 기존 연산보다 조금 느립니다.
[shape: (2, 3)
┌─────┬─────┬─────┐
│ a ┆ b ┆ c │
│ --- ┆ --- ┆ --- │
│ str ┆ i64 ┆ i64 │
╞═════╪═════╪═════╡
│ a ┆ 1 ┆ 5 │
│ a ┆ 1 ┆ 3 │
└─────┴─────┴─────┘,
shape: (2, 3)
┌─────┬─────┬─────┐
│ a ┆ b ┆ c │
│ --- ┆ --- ┆ --- │
│ str ┆ i64 ┆ i64 │
╞═════╪═════╪═════╡
│ b ┆ 2 ┆ 4 │
│ b ┆ 3 ┆ 2 │
└─────┴─────┴─────┘,
shape: (1, 3)
┌─────┬─────┬─────┐
│ a ┆ b ┆ c │
│ --- ┆ --- ┆ --- │
│ str ┆ i64 ┆ i64 │
╞═════╪═════╪═════╡
│ c ┆ 3 ┆ 1 │
└─────┴─────┴─────┘]
[shape: (2, 3)
┌─────┬─────┬─────┐
│ a ┆ b ┆ c │
│ --- ┆ --- ┆ --- │
│ str ┆ i64 ┆ i64 │
╞═════╪═════╪═════╡
│ a ┆ 1 ┆ 5 │
│ a ┆ 1 ┆ 3 │
└─────┴─────┴─────┘,
shape: (2, 3)
┌─────┬─────┬─────┐
│ a ┆ b ┆ c │
│ --- ┆ --- ┆ --- │
│ str ┆ i64 ┆ i64 │
╞═════╪═════╪═════╡
│ b ┆ 2 ┆ 4 │
│ b ┆ 3 ┆ 2 │
└─────┴─────┴─────┘,
shape: (1, 3)
┌─────┬─────┬─────┐
│ a ┆ b ┆ c │
│ --- ┆ --- ┆ --- │
│ str ┆ i64 ┆ i64 │
╞═════╪═════╪═════╡
│ c ┆ 3 ┆ 1 │
└─────┴─────┴─────┘]
print(df.partition_by("a", include_key=True))
print(df.partition_by("a", include_key=True))
- include_key : True로 설정하면 데이터프레임을 분할하는데 사용되는 열을 포함합니다.
[shape: (2, 3)
┌─────┬─────┬─────┐
│ a ┆ b ┆ c │
│ --- ┆ --- ┆ --- │
│ str ┆ i64 ┆ i64 │
╞═════╪═════╪═════╡
│ a ┆ 1 ┆ 5 │
│ a ┆ 1 ┆ 3 │
└─────┴─────┴─────┘,
shape: (2, 3)
┌─────┬─────┬─────┐
│ a ┆ b ┆ c │
│ --- ┆ --- ┆ --- │
│ str ┆ i64 ┆ i64 │
╞═════╪═════╪═════╡
│ b ┆ 2 ┆ 4 │
│ b ┆ 3 ┆ 2 │
└─────┴─────┴─────┘,
shape: (1, 3)
┌─────┬─────┬─────┐
│ a ┆ b ┆ c │
│ --- ┆ --- ┆ --- │
│ str ┆ i64 ┆ i64 │
╞═════╪═════╪═════╡
│ c ┆ 3 ┆ 1 │
└─────┴─────┴─────┘]
[shape: (2, 3)
┌─────┬─────┬─────┐
│ a ┆ b ┆ c │
│ --- ┆ --- ┆ --- │
│ str ┆ i64 ┆ i64 │
╞═════╪═════╪═════╡
│ a ┆ 1 ┆ 5 │
│ a ┆ 1 ┆ 3 │
└─────┴─────┴─────┘,
shape: (2, 3)
┌─────┬─────┬─────┐
│ a ┆ b ┆ c │
│ --- ┆ --- ┆ --- │
│ str ┆ i64 ┆ i64 │
╞═════╪═════╪═════╡
│ b ┆ 2 ┆ 4 │
│ b ┆ 3 ┆ 2 │
└─────┴─────┴─────┘,
shape: (1, 3)
┌─────┬─────┬─────┐
│ a ┆ b ┆ c │
│ --- ┆ --- ┆ --- │
│ str ┆ i64 ┆ i64 │
╞═════╪═════╪═════╡
│ c ┆ 3 ┆ 1 │
└─────┴─────┴─────┘]
False로 설정하시면 데이터프레임을 분할하는데 사용되는 열을 포함하지 않습니다.
print(df.partition_by("a", include_key=False))
print(df.partition_by("a", include_key=False))
[shape: (2, 2)
┌─────┬─────┐
│ b ┆ c │
│ --- ┆ --- │
│ i64 ┆ i64 │
╞═════╪═════╡
│ 1 ┆ 5 │
│ 1 ┆ 3 │
└─────┴─────┘,
shape: (2, 2)
┌─────┬─────┐
│ b ┆ c │
│ --- ┆ --- │
│ i64 ┆ i64 │
╞═════╪═════╡
│ 2 ┆ 4 │
│ 3 ┆ 2 │
└─────┴─────┘,
shape: (1, 2)
┌─────┬─────┐
│ b ┆ c │
│ --- ┆ --- │
│ i64 ┆ i64 │
╞═════╪═════╡
│ 3 ┆ 1 │
└─────┴─────┘]
[shape: (2, 2)
┌─────┬─────┐
│ b ┆ c │
│ --- ┆ --- │
│ i64 ┆ i64 │
╞═════╪═════╡
│ 1 ┆ 5 │
│ 1 ┆ 3 │
└─────┴─────┘,
shape: (2, 2)
┌─────┬─────┐
│ b ┆ c │
│ --- ┆ --- │
│ i64 ┆ i64 │
╞═════╪═════╡
│ 2 ┆ 4 │
│ 3 ┆ 2 │
└─────┴─────┘,
shape: (1, 2)
┌─────┬─────┐
│ b ┆ c │
│ --- ┆ --- │
│ i64 ┆ i64 │
╞═════╪═════╡
│ 3 ┆ 1 │
└─────┴─────┘]
import polars.selectors as cs
df.partition_by(cs.string(), as_dict=True)
import polars.selectors as cs
df.partition_by(cs.string(), as_dict=True)
- as_dict : True로 설정하여 파티션을 리스트 대신 딕셔너리로 반환합니다. 딕셔너리 키는 각 그룹을 식별하는 고유한 그룹 값의 튜플입니다.
{('a',): shape: (2, 3)
┌─────┬─────┬─────┐
│ a ┆ b ┆ c │
│ --- ┆ --- ┆ --- │
│ str ┆ i64 ┆ i64 │
╞═════╪═════╪═════╡
│ a ┆ 1 ┆ 5 │
│ a ┆ 1 ┆ 3 │
└─────┴─────┴─────┘,
('b',): shape: (2, 3)
┌─────┬─────┬─────┐
│ a ┆ b ┆ c │
│ --- ┆ --- ┆ --- │
│ str ┆ i64 ┆ i64 │
╞═════╪═════╪═════╡
│ b ┆ 2 ┆ 4 │
│ b ┆ 3 ┆ 2 │
└─────┴─────┴─────┘,
('c',): shape: (1, 3)
┌─────┬─────┬─────┐
│ a ┆ b ┆ c │
│ --- ┆ --- ┆ --- │
│ str ┆ i64 ┆ i64 │
╞═════╪═════╪═════╡
│ c ┆ 3 ┆ 1 │
└─────┴─────┴─────┘}
{('a',): shape: (2, 3)
┌─────┬─────┬─────┐
│ a ┆ b ┆ c │
│ --- ┆ --- ┆ --- │
│ str ┆ i64 ┆ i64 │
╞═════╪═════╪═════╡
│ a ┆ 1 ┆ 5 │
│ a ┆ 1 ┆ 3 │
└─────┴─────┴─────┘,
('b',): shape: (2, 3)
┌─────┬─────┬─────┐
│ a ┆ b ┆ c │
│ --- ┆ --- ┆ --- │
│ str ┆ i64 ┆ i64 │
╞═════╪═════╪═════╡
│ b ┆ 2 ┆ 4 │
│ b ┆ 3 ┆ 2 │
└─────┴─────┴─────┘,
('c',): shape: (1, 3)
┌─────┬─────┬─────┐
│ a ┆ b ┆ c │
│ --- ┆ --- ┆ --- │
│ str ┆ i64 ┆ i64 │
╞═════╪═════╪═════╡
│ c ┆ 3 ┆ 1 │
└─────┴─────┴─────┘}
3. 피벗 테이블
3.1 pivot
pivot 메서드는 데이터프레임으로 스프레드시트 스타일의 피벗 테이블을 만듭니다. 세로로 긴 형식에서 넓은 형식으로 데이터프레임을 재구성할 수 있습니다. 이 메서드는 Eager 모드에서만 사용 가능하며, 고유한 열 값을 미리 알고 있는 경우 lazy pivot을 수행합니다.
각 행이 서로 다른 시험을 나타내는 일부 학생의 시험 점수에 대한 데이터프레임이 있다고 가정해 보겠습니다.
df = pl.DataFrame({
"name": ["Cady", "Cady", "Karen", "Karen"],
"subject": ["maths", "physics", "maths", "physics"],
"test_1": [98, 99, 61, 58],
"test_2": [100, 100, 60, 60],
})
print(df)
df = pl.DataFrame({
"name": ["Cady", "Cady", "Karen", "Karen"],
"subject": ["maths", "physics", "maths", "physics"],
"test_1": [98, 99, 61, 58],
"test_2": [100, 100, 60, 60],
})
print(df)
shape: (4, 4)
┌───────┬─────────┬────────┬────────┐
│ name ┆ subject ┆ test_1 ┆ test_2 │
│ --- ┆ --- ┆ --- ┆ --- │
│ str ┆ str ┆ i64 ┆ i64 │
╞═══════╪═════════╪════════╪════════╡
│ Cady ┆ maths ┆ 98 ┆ 100 │
│ Cady ┆ physics ┆ 99 ┆ 100 │
│ Karen ┆ maths ┆ 61 ┆ 60 │
│ Karen ┆ physics ┆ 58 ┆ 60 │
└───────┴─────────┴────────┴────────┘
shape: (4, 4)
┌───────┬─────────┬────────┬────────┐
│ name ┆ subject ┆ test_1 ┆ test_2 │
│ --- ┆ --- ┆ --- ┆ --- │
│ str ┆ str ┆ i64 ┆ i64 │
╞═══════╪═════════╪════════╪════════╡
│ Cady ┆ maths ┆ 98 ┆ 100 │
│ Cady ┆ physics ┆ 99 ┆ 100 │
│ Karen ┆ maths ┆ 61 ┆ 60 │
│ Karen ┆ physics ┆ 58 ┆ 60 │
└───────┴─────────┴────────┴────────┘
학생 한 명당 한 행을 갖고, 열에는 다른 과목을, 값은 test_1 점수로 구성해보도록 하겠습니다.
print(df.pivot("subject", index="name", values="test_1"))
print(df.pivot("subject", index="name", values="test_1"))
- on : 반환될 데이터프레임의 새 열로 값이 사용될 열입니다.
- index : 반환될 데이터프레임에는 인덱스 값의 고유한 값으로 구성합니다. None으로 설정할 경우, on이나 값에 지정되지 않은 나머지 모든 열이 사용됩니다. 그러므로 index나 values 중 하나 이상의 매개변수를 지정하셔야 합니다.
- values : 인덱스에서 새 열 아래로 이동될 값의 기존 열이며, 집계를 지정한 경우 집계가 계산될 값입니다. None으로 설정할 경우, on 및 index에 지정되지 않은 나머지 모든 열이 사용됩니다. 그러므로 index나 values 중 하나 이상의 매개변수를 지정하셔야 합니다.
shape: (2, 3)
┌───────┬───────┬─────────┐
│ name ┆ maths ┆ physics │
│ --- ┆ --- ┆ --- │
│ str ┆ i64 ┆ i64 │
╞═══════╪═══════╪═════════╡
│ Cady ┆ 98 ┆ 99 │
│ Karen ┆ 61 ┆ 58 │
└───────┴───────┴─────────┘
shape: (2, 3)
┌───────┬───────┬─────────┐
│ name ┆ maths ┆ physics │
│ --- ┆ --- ┆ --- │
│ str ┆ i64 ┆ i64 │
╞═══════╪═══════╪═════════╡
│ Cady ┆ 98 ┆ 99 │
│ Karen ┆ 61 ┆ 58 │
└───────┴───────┴─────────┘
Polars 셀럭터를 사용하여 피벗 테이블에 모든 테스트 점수를 포함해보도록 하겠습니다. 출력해보시면 name 행에는 고유한 이름이 들어간 것을 보실 수 있으며, 과목별 점수가 컬럼명으로 들어가 있고, 값에는 그에 맞는 값이 들어간 것을 보실 수 있습니다.
import polars.selectors as cs
print(df.pivot("subject", values=cs.starts_with("test")))
import polars.selectors as cs
print(df.pivot("subject", values=cs.starts_with("test")))
- cs.starts_with("텍스트"): 텍스트로 시작하는 값을 반환합니다.
shape: (2, 5)
┌───────┬──────────────┬────────────────┬──────────────┬────────────────┐
│ name ┆ test_1_maths ┆ test_1_physics ┆ test_2_maths ┆ test_2_physics │
│ --- ┆ --- ┆ --- ┆ --- ┆ --- │
│ str ┆ i64 ┆ i64 ┆ i64 ┆ i64 │
╞═══════╪══════════════╪════════════════╪══════════════╪════════════════╡
│ Cady ┆ 98 ┆ 99 ┆ 100 ┆ 100 │
│ Karen ┆ 61 ┆ 58 ┆ 60 ┆ 60 │
└───────┴──────────────┴────────────────┴──────────────┴────────────────┘
shape: (2, 5)
┌───────┬──────────────┬────────────────┬──────────────┬────────────────┐
│ name ┆ test_1_maths ┆ test_1_physics ┆ test_2_maths ┆ test_2_physics │
│ --- ┆ --- ┆ --- ┆ --- ┆ --- │
│ str ┆ i64 ┆ i64 ┆ i64 ┆ i64 │
╞═══════╪══════════════╪════════════════╪══════════════╪════════════════╡
│ Cady ┆ 98 ┆ 99 ┆ 100 ┆ 100 │
│ Karen ┆ 61 ┆ 58 ┆ 60 ┆ 60 │
└───────┴──────────────┴────────────────┴──────────────┴────────────────┘
셀당 여러 값이 있는 경우 aggregate_function
매개변수를 사용하여 값을 집계할 수 있습니다. 출력 결과를 보시면 인덱스에는 ix 컬럼의 고유값이 col과 남은 컬럼을 기준으로 합계를 계산한 것을 보실 수 있습니다.
df = pl.DataFrame({
"ix": [1, 1, 2, 2, 1, 2],
"col": ["a", "a", "a", "a", "b", "b"],
"foo": [0, 1, 2, 2, 7, 1],
"bar": [0, 2, 0, 0, 9, 4],
})
print(df.pivot("col", index="ix", aggregate_function="sum"))
df = pl.DataFrame({
"ix": [1, 1, 2, 2, 1, 2],
"col": ["a", "a", "a", "a", "b", "b"],
"foo": [0, 1, 2, 2, 7, 1],
"bar": [0, 2, 0, 0, 9, 4],
})
print(df.pivot("col", index="ix", aggregate_function="sum"))
- aggregate_function
- 미리 정의된 집계 함수(min, max, first, last, sum, mean, median, len) 중 하나를 선택합니다.
- 집계를 수행하는 표현식을 작성합니다.
- None : 집계가 수행되지 않으며, 여러 값이 그룹에 있으면 오류가 발생합니다.
shape: (2, 5)
┌─────┬───────┬───────┬───────┬───────┐
│ ix ┆ foo_a ┆ foo_b ┆ bar_a ┆ bar_b │
│ --- ┆ --- ┆ --- ┆ --- ┆ --- │
│ i64 ┆ i64 ┆ i64 ┆ i64 ┆ i64 │
╞═════╪═══════╪═══════╪═══════╪═══════╡
│ 1 ┆ 1 ┆ 7 ┆ 2 ┆ 9 │
│ 2 ┆ 4 ┆ 1 ┆ 0 ┆ 4 │
└─────┴───────┴───────┴───────┴───────┘
shape: (2, 5)
┌─────┬───────┬───────┬───────┬───────┐
│ ix ┆ foo_a ┆ foo_b ┆ bar_a ┆ bar_b │
│ --- ┆ --- ┆ --- ┆ --- ┆ --- │
│ i64 ┆ i64 ┆ i64 ┆ i64 ┆ i64 │
╞═════╪═══════╪═══════╪═══════╪═══════╡
│ 1 ┆ 1 ┆ 7 ┆ 2 ┆ 9 │
│ 2 ┆ 4 ┆ 1 ┆ 0 ┆ 4 │
└─────┴───────┴───────┴───────┴───────┘
polars.element()
을 사용하여 사용자 지정 집계 함수를 수행해보도록 하겠습니다. index에는 col1, column에는 col2를 넣고 값에는 각각의 값에 맞는 col3의 평균을 계산해보도록 하겠습니다.
df = pl.DataFrame({
"col1": ["a", "a", "a", "b", "b", "b"],
"col2": ["x", "x", "x", "x", "y", "y"],
"col3": [6, 7, 3, 2, 5, 7],
})
print(df.pivot("col2",index="col1",values="col3",aggregate_function=pl.element().mean(),))
df = pl.DataFrame({
"col1": ["a", "a", "a", "b", "b", "b"],
"col2": ["x", "x", "x", "x", "y", "y"],
"col3": [6, 7, 3, 2, 5, 7],
})
print(df.pivot("col2",index="col1",values="col3",aggregate_function=pl.element().mean(),))
- pl.element() : 특정 위치의 요소를 가져옵니다.
shape: (2, 3)
┌──────┬──────────┬──────────┐
│ col1 ┆ x ┆ y │
│ --- ┆ --- ┆ --- │
│ str ┆ f64 ┆ f64 │
╞══════╪══════════╪══════════╡
│ a ┆ 0.998347 ┆ null │
│ b ┆ 0.964028 ┆ 0.999954 │
└──────┴──────────┴──────────┘
shape: (2, 3)
┌──────┬──────────┬──────────┐
│ col1 ┆ x ┆ y │
│ --- ┆ --- ┆ --- │
│ str ┆ f64 ┆ f64 │
╞══════╪══════════╪══════════╡
│ a ┆ 0.998347 ┆ null │
│ b ┆ 0.964028 ┆ 0.999954 │
└──────┴──────────┴──────────┘
고유한 열 값을 미리 알고 있는 경우, Lazy 모드에서 polars.LazyFrame.group_by()
를 사용하여 위와 동일한 결과를 얻을 수 있습니다.
index = pl.col("col1")
on = pl.col("col2")
values = pl.col("col3")
unique_column_values = ["x", "y"]
aggregate_function = lambda col: col.mean()
print(df.lazy().group_by(index).agg(
aggregate_function(values.filter(on == value)).alias(value)
for value in unique_column_values
).collect())
index = pl.col("col1")
on = pl.col("col2")
values = pl.col("col3")
unique_column_values = ["x", "y"]
aggregate_function = lambda col: col.mean()
print(df.lazy().group_by(index).agg(
aggregate_function(values.filter(on == value)).alias(value)
for value in unique_column_values
).collect())
- collect() : collect를 호출하기 전까지는 실행할 연산들을 계획으로만 세워두고 collect를 호출하실 때 지연 실행된 연산을 실제로 수행하고 결과를 반환합니다.
shape: (2, 3)
┌──────┬──────────┬──────────┐
│ col1 ┆ x ┆ y │
│ --- ┆ --- ┆ --- │
│ str ┆ f64 ┆ f64 │
╞══════╪══════════╪══════════╡
│ a ┆ 0.998347 ┆ null │
│ b ┆ 0.964028 ┆ 0.999954 │
└──────┴──────────┴──────────┘
shape: (2, 3)
┌──────┬──────────┬──────────┐
│ col1 ┆ x ┆ y │
│ --- ┆ --- ┆ --- │
│ str ┆ f64 ┆ f64 │
╞══════╪══════════╪══════════╡
│ a ┆ 0.998347 ┆ null │
│ b ┆ 0.964028 ┆ 0.999954 │
└──────┴──────────┴──────────┘
lazy().group_by()를 사용하는 이유?
- 여러 연산을 최적화 시킬 수 있습니다.
- 메모리를 효율적으로 사용할 수 있습니다.
- 대용량 데이터에서 효과적으로 사용할 수 있습니다.
3.2 unpivot
unpivot 메서드는 데이터프레임을 넓은 형식에서 긴 형식으로 피벗을 해제합니다. 이때, 선택적으로 어떤 열들은 변형하지 않고 그대로 유지하며, 나머지 열들만 피벗 해제할 수 있습니다.
인덱스를 a로 설정하고 나머지 값들은 피벗 해제해보도록 하겠습니다.
df = pl.DataFrame({
"a": ["x", "y", "z"],
"b": [1, 3, 5],
"c": [2, 4, 6],
})
print(df.unpivot(cs.numeric(), index="a"))
df = pl.DataFrame({
"a": ["x", "y", "z"],
"b": [1, 3, 5],
"c": [2, 4, 6],
})
print(df.unpivot(cs.numeric(), index="a"))
- on : 값 변수로 사용할 열입니다. on 비어 있으면 index에 없는 모든 열이 사용됩니다.
- index : 인덱스로 사용할 열로 데이터프레임에 그대로 유지됩니다.
shape: (6, 3)
┌─────┬──────────┬───────┐
│ a ┆ variable ┆ value │
│ --- ┆ --- ┆ --- │
│ str ┆ str ┆ i64 │
╞═════╪══════════╪═══════╡
│ x ┆ b ┆ 1 │
│ y ┆ b ┆ 3 │
│ z ┆ b ┆ 5 │
│ x ┆ c ┆ 2 │
│ y ┆ c ┆ 4 │
│ z ┆ c ┆ 6 │
└─────┴──────────┴───────┘
shape: (6, 3)
┌─────┬──────────┬───────┐
│ a ┆ variable ┆ value │
│ --- ┆ --- ┆ --- │
│ str ┆ str ┆ i64 │
╞═════╪══════════╪═══════╡
│ x ┆ b ┆ 1 │
│ y ┆ b ┆ 3 │
│ z ┆ b ┆ 5 │
│ x ┆ c ┆ 2 │
│ y ┆ c ┆ 4 │
│ z ┆ c ┆ 6 │
└─────┴──────────┴───────┘
위의 코드 출력 결과를 보시면, 원래 컬럼명들은 variable 열에 값들은 value 열에 들어간 것을 보실 수 있습니다. 만약, variable 열 이름과 value 열 이름을 변경하고 싶으시다면 variable_name, value_name을 설정하여 변경하실 수 있습니다.
print(df.unpivot(cs.numeric(), index="a", variable_name='string', value_name='num'))
print(df.unpivot(cs.numeric(), index="a", variable_name='string', value_name='num'))
- variable_name : variable 열에 지정할 이름입니다. 기본값은 "variable"입니다.
- value_name : value 열에 지정할 이름입니다. 기본값은 "value"입니다.
shape: (6, 3)
┌─────┬──────────┬───────┐
│ a ┆ variable ┆ value │
│ --- ┆ --- ┆ --- │
│ str ┆ str ┆ i64 │
╞═════╪══════════╪═══════╡
│ x ┆ b ┆ 1 │
│ y ┆ b ┆ 3 │
│ z ┆ b ┆ 5 │
│ x ┆ c ┆ 2 │
│ y ┆ c ┆ 4 │
│ z ┆ c ┆ 6 │
└─────┴──────────┴───────┘
shape: (6, 3)
┌─────┬──────────┬───────┐
│ a ┆ variable ┆ value │
│ --- ┆ --- ┆ --- │
│ str ┆ str ┆ i64 │
╞═════╪══════════╪═══════╡
│ x ┆ b ┆ 1 │
│ y ┆ b ┆ 3 │
│ z ┆ b ┆ 5 │
│ x ┆ c ┆ 2 │
│ y ┆ c ┆ 4 │
│ z ┆ c ┆ 6 │
└─────┴──────────┴───────┘
unpivot은 pandas의 melt와 유사하지만 인덱스가 id_vars를 대체하고 컬럼이 value_vars를 대체한다는 점이 다릅니다. R에서는 unpivot을 pivot_longer 메서드로 사용하실 수 있습니다.
# Pandas
df.melt(id_vars=['a'], value_vars=['b','c'])
# Pandas
df.melt(id_vars=['a'], value_vars=['b','c'])
# Polars
df.unpivot(cs.numeric(), index="a")
# Polars
df.unpivot(cs.numeric(), index="a")
4. 사용자 정의 함수
4.1 map_rows
map_rows 메서드는 각 행의 값을 튜플로 받아 사용자 정의 함수(또는 람다 함수)에 적용하여 데이터프레임으로 반환합니다. foo에 2를 곱하고 bar에 3을 곱한 값을 람다 함수로 만들고 적용시켜보도록 하겠습니다.
df = pl.DataFrame({"foo": [1, 2, 3], "bar": [-1, 5, 8]})
print(df.map_rows(lambda t: (t[0] * 2, t[1] * 3)))
df = pl.DataFrame({"foo": [1, 2, 3], "bar": [-1, 5, 8]})
print(df.map_rows(lambda t: (t[0] * 2, t[1] * 3)))
shape: (3, 2)
┌──────────┬──────────┐
│ column_0 ┆ column_1 │
│ --- ┆ --- │
│ i64 ┆ i64 │
╞══════════╪══════════╡
│ 2 ┆ -3 │
│ 4 ┆ 15 │
│ 6 ┆ 24 │
└──────────┴──────────┘
shape: (3, 2)
┌──────────┬──────────┐
│ column_0 ┆ column_1 │
│ --- ┆ --- │
│ i64 ┆ i64 │
╞══════════╪══════════╡
│ 2 ┆ -3 │
│ 4 ┆ 15 │
│ 6 ┆ 24 │
└──────────┴──────────┘
이번에는 사용자 정의 함수 적용 후, 반환되는 열의 데이터 타입 지정 방법에 대해 알아보도록 하겠습니다.
result = df.map_rows(
# row를 반환하는 경우
lambda t: t, # 각 행을 그대로 반환
inference_size=1 # 첫 번째 행으로 데이터 타입 추론
)
print(result)
# 또는 행의 일부를 선택해서 반환
result = df.map_rows(
lambda t: (t[0], t[1]), # 원하는 열만 선택
inference_size=1
)
print(result)
result = df.map_rows(
# row를 반환하는 경우
lambda t: t, # 각 행을 그대로 반환
inference_size=1 # 첫 번째 행으로 데이터 타입 추론
)
print(result)
# 또는 행의 일부를 선택해서 반환
result = df.map_rows(
lambda t: (t[0], t[1]), # 원하는 열만 선택
inference_size=1
)
print(result)
- inference_size : 사용자 정의 함수가 행을 반환하는 경우에만 사용되며,
n
번째 행을 사용하여 출력 스키마를 결정합니다.
shape: (3, 2)
┌──────────┬──────────┐
│ column_0 ┆ column_1 │
│ --- ┆ --- │
│ i64 ┆ i64 │
╞══════════╪══════════╡
│ 1 ┆ -1 │
│ 2 ┆ 5 │
│ 3 ┆ 8 │
└──────────┴──────────┘
shape: (3, 2)
┌──────────┬──────────┐
│ column_0 ┆ column_1 │
│ --- ┆ --- │
│ i64 ┆ i64 │
╞══════════╪══════════╡
│ 1 ┆ -1 │
│ 2 ┆ 5 │
│ 3 ┆ 8 │
└──────────┴──────────┘
shape: (3, 2)
┌──────────┬──────────┐
│ column_0 ┆ column_1 │
│ --- ┆ --- │
│ i64 ┆ i64 │
╞══════════╪══════════╡
│ 1 ┆ -1 │
│ 2 ┆ 5 │
│ 3 ┆ 8 │
└──────────┴──────────┘
shape: (3, 2)
┌──────────┬──────────┐
│ column_0 ┆ column_1 │
│ --- ┆ --- │
│ i64 ┆ i64 │
╞══════════╪══════════╡
│ 1 ┆ -1 │
│ 2 ┆ 5 │
│ 3 ┆ 8 │
└──────────┴──────────┘
result = df.map_rows(
lambda t: (t[0]+t[1]), # 원하는 열만 선택
return_dtype=pl.Float64
)
print(result)
result = df.map_rows(
lambda t: (t[0]+t[1]), # 원하는 열만 선택
return_dtype=pl.Float64
)
print(result)
- return_dtype : 반환 데이터 타입으로 따로 설정하지 않으면 Polars가 데이터 타입을 추론합니다.
shape: (3, 1)
┌───────┐
│ map │
│ --- │
│ i64 │
╞═══════╡
│ 0.0 │
│ 7.0 │
│ 11.0 │
└───────┘
shape: (3, 1)
┌───────┐
│ map │
│ --- │
│ i64 │
╞═══════╡
│ 0.0 │
│ 7.0 │
│ 11.0 │
└───────┘
map_rows 사용 시 고려사항
-
열 이름 추적 문제
map_rows
는 데이터프레임 수준에서 열 이름을 추적할 수 없으며, 이는 사용자 정의 함수가 임의로 열을 삭제, 재배열, 변환 또는 추가할 수 있는 블랙박스이기 때문입니다. 그렇기 때문에 열 이름이 유지되는 방식으로 사용자 정의 함수를 적용하려면map_elements
표현식 구문을 대신 사용해야 합니다.name 열의 값은 모두 대문자로 변경하고 age 열의 값은 1을 더하는 사용자 정의 함수를 적용시켜보도록 하겠습니다.
- map_rows 사용 예시 (열 이름 손실)
df = pl.DataFrame({ "name": ["John", "Jane"], "age": [25, 30] }) # map_rows 사용 - 열 이름이 유지되지 않음 result1 = df.map_rows(lambda row: (row[0].upper(), row[1] + 1)) print(result1)
df = pl.DataFrame({ "name": ["John", "Jane"], "age": [25, 30] }) # map_rows 사용 - 열 이름이 유지되지 않음 result1 = df.map_rows(lambda row: (row[0].upper(), row[1] + 1)) print(result1)
column_0, column_1과 같은 기본 이름으로 출력됩니다.
shape: (2, 2) ┌──────────┬──────────┐ │ column_0 ┆ column_1 │ │ --- ┆ --- │ │ str ┆ i64 │ ╞══════════╪══════════╡ │ JOHN ┆ 26 │ │ JANE ┆ 31 │ └──────────┴──────────┘
shape: (2, 2) ┌──────────┬──────────┐ │ column_0 ┆ column_1 │ │ --- ┆ --- │ │ str ┆ i64 │ ╞══════════╪══════════╡ │ JOHN ┆ 26 │ │ JANE ┆ 31 │ └──────────┴──────────┘
- 표현식 수준 구문 사용 (권장 방법)
# 표현식 사용 - 열 이름 유지 result2 = df.select([ pl.col("name").str.to_uppercase(), pl.col("age") + 1 ]) print(result2)
# 표현식 사용 - 열 이름 유지 result2 = df.select([ pl.col("name").str.to_uppercase(), pl.col("age") + 1 ]) print(result2)
name, age 열 이름이 유지됩니다.
shape: (2, 2) ┌──────┬─────┐ │ name ┆ age │ │ --- ┆ --- │ │ str ┆ i64 │ ╞══════╪═════╡ │ JOHN ┆ 26 │ │ JANE ┆ 31 │ └──────┴─────┘
shape: (2, 2) ┌──────┬─────┐ │ name ┆ age │ │ --- ┆ --- │ │ str ┆ i64 │ ╞══════╪═════╡ │ JOHN ┆ 26 │ │ JANE ┆ 31 │ └──────┴─────┘
-
성능 최적화 - @lru_cache 사용
중복된 값에 대한 연산이나 비용이 많이 드는 계산이나 반복될 경우,
@lru_cache
데코레이터를 사용하여 성능을 개선할 수 있습니다.from functools import lru_cache @lru_cache # 캐시 데코레이터 적용 def expensive_calculation(value): result = 0 for i in range(1000): # 복잡한 계산 예시 result += value * i return result df = pl.DataFrame({ "value": [1, 2, 1, 2, 1, 2] # 중복된 값들 }) # 중복 값에 대한 계산을 재사용 result = df.map_rows(lambda row: (row[0], expensive_calculation(row[0]))) print(result)
from functools import lru_cache @lru_cache # 캐시 데코레이터 적용 def expensive_calculation(value): result = 0 for i in range(1000): # 복잡한 계산 예시 result += value * i return result df = pl.DataFrame({ "value": [1, 2, 1, 2, 1, 2] # 중복된 값들 }) # 중복 값에 대한 계산을 재사용 result = df.map_rows(lambda row: (row[0], expensive_calculation(row[0]))) print(result)
shape: (6, 2) ┌──────────┬──────────┐ │ column_0 ┆ column_1 │ │ --- ┆ --- │ │ i64 ┆ i64 │ ╞══════════╪══════════╡ │ 1 ┆ 499500 │ │ 2 ┆ 999000 │ │ 1 ┆ 499500 │ │ 2 ┆ 999000 │ │ 1 ┆ 499500 │ │ 2 ┆ 999000 │ └──────────┴──────────┘
shape: (6, 2) ┌──────────┬──────────┐ │ column_0 ┆ column_1 │ │ --- ┆ --- │ │ i64 ┆ i64 │ ╞══════════╪══════════╡ │ 1 ┆ 499500 │ │ 2 ┆ 999000 │ │ 1 ┆ 499500 │ │ 2 ┆ 999000 │ │ 1 ┆ 499500 │ │ 2 ┆ 999000 │ └──────────┴──────────┘
-
권장되는 표현식 API 사용 방법
map_rows
대신 표현식 API를 사용하면 더 빠르고 메모리 측면에서 더 빠르고 효율적인 코드를 작성할 수 있습니다. 각 행을 스칼라에 매핑하여 단일 열이 있는 데이터프레임을 반환해보도록 하겠습니다. foo 열에 곱하기 2한 후 bar의 값을 더한 값을 반환해보도록 하겠습니다.```python df = pl.DataFrame({"foo": [1, 2, 3], "bar": [-1, 5, 8]}) print(df.map_rows(lambda t: (t[0] * 2 + t[1]))) # 비효율적인 방법 print(df.select(pl.col("foo") * 2 + pl.col("bar"))) # 효율적인 방법 ``` ``` shape: (3, 1) ┌─────┐ │ map │ │ --- │ │ i64 │ ╞═════╡ │ 1 │ │ 9 │ │ 14 │ └─────┘ shape: (3, 1) ┌─────┐ │ foo │ │ --- │ │ i64 │ ╞═════╡ │ 1 │ │ 9 │ │ 14 │ └─────┘ ``` - 사용자 정의 함수는 Python 기반, 표현식은 Rust 기반으로 실행되므로 표현식을 사용하면 최적화된 성능 제공할 수 있습니다.(성능 : Rust>Python) - 병렬 처리와 논리적 최적화는 표현식에서만 가능합니다. - 표현식이 사용자 정의 함수보다 훨씬 빠르고 성능과 메모리 측면에서 효율적이기 때문에 가능한 표현식을 사용을 권장합니다.(사용자 정의 함수는 표현식으로 구현이 불가능한 경우에만 사용하며, 데이터프레임 메모리 구체화 필요) - 복잡한 연산도 가능한 한 표현식 API로 구현 권장합니다.
```python df = pl.DataFrame({"foo": [1, 2, 3], "bar": [-1, 5, 8]}) print(df.map_rows(lambda t: (t[0] * 2 + t[1]))) # 비효율적인 방법 print(df.select(pl.col("foo") * 2 + pl.col("bar"))) # 효율적인 방법 ``` ``` shape: (3, 1) ┌─────┐ │ map │ │ --- │ │ i64 │ ╞═════╡ │ 1 │ │ 9 │ │ 14 │ └─────┘ shape: (3, 1) ┌─────┐ │ foo │ │ --- │ │ i64 │ ╞═════╡ │ 1 │ │ 9 │ │ 14 │ └─────┘ ``` - 사용자 정의 함수는 Python 기반, 표현식은 Rust 기반으로 실행되므로 표현식을 사용하면 최적화된 성능 제공할 수 있습니다.(성능 : Rust>Python) - 병렬 처리와 논리적 최적화는 표현식에서만 가능합니다. - 표현식이 사용자 정의 함수보다 훨씬 빠르고 성능과 메모리 측면에서 효율적이기 때문에 가능한 표현식을 사용을 권장합니다.(사용자 정의 함수는 표현식으로 구현이 불가능한 경우에만 사용하며, 데이터프레임 메모리 구체화 필요) - 복잡한 연산도 가능한 한 표현식 API로 구현 권장합니다.
:::
4.2 pipe
pipe 메서드는 데이터프레임에 사용자 정의 함수를 체계적으로 시퀀스를 적용하는 방법을 제공합니다.
먼저, 문자열을 정수로 데이터 타입 변환해보도록 하겠습니다. 데이터프레임과 컬럼명을 받아 컬럼의 값들을 정수형으로 변환해보도록 하겠습니다.
def cast_str_to_int(data, col_name):
return data.with_columns(pl.col(col_name).cast(pl.Int64))
df = pl.DataFrame({
"a": [1, 2, 3, 4],
"b": ["10", "20", "30", "40"]
})
result = df.pipe(cast_str_to_int, col_name="b")
print(result)
def cast_str_to_int(data, col_name):
return data.with_columns(pl.col(col_name).cast(pl.Int64))
df = pl.DataFrame({
"a": [1, 2, 3, 4],
"b": ["10", "20", "30", "40"]
})
result = df.pipe(cast_str_to_int, col_name="b")
print(result)
- function: 데이터프레임을 첫 번째 매개변수로 받는 호출 가능한 함수입니다.
- args: 사용자 정의 함수에 전달할 추가 인수입니다.
shape: (4, 2)
┌─────┬─────┐
│ a ┆ b │
│ --- ┆ --- │
│ i64 ┆ i64 │
╞═════╪═════╡
│ 1 ┆ 10 │
│ 2 ┆ 20 │
│ 3 ┆ 30 │
│ 4 ┆ 40 │
└─────┴─────┘
shape: (4, 2)
┌─────┬─────┐
│ a ┆ b │
│ --- ┆ --- │
│ i64 ┆ i64 │
╞═════╪═════╡
│ 1 ┆ 10 │
│ 2 ┆ 20 │
│ 3 ┆ 30 │
│ 4 ┆ 40 │
└─────┴─────┘
열 이름을 기준으로 정렬한 후에 출력해보도록 하겠습니다.
df = pl.DataFrame({
"b": [1, 2],
"a": [3, 4]
})
result = df.pipe(lambda tdf: tdf.select(sorted(tdf.columns)))
print(result)
df = pl.DataFrame({
"b": [1, 2],
"a": [3, 4]
})
result = df.pipe(lambda tdf: tdf.select(sorted(tdf.columns)))
print(result)
shape: (2, 2)
┌─────┬─────┐
│ a ┆ b │
│ --- ┆ --- │
│ i64 ┆ i64 │
╞═════╪═════╡
│ 3 ┆ 1 │
│ 4 ┆ 2 │
└─────┴─────┘
shape: (2, 2)
┌─────┬─────┐
│ a ┆ b │
│ --- ┆ --- │
│ i64 ┆ i64 │
╞═════╪═════╡
│ 3 ┆ 1 │
│ 4 ┆ 2 │
└─────┴─────┘
성능 최적화 팁
- 쿼리 최적화와 병렬화를 최대한 활용하기 위해
LazyFrame
사용 권장 df.lazy()
를 통해 LazyFrame으로 변환 가능
이처럼 pipe 함수는 메서드 체이닝으로 여러 데이터 처리 단계를 연속적으로 연결하여 깔끔한 데이터 파이프라인 구성할 수 있으며, 데이터프레임을 첫 번째 인자로 자동 전달하기 때문에 함수 작성 시 데이터프레임 컨텍스트를 유지할 수 있습니다.
4.3 fold
fold 메서드는 데이터프레임에 수평 축소를 적용하는 메서드로 행 단위 집계에 효과적이며, 슈퍼 캐스팅(비슷한 부모 유형으로 캐스팅)이 가능한 모든 데이터 타입에 적용할 수 있고, NULL 값을 자동 처리해주며 다양한 데이터 타입에 대해 유연한 사용자 정의 연산을 지원합니다.
슈퍼캐스팅 규칙(다른 DataType 간 연산 시 적용되는 규칙)
- Int8 + 문자열 → 문자열
- Float32 + Int64 → Float32
- Float32 + Float64 → Float64
두 개의 시리즈를 받아서 수평 합 연산을 수행한 후 시리즈를 반환해보도록 하겠습니다.
df = pl.DataFrame({
"a": [2, 1, 3],
"b": [1, 2, 3],
"c": [1.0, 2.0, 3.0],
})
result = df.fold(lambda s1, s2: s1 + s2)
print(result)
df = pl.DataFrame({
"a": [2, 1, 3],
"b": [1, 2, 3],
"c": [1.0, 2.0, 3.0],
})
result = df.fold(lambda s1, s2: s1 + s2)
print(result)
shape: (3,)
Series: 'a' [f64]
[
4.0
5.0
9.0
]
shape: (3,)
Series: 'a' [f64]
[
4.0
5.0
9.0
]
zip_with 메서드를 사용하여 두 시리즈 중 최소값을 선택하여 출력해보도록 하겠습니다.
result = df.fold(lambda s1, s2: s1.zip_with(s1 < s2, s2))
print(result)
result = df.fold(lambda s1, s2: s1.zip_with(s1 < s2, s2))
print(result)
- series1.zip_with(조건, series2) : 조건이 True일 때 s1의 값을, False일 때 s2의 값을 반환합니다.
shape: (3,)
Series: 'a' [f64]
[
1.0
1.0
3.0
]
shape: (3,)
Series: 'a' [f64]
[
1.0
1.0
3.0
]
두 시리즈의 문자열 연결해보도록 하겠습니다. 출력 결과를 보시면 마지막 값은 null로 반환된 것을 보실 수 있습니다. 이처럼 null 값은 다른 어떠한 값과 연산을 수행할 수 없음을 의미합니다.
df = pl.DataFrame({
"a": ["foo", "bar", None],
"b": [1, 2, 3],
"c": [1.0, 2.0, 3.0],
})
result = df.fold(lambda s1, s2: s1 + s2)
print(result)
df = pl.DataFrame({
"a": ["foo", "bar", None],
"b": [1, 2, 3],
"c": [1.0, 2.0, 3.0],
})
result = df.fold(lambda s1, s2: s1 + s2)
print(result)
shape: (3,)
Series: 'a' [str]
[
"foo11.0"
"bar22.0"
null
]
shape: (3,)
Series: 'a' [str]
[
"foo11.0"
"bar22.0"
null
]
boolean 값들을 수평 또는 행 방향으로 두 시리즈 간의 OR(|) 연산을 수행해보도록 하겠습니다. 이 연산은 .any() 연산과 비슷하게 동작합니다.
df = pl.DataFrame({
"a": [False, False, True],
"b": [False, True, False],
})
result = df.fold(lambda s1, s2: s1 | s2)
print(result)
df = pl.DataFrame({
"a": [False, False, True],
"b": [False, True, False],
})
result = df.fold(lambda s1, s2: s1 | s2)
print(result)
shape: (3,)
Series: 'a' [bool]
[
false
true
true
]
shape: (3,)
Series: 'a' [bool]
[
false
true
true
]
5. 기타 함수
5.1 estimated_size
estimated_size 메서드는 데이터프레임의 할당된 크기를 추정하여 반환합니다. 예상 크기는 지정된 단위(기본값은 바이트)로 제공됩니다.
df = pl.DataFrame(
{
"x": list(reversed(range(1_000_000))),
"y": [v / 1000 for v in range(1_000_000)],
"z": [str(v) for v in range(1_000_000)],
},
schema=[("x", pl.UInt32), ("y", pl.Float64), ("z", pl.String)],
)
df = pl.DataFrame(
{
"x": list(reversed(range(1_000_000))),
"y": [v / 1000 for v in range(1_000_000)],
"z": [str(v) for v in range(1_000_000)],
},
schema=[("x", pl.UInt32), ("y", pl.Float64), ("z", pl.String)],
)
- reversed : 거꾸로 출력합니다.
# 바이트 단위로 크기 확인
print(df.estimated_size())
# 메가 바이트 단위로 크기 확인
print(df.estimated_size("mb"))
# 바이트 단위로 크기 확인
print(df.estimated_size())
# 메가 바이트 단위로 크기 확인
print(df.estimated_size("mb"))
- unit : 반환된 크기를 주어진 단위로 조정합니다.
- 'b': 바이트
- 'kb': 킬로바이트
- 'mb': 메가바이트
- 'gb': 기가바이트
- 'tb': 테라바이트
17888890
17.0601749420166
17888890
17.0601749420166
메모리 추정과 배열 공유
메모리는 효율성을 위해 여러 배열이 공유할 수 있습니다. 이는 시스템의 메모리 사용을 최적화하는 중요한 메커니즘입니다. 메모리 사용 추정값은 중첩된 배열을 포함한 버퍼 크기(FFI 버퍼 포함)에서 실제로 유효한(사용되는) 부분의 크기를 모두 더한 값입니다. 여러 배열은 버퍼/비트맵(같은 메모리 공간)을 공유할 수 있습니다. 따라서 2개 배열의 크기는 함수에서 계산된 크기의 합계가 아닙니다. 예를 들면, 두 배열이 같은 메모리를 공유하면, 실제 크기는 각각의 크기를 더한 것보다 작을 수 있습니다. 특히 StructArray(구조체 배열)의 계산된 크기는 상한(최대 가능한 크기)를 나타냅니다.
-
배열 공유와 크기 계산 동일한 데이터는 한번만 저장되고 메모리 공유가 되기 때문에 중복 데이터 방지할 수 있을 뿐만 아니라 효율적으로 메모리 사용을 할 수 있습니다. 크기 계산 시, 실제 사용 중인 메모리만 계산됩니다.
arr1 = [1, 2, 3] # 메모리에 [1,2,3] 저장 arr2 = arr1 # 같은 메모리 위치 참조 # 실제 메모리: [1,2,3] (한 번만 저장) # estimated_size는 실제 사용 중인 메모리만 계산
arr1 = [1, 2, 3] # 메모리에 [1,2,3] 저장 arr2 = arr1 # 같은 메모리 위치 참조 # 실제 메모리: [1,2,3] (한 번만 저장) # estimated_size는 실제 사용 중인 메모리만 계산
-
StructArray(구조체 배열)와 메모리 상한 StructArray의 크기는 일반적으로 상한값으로 계산됩니다.
struct_data = { "a": [1, 2, 3], "b": [1, 2, 3] # 'a'와 같은 값 } # 최대 예상 크기: 전체 요소 공간(6개 요소) # 실제 크기: 공유된 메모리로 인해 더 작을 수 있음
struct_data = { "a": [1, 2, 3], "b": [1, 2, 3] # 'a'와 같은 값 } # 최대 예상 크기: 전체 요소 공간(6개 요소) # 실제 크기: 공유된 메모리로 인해 더 작을 수 있음
-
슬라이싱과 메모리 할당 배열이 슬라이스되면 기존 버퍼(실제 메모리에 있는 데이터)는 유지되지만 메모리에 할당된 크기는 일정하게 유지됩니다. 이때, 함수의 크기는 버퍼의 총 용량이 아닌 실제 사용 중인 데이터만 계산하기 때문에 슬라이스 된 데이터는 원본보다 작은 크기로 계산됩니다. 이로 인해 불필요한 메모리 할당 방지하고 최적화를 수행할 수 있습니다.
original = [1, 2, 3, 4, 5] # 메모리: [1,2,3,4,5] sliced = original[0:3] # 메모리는 여전히 [1,2,3,4,5] # 할당된 실제 크기: 전체 배열 크기 유지(5개 요소) # estimated_size가 반환하는 크기: 실제 사용 중인 데이터만 반영(3개 요소)
original = [1, 2, 3, 4, 5] # 메모리: [1,2,3,4,5] sliced = original[0:3] # 메모리는 여전히 [1,2,3,4,5] # 할당된 실제 크기: 전체 배열 크기 유지(5개 요소) # estimated_size가 반환하는 크기: 실제 사용 중인 데이터만 반영(3개 요소)
5.2 transpose
transpose 메서드는 대각선으로 데이터프레임을 전치합니다. 출력 결과를 보시면 열의 위치한 값들이 행 방향으로 행방향에 있던 값은 열로 바뀐것을 보실 수 있습니다.
df = pl.DataFrame({"a": [1, 2, 3], "b": [4, 5, 6]})
print(df.transpose(include_header=True))
df = pl.DataFrame({"a": [1, 2, 3], "b": [4, 5, 6]})
print(df.transpose(include_header=True))
- include_header : 열 이름이 첫 번째 열에 추가됩니다.
shape: (2, 4)
┌────────┬──────────┬──────────┬──────────┐
│ column ┆ column_0 ┆ column_1 ┆ column_2 │
│ --- ┆ --- ┆ --- ┆ --- │
│ str ┆ i64 ┆ i64 ┆ i64 │
╞════════╪══════════╪══════════╪══════════╡
│ a ┆ 1 ┆ 2 ┆ 3 │
│ b ┆ 4 ┆ 5 ┆ 6 │
└────────┴──────────┴──────────┴──────────┘
shape: (2, 4)
┌────────┬──────────┬──────────┬──────────┐
│ column ┆ column_0 ┆ column_1 ┆ column_2 │
│ --- ┆ --- ┆ --- ┆ --- │
│ str ┆ i64 ┆ i64 ┆ i64 │
╞════════╪══════════╪══════════╪══════════╡
│ a ┆ 1 ┆ 2 ┆ 3 │
│ b ┆ 4 ┆ 5 ┆ 6 │
└────────┴──────────┴──────────┴──────────┘
위의 출력 결과를 보시면 자동으로 열이름이 설정된 것을 보실 수 있습니다. 이번에는 자동 생성된 열 이름을 다른 이름으로 바꿔보도록 하겠습니다.
print(df.transpose(include_header=False, column_names=["x", "y", "z"]))
print(df.transpose(include_header=False, column_names=["x", "y", "z"]))
- column_names : 기존 열의 이름을 지정하는 문자열을 리스트로 새로운 컬럼명을 전달할 수 있습니다. 이는 전치된 데이터의 값(헤더 아님) 열의 이름을 지정합니다.
shape: (2, 3)
┌─────┬─────┬─────┐
│ x ┆ y ┆ z │
│ --- ┆ --- ┆ --- │
│ i64 ┆ i64 ┆ i64 │
╞═════╪═════╪═════╡
│ 1 ┆ 2 ┆ 3 │
│ 4 ┆ 5 ┆ 6 │
└─────┴─────┴─────┘
shape: (2, 3)
┌─────┬─────┬─────┐
│ x ┆ y ┆ z │
│ --- ┆ --- ┆ --- │
│ i64 ┆ i64 ┆ i64 │
╞═════╪═════╪═════╡
│ 1 ┆ 2 ┆ 3 │
│ 4 ┆ 5 ┆ 6 │
└─────┴─────┴─────┘
만약 헤더 열 이름을 지정하고 싶으시다면 header_name 매개변수에 헤더 이름을 작성하여 별도의 열로 출력합니다.
print(df.transpose(include_header=True, header_name="foo", column_names=["x", "y", "z"]))
print(df.transpose(include_header=True, header_name="foo", column_names=["x", "y", "z"]))
- header_name :
include_header
설정된 경우 , 삽입될 열의 이름입니다.
shape: (2, 4)
┌─────┬─────┬─────┬─────┐
│ foo ┆ x ┆ y ┆ z │
│ --- ┆ --- ┆ --- ┆ --- │
│ str ┆ i64 ┆ i64 ┆ i64 │
╞═════╪═════╪═════╪═════╡
│ a ┆ 1 ┆ 2 ┆ 3 │
│ b ┆ 4 ┆ 5 ┆ 6 │
└─────┴─────┴─────┴─────┘
shape: (2, 4)
┌─────┬─────┬─────┬─────┐
│ foo ┆ x ┆ y ┆ z │
│ --- ┆ --- ┆ --- ┆ --- │
│ str ┆ i64 ┆ i64 ┆ i64 │
╞═════╪═════╪═════╪═════╡
│ a ┆ 1 ┆ 2 ┆ 3 │
│ b ┆ 4 ┆ 5 ┆ 6 │
└─────┴─────┴─────┴─────┘
자동 생성된 열을 함수를 사용해 규칙적인 열 이름으로 바꿔보도록 하겠습니다.
def name_generator():
base_name = "my_column_"
count = 0
while True:
yield f"{base_name}{count}"
count += 1
print(df.transpose(include_header=False, column_names=name_generator()))
def name_generator():
base_name = "my_column_"
count = 0
while True:
yield f"{base_name}{count}"
count += 1
print(df.transpose(include_header=False, column_names=name_generator()))
shape: (2, 3)
┌─────────────┬─────────────┬─────────────┐
│ my_column_0 ┆ my_column_1 ┆ my_column_2 │
│ --- ┆ --- ┆ --- │
│ i64 ┆ i64 ┆ i64 │
╞═════════════╪═════════════╪═════════════╡
│ 1 ┆ 2 ┆ 3 │
│ 4 ┆ 5 ┆ 6 │
└─────────────┴─────────────┴─────────────┘
shape: (2, 3)
┌─────────────┬─────────────┬─────────────┐
│ my_column_0 ┆ my_column_1 ┆ my_column_2 │
│ --- ┆ --- ┆ --- │
│ i64 ┆ i64 ┆ i64 │
╞═════════════╪═════════════╪═════════════╡
│ 1 ┆ 2 ┆ 3 │
│ 4 ┆ 5 ┆ 6 │
└─────────────┴─────────────┴─────────────┘
기존 열(id)을 반환될 데이터프레임 열 이름으로 사용해보도록 하겠습니다.
df = pl.DataFrame(dict(id=["i", "j", "k"], a=[1, 2, 3], b=[4, 5, 6]))
print(df.transpose(column_names="id"))
df = pl.DataFrame(dict(id=["i", "j", "k"], a=[1, 2, 3], b=[4, 5, 6]))
print(df.transpose(column_names="id"))
shape: (2, 3)
┌─────┬─────┬─────┐
│ i ┆ j ┆ k │
│ --- ┆ --- ┆ --- │
│ i64 ┆ i64 ┆ i64 │
╞═════╪═════╪═════╡
│ 1 ┆ 2 ┆ 3 │
│ 4 ┆ 5 ┆ 6 │
└─────┴─────┴─────┘
shape: (2, 3)
┌─────┬─────┬─────┐
│ i ┆ j ┆ k │
│ --- ┆ --- ┆ --- │
│ i64 ┆ i64 ┆ i64 │
╞═════╪═════╪═════╡
│ 1 ┆ 2 ┆ 3 │
│ 4 ┆ 5 ┆ 6 │
└─────┴─────┴─────┘
원래 열 이름들을 새로운 컬럼으로 생성하고 new_id 열에 저장해보도록 하겠습니다.
print(df.transpose(include_header=True, header_name="new_id", column_names="id"))
print(df.transpose(include_header=True, header_name="new_id", column_names="id"))
shape: (2, 4)
┌────────┬─────┬─────┬─────┐
│ new_id ┆ i ┆ j ┆ k │
│ --- ┆ --- ┆ --- ┆ --- │
│ str ┆ i64 ┆ i64 ┆ i64 │
╞════════╪═════╪═════╪═════╡
│ a ┆ 1 ┆ 2 ┆ 3 │
│ b ┆ 4 ┆ 5 ┆ 6 │
└────────┴─────┴─────┴─────┘
shape: (2, 4)
┌────────┬─────┬─────┬─────┐
│ new_id ┆ i ┆ j ┆ k │
│ --- ┆ --- ┆ --- ┆ --- │
│ str ┆ i64 ┆ i64 ┆ i64 │
╞════════╪═════╪═════╪═════╡
│ a ┆ 1 ┆ 2 ┆ 3 │
│ b ┆ 4 ┆ 5 ┆ 6 │
└────────┴─────┴─────┴─────┘
6. 시계열 데이터 처리
날짜와 시간을 처리하는 방법에 대해 알아보도록 하겠습니다.
6.1 시계열 데이터 생성
from datetime import datetime
# 날짜 범위 생성
dates = pl.date_range(start=datetime(2023,1,1), end=datetime(2023,1,5), interval="1d", eager=True)
df = pl.DataFrame({
'dates':dates
})
print(df)
# 시간 범위 생성
times = pl.datetime_range(start=datetime(2023,1,1), end=datetime(2023,1,5,3), interval="1h")
df = pl.DataFrame({
'times':times
})
print(df)
# 특정 timestamp 생성
timestamp = pl.datetime(2020, 3, 14, 15)
print(timestamp)
from datetime import datetime
# 날짜 범위 생성
dates = pl.date_range(start=datetime(2023,1,1), end=datetime(2023,1,5), interval="1d", eager=True)
df = pl.DataFrame({
'dates':dates
})
print(df)
# 시간 범위 생성
times = pl.datetime_range(start=datetime(2023,1,1), end=datetime(2023,1,5,3), interval="1h")
df = pl.DataFrame({
'times':times
})
print(df)
# 특정 timestamp 생성
timestamp = pl.datetime(2020, 3, 14, 15)
print(timestamp)
shape: (5, 1)
┌────────────┐
│ dates │
│ --- │
│ date │
╞════════════╡
│ 2023-01-01 │
│ 2023-01-02 │
│ 2023-01-03 │
│ 2023-01-04 │
│ 2023-01-05 │
└────────────┘
shape: (100, 1)
┌─────────────────────┐
│ times │
│ --- │
│ datetime[μs] │
╞═════════════════════╡
│ 2023-01-01 00:00:00 │
│ 2023-01-01 01:00:00 │
│ 2023-01-01 02:00:00 │
│ 2023-01-01 03:00:00 │
│ 2023-01-01 04:00:00 │
│ … │
│ 2023-01-04 23:00:00 │
│ 2023-01-05 00:00:00 │
│ 2023-01-05 01:00:00 │
│ 2023-01-05 02:00:00 │
│ 2023-01-05 03:00:00 │
└─────────────────────┘
2020-03-14 15:00:00.alias("datetime")
shape: (5, 1)
┌────────────┐
│ dates │
│ --- │
│ date │
╞════════════╡
│ 2023-01-01 │
│ 2023-01-02 │
│ 2023-01-03 │
│ 2023-01-04 │
│ 2023-01-05 │
└────────────┘
shape: (100, 1)
┌─────────────────────┐
│ times │
│ --- │
│ datetime[μs] │
╞═════════════════════╡
│ 2023-01-01 00:00:00 │
│ 2023-01-01 01:00:00 │
│ 2023-01-01 02:00:00 │
│ 2023-01-01 03:00:00 │
│ 2023-01-01 04:00:00 │
│ … │
│ 2023-01-04 23:00:00 │
│ 2023-01-05 00:00:00 │
│ 2023-01-05 01:00:00 │
│ 2023-01-05 02:00:00 │
│ 2023-01-05 03:00:00 │
└─────────────────────┘
2020-03-14 15:00:00.alias("datetime")
6.2 문자열을 datetime으로 변환
데이터 타입 변환 시, strptime
또는 strftime
사용합니다.
df = pl.DataFrame({
'date': [
'2022-01-01 09:21:00',
'2022-01-02 09:22:00',
'2022-01-03 09:23:00'
]
})
print(df)
df = df.with_columns(
pl.col('date').str.strptime(pl.Datetime, format="%Y-%m-%d %H:%M:%S")
)
print(df)
df = pl.DataFrame({
'date': [
'2022-01-01 09:21:00',
'2022-01-02 09:22:00',
'2022-01-03 09:23:00'
]
})
print(df)
df = df.with_columns(
pl.col('date').str.strptime(pl.Datetime, format="%Y-%m-%d %H:%M:%S")
)
print(df)
shape: (3, 1)
┌─────────────────────┐
│ date │
│ --- │
│ str │
╞═════════════════════╡
│ 2022-01-01 09:21:00 │
│ 2022-01-02 09:22:00 │
│ 2022-01-03 09:23:00 │
└─────────────────────┘
shape: (3, 1)
┌─────────────────────┐
│ date │
│ --- │
│ datetime[μs] │
╞═════════════════════╡
│ 2022-01-01 09:21:00 │
│ 2022-01-02 09:22:00 │
│ 2022-01-03 09:23:00 │
└─────────────────────┘
shape: (3, 1)
┌─────────────────────┐
│ date │
│ --- │
│ str │
╞═════════════════════╡
│ 2022-01-01 09:21:00 │
│ 2022-01-02 09:22:00 │
│ 2022-01-03 09:23:00 │
└─────────────────────┘
shape: (3, 1)
┌─────────────────────┐
│ date │
│ --- │
│ datetime[μs] │
╞═════════════════════╡
│ 2022-01-01 09:21:00 │
│ 2022-01-02 09:22:00 │
│ 2022-01-03 09:23:00 │
└─────────────────────┘
6.3 날짜/시간 데이터 추출
dt
메서드를 사용하여 날짜/시간 관련 데이터를 추출할 수 있습니다.
df = df.with_columns([
# 날짜 관련
pl.col('date').dt.date().alias('date_only'), # YYYY-MM-DD
pl.col('date').dt.year().alias('year'), # 연도
pl.col('date').dt.month().alias('month'), # 월
pl.col('date').dt.day().alias('day'), # 일
# 시간 관련
pl.col('date').dt.hour().alias('hour'), # 시
pl.col('date').dt.minute().alias('minute'), # 분
pl.col('date').dt.second().alias('second'), # 초
])
df = df.with_columns([
# 날짜 관련
pl.col('date').dt.date().alias('date_only'), # YYYY-MM-DD
pl.col('date').dt.year().alias('year'), # 연도
pl.col('date').dt.month().alias('month'), # 월
pl.col('date').dt.day().alias('day'), # 일
# 시간 관련
pl.col('date').dt.hour().alias('hour'), # 시
pl.col('date').dt.minute().alias('minute'), # 분
pl.col('date').dt.second().alias('second'), # 초
])
shape: (3, 8)
┌─────────────────────┬────────────┬──────┬───────┬─────┬──────┬────────┬────────┐
│ date ┆ date_only ┆ year ┆ month ┆ day ┆ hour ┆ minute ┆ second │
│ --- ┆ --- ┆ --- ┆ --- ┆ --- ┆ --- ┆ --- ┆ --- │
│ datetime[μs] ┆ date ┆ i32 ┆ i8 ┆ i8 ┆ i8 ┆ i8 ┆ i8 │
╞═════════════════════╪════════════╪══════╪═══════╪═════╪══════╪════════╪════════╡
│ 2022-01-01 09:21:00 ┆ 2022-01-01 ┆ 2022 ┆ 1 ┆ 1 ┆ 9 ┆ 21 ┆ 0 │
│ 2022-01-02 09:22:00 ┆ 2022-01-02 ┆ 2022 ┆ 1 ┆ 2 ┆ 9 ┆ 22 ┆ 0 │
│ 2022-01-03 09:23:00 ┆ 2022-01-03 ┆ 2022 ┆ 1 ┆ 3 ┆ 9 ┆ 23 ┆ 0 │
└─────────────────────┴────────────┴──────┴───────┴─────┴──────┴────────┴────────┘
shape: (3, 8)
┌─────────────────────┬────────────┬──────┬───────┬─────┬──────┬────────┬────────┐
│ date ┆ date_only ┆ year ┆ month ┆ day ┆ hour ┆ minute ┆ second │
│ --- ┆ --- ┆ --- ┆ --- ┆ --- ┆ --- ┆ --- ┆ --- │
│ datetime[μs] ┆ date ┆ i32 ┆ i8 ┆ i8 ┆ i8 ┆ i8 ┆ i8 │
╞═════════════════════╪════════════╪══════╪═══════╪═════╪══════╪════════╪════════╡
│ 2022-01-01 09:21:00 ┆ 2022-01-01 ┆ 2022 ┆ 1 ┆ 1 ┆ 9 ┆ 21 ┆ 0 │
│ 2022-01-02 09:22:00 ┆ 2022-01-02 ┆ 2022 ┆ 1 ┆ 2 ┆ 9 ┆ 22 ┆ 0 │
│ 2022-01-03 09:23:00 ┆ 2022-01-03 ┆ 2022 ┆ 1 ┆ 3 ┆ 9 ┆ 23 ┆ 0 │
└─────────────────────┴────────────┴──────┴───────┴─────┴──────┴────────┴────────┘
6.4 날짜/시간 포맷팅
데이터 포맷 변환 시, strptime
또는 strftime
사용합니다.
df = df.with_columns([
# 날짜를 특정 형식으로 포맷팅
pl.col('date').dt.strftime("%Y-%m-%d").alias('formatted_date'),
# 시간을 특정 형식으로 포맷팅
pl.col('date').dt.strftime("%H:%M:%S").alias('formatted_time')
])
print(df)
df = df.with_columns([
# 날짜를 특정 형식으로 포맷팅
pl.col('date').dt.strftime("%Y-%m-%d").alias('formatted_date'),
# 시간을 특정 형식으로 포맷팅
pl.col('date').dt.strftime("%H:%M:%S").alias('formatted_time')
])
print(df)
shape: (3, 10)
┌────────────────┬────────────┬──────┬───────┬───┬────────┬────────┬───────────────┬───────────────┐
│ date ┆ date_only ┆ year ┆ month ┆ … ┆ minute ┆ second ┆ formatted_dat ┆ formatted_tim │
│ --- ┆ --- ┆ --- ┆ --- ┆ ┆ --- ┆ --- ┆ e ┆ e │
│ datetime[μs] ┆ date ┆ i32 ┆ i8 ┆ ┆ i8 ┆ i8 ┆ --- ┆ --- │
│ ┆ ┆ ┆ ┆ ┆ ┆ ┆ str ┆ str │
╞════════════════╪════════════╪══════╪═══════╪═══╪════════╪════════╪═══════════════╪═══════════════╡
│ 2022-01-01 ┆ 2022-01-01 ┆ 2022 ┆ 1 ┆ … ┆ 21 ┆ 0 ┆ 2022-01-01 ┆ 09:21:00 │
│ 09:21:00 ┆ ┆ ┆ ┆ ┆ ┆ ┆ ┆ │
│ 2022-01-02 ┆ 2022-01-02 ┆ 2022 ┆ 1 ┆ … ┆ 22 ┆ 0 ┆ 2022-01-02 ┆ 09:22:00 │
│ 09:22:00 ┆ ┆ ┆ ┆ ┆ ┆ ┆ ┆ │
│ 2022-01-03 ┆ 2022-01-03 ┆ 2022 ┆ 1 ┆ … ┆ 23 ┆ 0 ┆ 2022-01-03 ┆ 09:23:00 │
│ 09:23:00 ┆ ┆ ┆ ┆ ┆ ┆ ┆ ┆ │
└────────────────┴────────────┴──────┴───────┴───┴────────┴────────┴───────────────┴───────────────┘
shape: (3, 10)
┌────────────────┬────────────┬──────┬───────┬───┬────────┬────────┬───────────────┬───────────────┐
│ date ┆ date_only ┆ year ┆ month ┆ … ┆ minute ┆ second ┆ formatted_dat ┆ formatted_tim │
│ --- ┆ --- ┆ --- ┆ --- ┆ ┆ --- ┆ --- ┆ e ┆ e │
│ datetime[μs] ┆ date ┆ i32 ┆ i8 ┆ ┆ i8 ┆ i8 ┆ --- ┆ --- │
│ ┆ ┆ ┆ ┆ ┆ ┆ ┆ str ┆ str │
╞════════════════╪════════════╪══════╪═══════╪═══╪════════╪════════╪═══════════════╪═══════════════╡
│ 2022-01-01 ┆ 2022-01-01 ┆ 2022 ┆ 1 ┆ … ┆ 21 ┆ 0 ┆ 2022-01-01 ┆ 09:21:00 │
│ 09:21:00 ┆ ┆ ┆ ┆ ┆ ┆ ┆ ┆ │
│ 2022-01-02 ┆ 2022-01-02 ┆ 2022 ┆ 1 ┆ … ┆ 22 ┆ 0 ┆ 2022-01-02 ┆ 09:22:00 │
│ 09:22:00 ┆ ┆ ┆ ┆ ┆ ┆ ┆ ┆ │
│ 2022-01-03 ┆ 2022-01-03 ┆ 2022 ┆ 1 ┆ … ┆ 23 ┆ 0 ┆ 2022-01-03 ┆ 09:23:00 │
│ 09:23:00 ┆ ┆ ┆ ┆ ┆ ┆ ┆ ┆ │
└────────────────┴────────────┴──────┴───────┴───┴────────┴────────┴───────────────┴───────────────┘